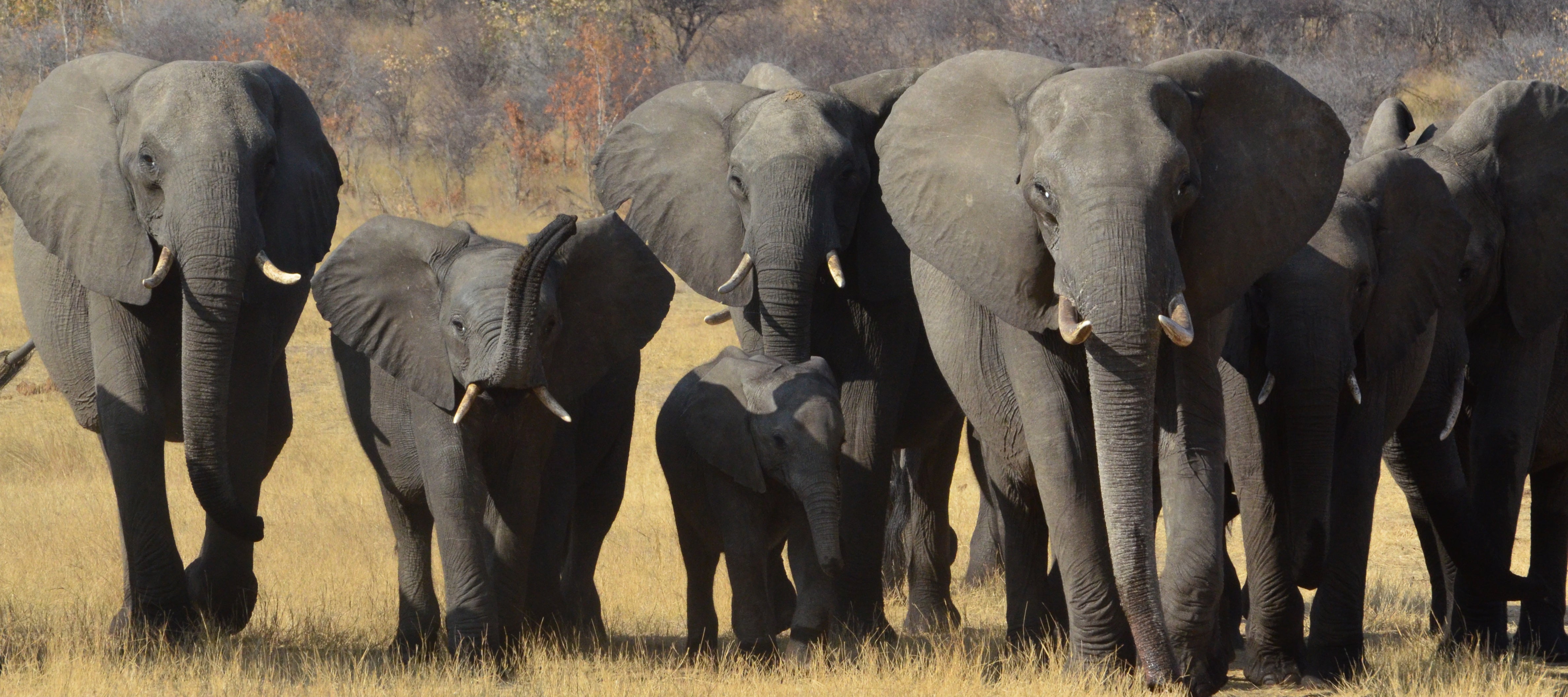
This post originally appeared at the Global Delivery Initiative's blog. GDI is a partnership of over 50 development organizations focused on collecting and sharing operational knowledge, insights, and lessons to better understand what works — and what doesn't — in implementation, including the adaptive implementation themes highlighted in this essay.
Is “adaptive development” a fad or a cure? Old wine in new bottles or a genuine shift in development orthodoxy that can succeed in building state capability for policy implementation?
The case for a new approach has been well made: A robust body of evidence demonstrates that the old orthodoxy — characterized by interventions that transplant ready-made, best-practice solutions from one country to another — not only fails to build such capability but can exact a crushing burden on existing institutions, sometimes undermining the weak extant capability and drive that had painstakingly been built to that point.
Emerging as a potential new orthodoxy, “adaptive development” or #adaptdev loosely describes a collection of approaches that distinguish themselves from the “cargo-transfer” or “solution-driven” tendencies of current practice. Broadly speaking, #adaptdev proponents insist on the primacy of context, the need for humility about exactly what works when it comes to complex development challenges, and the necessity of experimentation, iteration and learning (see here, here and here for discussions of the divergences and similarities between the likes of PDIA, TWP, DDD etc).
But the recurring question #adaptdev evangelists keep hearing from the colleagues they are trying to convert is simply, “Does it actually work?” In other words, has an adaptive approach been shown to fix problems that matter?
It turns out that developing and operationalizing alternatives to the solution-driven model of development that are sound, supportable, implementable and (ultimately, demonstrably) ‘better’ is not a simple task. According to a recent paper (Dasandi et al 2019), there are significant weaknesses in how the case for impact has been made thus far; “much evidence is anecdotal, does not meet high standards of robustness, is not comparative, and draws on self-selected successes reported by programme insiders.” Duncan Green pushes back on this judgement a little, suggesting that “anecdote” has its place and that “action research” is probably the best way to provide convincing evidence that pays due attention to context, chance, and the interaction between political economy and human agency and leadership.”
