Scientific advances are the result of a long, cumulative process of building knowledge and methodologies -- or, as the cliché goes, “standing on the shoulders of giants”. One often overlooked, but crucial part of this climb is a long tradition of standardization of everything from mathematical notation and scientific terminology, to format for academic articles and references.
Over the last years, the scope and complexity of data in development research have grown exponentially, and similar standardizations for data work are needed to enable our generation of researchers to stand on the shoulder of giants. Our team at DIME Analytics has the ambition to gather, document, contribute to and disseminate best practices for data work: ietoolkit is one of the results of this effort.
ietoolkit is a Stata package containing several commands to routinize tasks in impact evaluation. It can be installed through SSC and the codes are available on GitHub. We add commands to this package regularly, but currently it includes polished code and documentation for:
As you can see, there is no crazy new econometrics or methodology in ietoolkit, just the tasks research assistants do every day. With modular programming in mind, we standardized and wrapped them, because
One quick example
Just think of said RA: say they are asked by a Principal Investigator (PI) to generate a balance table. They probably know how to make t-tests or run regressions, save test statistics and create a table. However, if the PI wants to add a variable, a control, or additional test to the table, it is not certain that it is easily added to the code written for the first table, and might require a different approach. The command iebaltab saves a great deal of time in the initial implementation, and even more so when making changes or adding features as a long range of options are available. iebaltab does not do anything a good RA can’t already do, but it allows the RAs to save time and use their skills on more important things.
The command is used like this:
iebaltab can export both to Excel and LaTeX. And in addition to the functions iebaltab is explicitly asked to do, it tests for common pitfalls that could make the analysis invalid, and throws warnings about these when applicable.
Contributions
Like any code library in any programming language, a package of commands never reaches its full potential without feedback from users. If you are not familiar with GitHub you can email us using the current contact on the ietoolkit page.
This code is provided as a public good under the MIT license, so do whatever you like with it. And as any code is always work in progress, if you find a bug, have an improvement to suggest, or an idea for building something cool, let us know by either posting an issue on the GitHub repository or making a pull request to the repo with your own code.
Over the last years, the scope and complexity of data in development research have grown exponentially, and similar standardizations for data work are needed to enable our generation of researchers to stand on the shoulder of giants. Our team at DIME Analytics has the ambition to gather, document, contribute to and disseminate best practices for data work: ietoolkit is one of the results of this effort.
ietoolkit is a Stata package containing several commands to routinize tasks in impact evaluation. It can be installed through SSC and the codes are available on GitHub. We add commands to this package regularly, but currently it includes polished code and documentation for:
- iebaltab, to create multiple treatment arm balance tables
- iegraph, to visualize estimation results from common regression models (like diff-in-diff)
- iematch, to match observations in one group to “the most similar” observations in another
- ieduplicates and iecompdup, to identify and correct for duplicates
- ieboilstart, to standardize the boilerplate code at the top of all do-files
- iefolder, to set up project folders and create master do-files that link to all sub-folders
- iegitaddmd, to add README.md files to folders intended to be shared on GitHub
- iedropone, to drop an exact number of observations even as the data set changes
- ieboilsave, to perform checks before saving a dataset
As you can see, there is no crazy new econometrics or methodology in ietoolkit, just the tasks research assistants do every day. With modular programming in mind, we standardized and wrapped them, because
- There’s no need to reinvent the wheel every time you do a routine task;
- By using and building up commands, you avoid repeating mistakes others have made before;
- You can write just one easily readable line of code instead of many lines that later may be difficult to understand;
- It is easier for someone else to work with and build on standardized code. The “standing on shoulders of giants” part of data work.
One quick example
Just think of said RA: say they are asked by a Principal Investigator (PI) to generate a balance table. They probably know how to make t-tests or run regressions, save test statistics and create a table. However, if the PI wants to add a variable, a control, or additional test to the table, it is not certain that it is easily added to the code written for the first table, and might require a different approach. The command iebaltab saves a great deal of time in the initial implementation, and even more so when making changes or adding features as a long range of options are available. iebaltab does not do anything a good RA can’t already do, but it allows the RAs to save time and use their skills on more important things.
The command is used like this:
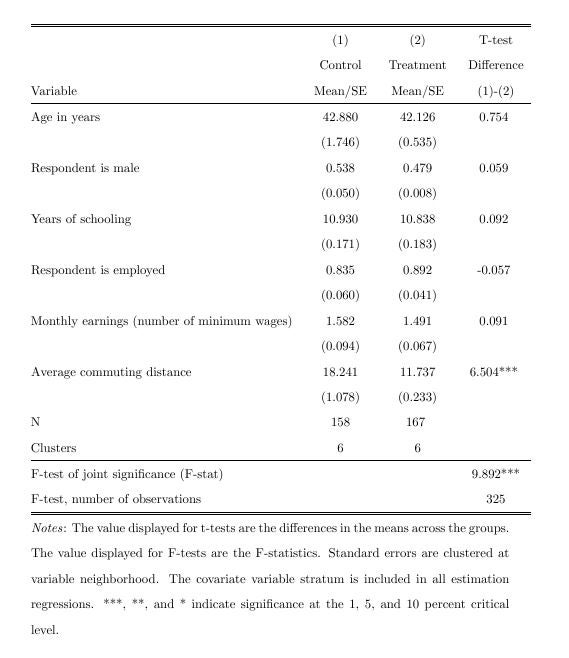
iebaltab can export both to Excel and LaTeX. And in addition to the functions iebaltab is explicitly asked to do, it tests for common pitfalls that could make the analysis invalid, and throws warnings about these when applicable.
Contributions
Like any code library in any programming language, a package of commands never reaches its full potential without feedback from users. If you are not familiar with GitHub you can email us using the current contact on the ietoolkit page.
This code is provided as a public good under the MIT license, so do whatever you like with it. And as any code is always work in progress, if you find a bug, have an improvement to suggest, or an idea for building something cool, let us know by either posting an issue on the GitHub repository or making a pull request to the repo with your own code.
Join the Conversation