In much of the world, a great deal of economic activity is informal. Estimates show that in the typical developing economy, about 70 percent of employment is in the informal sector, though this share of labor only makes up about 30 percent of production. However, in many cases data on most such informal businesses can be hard to obtain because they are absent from official or credible sources. Also, existing methods to obtain such data are costly.
One way to reach these informal businesses would be to survey them, but complete sampling frames—the comprehensive lists required for rigorously conducting a representative survey—usually exclude informal businesses.
Because policy making requires evidence-based, high-quality representative data, countries are making measuring informal businesses’ activity a priority. For example, India and Rwanda conduct establishment censuses that include unregistered, informal businesses. However, even in those instances, data collection remains expensive, and so the measurement of informal business activity is infrequent or completely absent in most countries.
In our recent paper, published in the Journal of Survey Statistics and Methodology, we explore a sampling methodology that targets a clustered population. Our paper applies a sampling methodology called Adaptive Cluster Sampling (ACS) to surveying informal businesses. ACS was originally proposed as a method to gather data on highly rare, but clustered populations. The method gained popularity in ecological fields: imagine a botanist attempting to measure a rare plant species. If a researcher were trying to generate data on this rare plant population by randomly selecting areas of a set size within a plot of land, a simple random sampling (SRS) would be inefficient. Consider that you, the researcher, randomly select a given area (with a set size) and encounter the rare plant species in this area. A standard SRS approach would mean that such a rare discovery would not change the chance of selecting any subsequent area to discover more occurrences of this rare species. You may be thinking that to use SRS in such a situation would be inefficient. You, the researcher, have found the rare species you have been looking for! So, why not explore nearby?
This intuition is what motives ACS. In this sampling method, the discovery of some occurrences of a ‘rare’ species propagates further exploration in nearby areas. Several existing areas of research have shown that such informed exploration can be unbiased and generate substantial gains in fieldwork efficiency.
In our paper, we show that ACS can be applied to informal businesses. Although this population is not very rare, when using ACS, it can be measured more efficiently because it is clustered. Using a unique dataset of geocoded census data on businesses, including informal ones, from Eswatini, our paper shows that generating population estimates through a survey of informal businesses often requires substantially less effort of fieldwork than, say, a simple random sample of a specified geographic area (typically, an urban area). Additionally, we show that for a given amount of fieldwork, the precision of the population estimator is often significantly higher using ACS compared with SRS. Since we have census-level data on the total population of informal businesses in Eswatini, we can compare our ACS-generated estimates of the total of that population to SRS comparators. Both ACS and SRS estimates of this population “total” are un-biased. However, the important finding for many researchers and survey implementers is that ACS-based estimates require substantially less fieldwork than SRS-based ones. Generally, estimates from ACS surveys are also less variable.
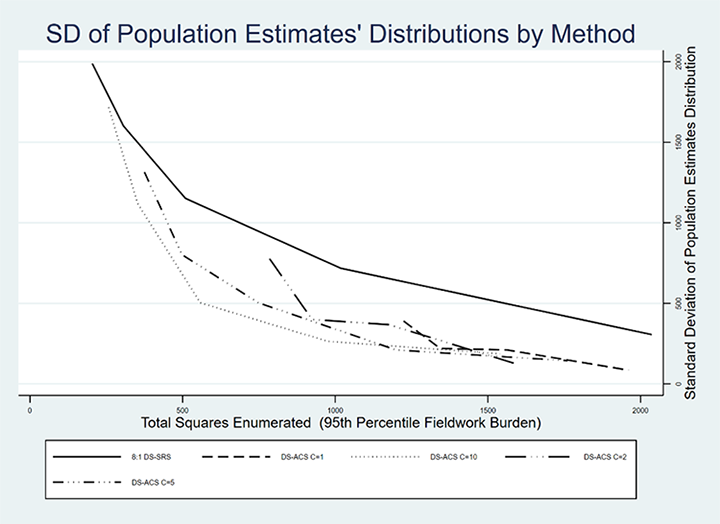
Why are such findings important? First, we show that ACS-based methods are comparatively less expensive because they reduce the costs of gathering information on key populations of interest Secondly, we provide practical implications that impact the choices that survey implementers will need to make under budget and time constraints. The efficiency gains generated by using ACS requires many practical decisions around survey management. Accordingly, our paper puts forth some general parameters for making these decisions.
Lastly, while our paper shows a generically applicable series of results, the World Bank is currently using such cost-efficient survey approaches to fill the data gap on recording data on informal businesses. To date, the methodology, under the Informal Sector Enterprise Surveys, has been applied in eight countries, covering 24 cities, interviewing about 15,000 informal businesses randomly selected from over 80,000 businesses that were enumerated in close to 22,000 blocks of 150 by 150 meters. There are eight other countries where data collection is currently underway. The provision of this public good allows academics and policy makers to conduct more in-depth analyses to deepen their understanding of some of the key issues around surveying informal businesses.
Join the Conversation