Aside from the fact that the #adaptdev often being undertaken is itself not immune to isomorphism, and/or can be heavily conditioned by the context within which it is implemented, the challenge for those wishing to argue for #adaptdev’s effectiveness is that its success or failure cannot always be measured with tools designed to measure traditional approaches.
So: how then can we demonstrate whether our adaptive efforts are working?
Thinking about measuring adaptive approaches in the health sector in Nigeria
In a recent World Bank Policy and Research Working Paper, which discusses an adaptive approach applied to health challenges in Nigerian states, we argue that adaptive approaches can and should be tested against the claims they make. Those claims are twofold: (1) that adaptive approaches can fix problems that matter, and (2) that they build problem-solving skills in the process. We’ll focus here on the first claim.
With regard to the critical question of “did we fix the problem?”, one of the issues we grapple with in the paper is the difficulty of deciding what level of “problem fixed" (e.g., national or local; whole of government or departmental) equates to success of the approach. In the Nigeria case, the success of the approach was primarily judged on the attainment of six state-wide aggregate health outcomes, including immunization rates, contraceptive use, skilled birth attendance, and utilization of insecticide-treated nets. The firm selected to facilitate the adaptive approach with the states was given a performance-based contract, and a third of their payment was made conditional on achievement of state-wide increases in these six outcomes (as measured by yearly surveys) in the eight states where they were operating.
The intent to have a clear measure of problem fixed is welcome, but were state-level health outcomes the right measure? The assemblage required to shift state level health outcomes in Nigeria comprises a vast array of intertwined political, economic and social factors. Some of these factors are observable (pre-existing policies, donor programs, funding levels, political priorities), while others are statistically unobservable (political favors, leadership quality, cultural issues, personality clashes). Collectively, these factors shape outcomes from both the supply and demand side. On the ‘supply side’, we find factors relating to where money goes, how reliably and frequently staff are paid, how diligently they work, and so forth. On the ‘demand side’ are factors that include whether people use birth control, immunize their children, adequately feed them, sleep under mosquito nets, and consult maternal health professionals, among many other behaviors. Together, this vast assemblage of factors make up what we came to refer to, during our research, as the “elephant.” All else considered, the elephant is likely to be by far the greatest determinant of aggregate outcomes.
By contrast, the country-wide, World Bank-supported health intervention under which these six outcomes were being targeted, we compared to a bird on the back of the elephant. (A commissioner we interviewed in one of the states likened their efforts under the intervention to “pouring a cup of tea into an ocean” — a reference to the ways that political machinations around civil servant pay were at that time overwhelming any possible program impact.) And to continue our fauna analogy, the adaptive component, funded at just 0.1% of the health intervention budget in which it sat, is a fly on the back of the bird. In the states that received this assistance, the overall movement in the six key aggregate indicators can thus be said to be driven by the combined influence of a fly, a bird and an elephant. (And massive contextual differences across the states mean that we really have different flies on different birds on different elephants!) Whatever movement, positive or otherwise, we see in these indicators cannot and should not be attributed to the fly alone.
The analogy isn’t perfect, but the point is this: yes, adaptive approaches should be judged on their ability to fix problems but those judgments of success must be closely aligned with the actual problems that the approach directly addresses. While the measures of success in the Nigeria case were at the state level, the problems the adaptive approach was actually working on were, due to the targeted nature and relatively small scale of the assistance, necessarily far more discrete and localized. These included issues like “people in X area use the bed nets we distribute for fishing so that they can catch fish to eat and sell”; “Health workers haven’t been paid for the past six months so they charge for services that should be free”; “Religious leaders in this locality are opposed to the use of contraceptives by their members, so women either don’t use them or don’t report the use”; “Health workers are absent because doctors have been kidnapped in the past”; and a host of others. It is on some of these localized, discrete manifestations of the problem, that the “fly” — the adaptive assistance — focused its efforts. We argue that in judging success of the fly’s efforts, evaluation should likewise focus on movements at this level.
Of course, one may still reasonably assume that the fixing of these discrete, local-level issues will progressively combine to shift state-wide health outcome indicators, but as with any theory of change, the assumption that flies can help move elephants requires testing. And demonstrating causation here will be tough. While it is reasonable is to expect to be able to discern effects that are closely coupled with the change (e.g., to see whether a designated solution to the issue of religious leaders’ opposition to contraception did in fact result in greater use of contraceptives in that locality) it does not follow that that same solution — even if unambiguously and wildly successful in resolving that localized problem — can be causally connected to changes (or not) in aggregate outcomes of contraceptive use at a state-wide level. There are just too many links in the chain — or, to put it differently, there is vastly too much “noise” in large complex systems drowning out whatever “signal” may be emanating from a particular location. Even if the vast assemblage of factors ultimately does come together to shift a state-wide indicator, the trajectory of change in such complex interventions is rarely linear and the specific contribution of the ‘adaptive’ component will be almost impossible to empirically isolate.
In short, we recommend having success measures that closely correlate to the problems at the level that the adaptive approach was actually working, rather than having them tied to a vision of success that is many miles down the road of one’s Theory of Change assumptions. And if this seems too much like “starting small” to you, then be reminded that thinking big, starting small and learning fast is one of the mantras that adaptive approaches have borrowed from their agile cousins in the world of lean tech startups. The good news is that solving local, discrete but functional problems matters — in its own right and because failing to do so creates a blockage point, or ‘binding constraint,’ on the larger system’s overall functionality.
We can be confident that the progressive unblocking of small, discrete problems is a valid task. And, if we wish to increase confidence in these methods, we should certainly be measuring our success in doing so with every adaptive approach we implement.
Join the Conversation