As a change from my usual posts, I thought I’d note five small things I’ve learned recently, mostly to do with Stata, with the hope that they might help others, or at least jog my memory when I unlearn them again soon.
1.Stata’s random number generator has a limit on the seed that you can set of 2,147,483,647.
Why did I learn this? We were doing a live random assignment for an impact evaluation I am starting in Colombia. We had programmed up the code, and tested it several times, with it working fine. In our test code, we had set the seed for random number generation as the date “04112018”. Then when my collaborator went to run this live, it was decided to also add the time of the drawing at the end, so that the seed was set as “041120180304”. This generated an error, and prevented the code from running. Luckily we could quickly fix it, and the live draw proceeded ok. But lesson learned, 2^31-1 is a large number, but sometimes binds.
2. Avoid Stata’s rank command if you want replicable random assignment.
This is a lesson that I have to keep on re-learning. The situation here is part of the same random assignment. We wanted to rank firms within strata according to an export practices index (EPindex), and then randomize within each strata among quadruplets of firms with similar practice levels. The problem that arises comes when observations are tied with the same value of the variable. If you use:
egen rankEPindex = rank(EPindex), by(strata) unique
Then the unique option splits ties, but it does so “arbitrarily”, with this being different each time you run the code, regardless of how you have set the seed. This is a problem for replicability.
Instead, you are better to use the following two lines:
sort strata (EPindex), stable
by strata: gen rankEPindex=_n
The stable option here to sort maintains the order that you have the data already, so will yield the same results each time you run the code.
[update: Arthur Alik Lagrange also helpfully informs me that Stata has a separate command set sortseed that sets the seed used for breaking ties when data are sorted]
I currently have a survey in the field in Nigeria, where we are collecting the GPS coordinates of businesses as part of the survey. I wanted to take a quick look to see where surveying had taken place, and to visually see whether my different treatment groups look like they are spatially randomly disbursed. I found this guide which helped quickly put the data on a map:
Step 1: download a shape file for your region. A quick google found this map library of Africa, and I could then get a shape file for Lagos in .shp form.
Step 2: convert the shape file into Stata format using shp2dta
ssc install shp2dta
shp2dta using " NIR-24.shp", data("lagos_data") coor("lagos_coordinates")
Step 3: Plot your data using the tmap command
tmap dot, by(treatment) ycoord(gps_Latitude) xcoord(gps_Longitude) map("lagos_coordinates.dta")
This gives a basic map, like the one below. You can of course then make it prettier, but from the point of view of quickly seeing where interviews have taken place, I found this very useful.
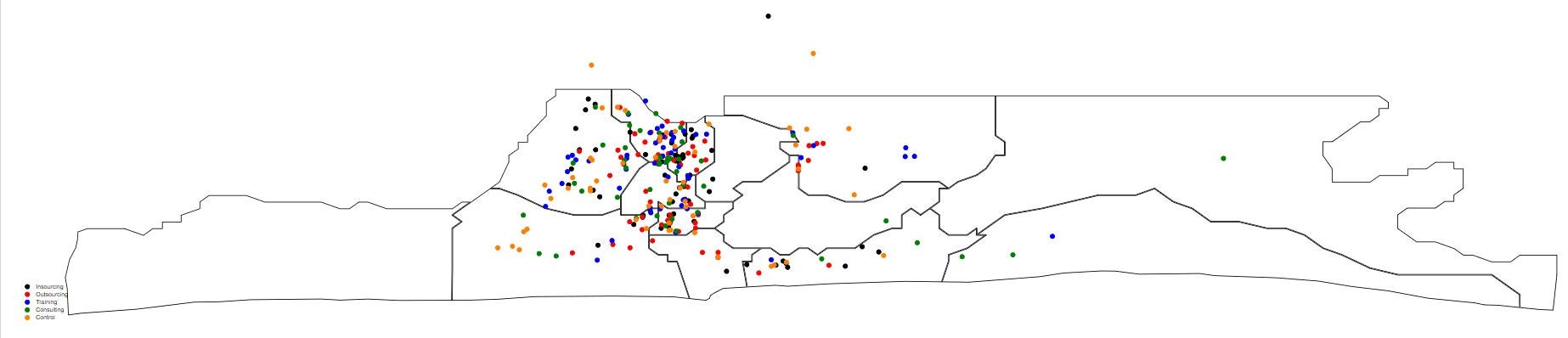
(note: I have randomly perturbed points for this illustration to maintain anonymity of respondents).
4. Getting rid of commas, dollar signs, etc. from numeric variables that appears as strings
I’ve been working with follow-up survey data from a project in the Western Balkans, where some of the data that was collected was entered with commas and dollar signs. The data are then a string variable, with values like $1,000,000. I don’t know why it took me this long to learn the subinstr command in Stata, that can get rid of commas and dollar signs.
E.g. if you want to get rid of the commas:
replace amountinvested = subinstr(amountinvested, ”,”,””,.)
5. Apparently not everyone thinks winsorizing and truncating are the same thing
I’ve never used the term winsorize in my papers, but instead have distinguished between truncation (by which I meant where values above the 99 th percentile are truncated or shortened by replacing them with the value of the 99 th percentile) and trimming (by which I meant where values above the 99 th percentile are trimmed or cut from the dataset and set to missing). I had thought then that people were using winsorize and truncation to mean the same thing. Only recently have I discovered that most people use truncation and trimming as the same thing, both to mean excluding data above or below some percentile, and only winsorizing to mean replacing outliers above or below some percentile with that percentile. So whenever you read truncate in my old papers, you should consider it to mean winsorized.
What small thing have you learned recently, that you wish you had known earlier or think others might appreciate knowing? Please share in the comments.
Join the Conversation