In recent years, the World Bank has been exploring the use of artificial intelligence for disaster risk management, such as how to capture housing data with machine learning, and how to do it in a way that is responsible and does no harm. Building upon this work, new research is showing that by integrating machine learning with strategic aerial imagery and local insights, nations stand to acquire pivotal housing data with unmatched speed and cost-efficiency.
Generating critical housing information
In 2017, Hurricane Maria made landfall in Dominica, bringing strong winds and torrential rain, and leaving a widespread trail of destruction in its wake. The Category 5 storm destroyed over 28,000 homes (nearly 90% of the building stock), with damages and losses estimated at $380 million in the housing sector alone. The devastation brought about by Hurricane Maria has spurred ambitious climate resilience initiatives by government agencies and international organizations aiming to reduce the impact of extreme weather events and other natural hazards. For example, the Resilient Housing Scheme by the Government of the Commonwealth of Dominica strives to make 90% of housing stock resilient by 2030. For these programs to be successful, accurate and up-to-date maps of buildings and their characteristics are needed to identify and retrofit damaged structures, relocate vulnerable and at-risk citizens, and construct new resilient homes. Traditional house-to-house surveys, although comprehensive, are time-consuming and expensive—often out of reach for developing countries. Without a way to rapidly generate critical baseline data, decision-makers risk allocating valuable resources to the wrong priorities and delaying urgent humanitarian response.
Combining AI, Earth observation, and local expertise
Fortunately, thanks to the rapid advancement of artificial intelligence (AI) and the increasing availability of Earth observation (EO) data, a solution is within reach. AI allows us to quickly and efficiently extract meaningful information, such as building footprints and roof material, from remote sensing data like satellite and drone images. This makes it a powerful tool for tackling disaster prevention and response challenges. However, to take advantage of these technologies requires strengthening local capacity. To address this challenge, we need to empower governments and stakeholders by building local skills and capacity while raising awareness about the capabilities, requirements, and limitations of these systems.
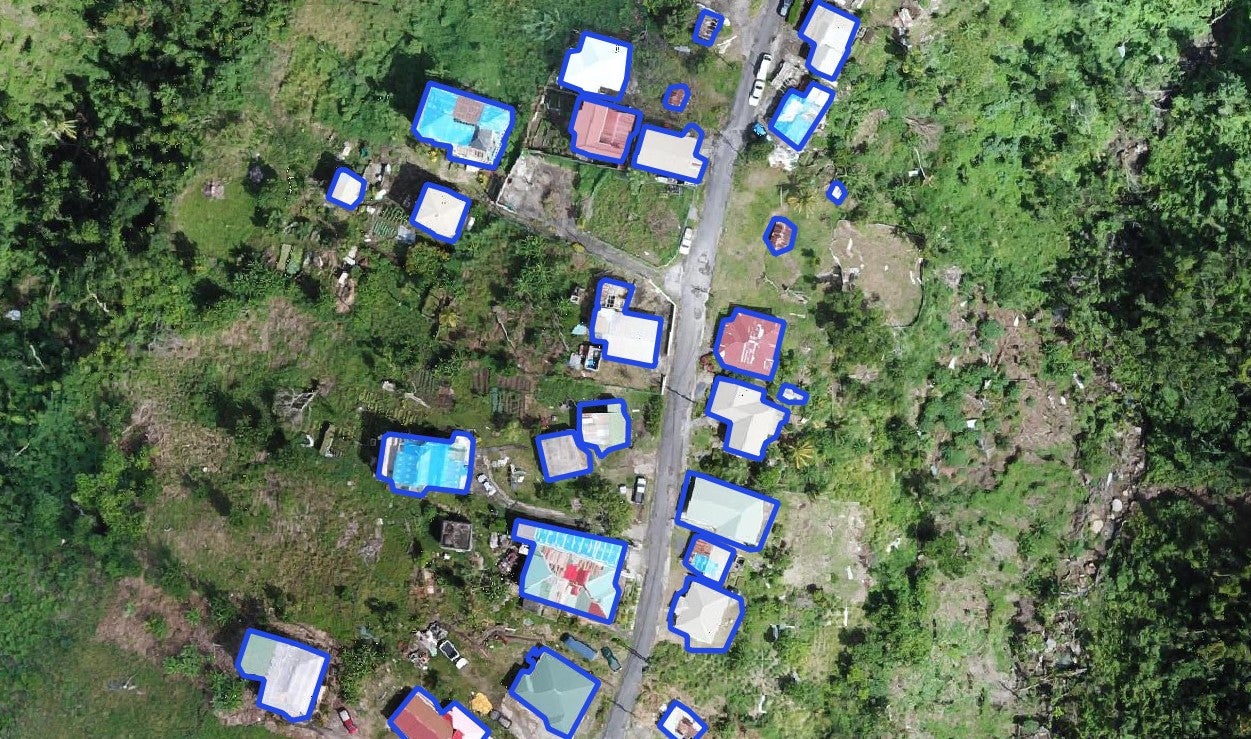
To do this, the World Bank with support from the Digital Earth Program under the Global Facility for Disaster Reduction and Recovery (GFDRR) is training local government staff and other key stakeholders from Dominica and Saint Lucia to manage large-scale geospatial datasets and operate drones to enable inexpensive and frequent collection of very high-resolution aerial images. From the drone images, AI tools can be used to extract building characteristics such as building size, roof material, and damage levels. Building on the work done by the Global Program for Resilient Housing (GPRH), we conducted a pilot study in Dominica where we used AI to automatically extract building footprints and classify rooftops from very high-resolution orthophotos, achieving correct prediction for approximately 90% of the houses. The model outputs consist of building footprints labeled with their corresponding roof type (e.g. flat, gable, hip) and roof material (e.g. concrete, metal, blue tarpaulin, incomplete), attributes that are key to determining a building’s resistance to wind or whether it has been damaged. By investing in the regular collection of aerial images, governments can also use pre- and post-disaster aerial images to rapidly identify damaged buildings after an extreme hazard event.
Improving decision-making with Human-AI collaboration
Later this year, the World Bank aims to further this endeavor, collaborating with local experts to manually interpret, validate, and refine the AI model outputs. Integrating human-in-the-loop workflows allows human validators to address model limitations to obtain the most accurate results, increasing confidence and trust in the generated maps. Moreover, overlaying the AI-generated information with hazard maps (e.g. flood inundation maps, storm surge risk maps) can help decision-makers quickly and efficiently identify high-risk structures. Using a combination of AI and human expertise, local government units can inexpensively generate critical baseline information at a household level in a short time.
Combining technologies such as AI and EO with local knowledge and expertise has immense potential to enable more timely, data-driven decision-making in support of resilient housing and infrastructure. By using innovative technologies and building local capacity, governments can be better equipped to not only respond more immediately to post-disaster needs but also anticipate and manage risks before a calamity strikes. It’s time we harness the potential of AI, EO, and local expertise to reduce the adverse effects of climate change and prevent the loss of human lives.
Join the Conversation