Motivation
Nothing keeps development economists awake at night like yield gaps across continents. While these gaps have persisted, lessons from countries that have closed these gaps suggest that massive adoption of a new technology can lead to rapid diffusion and productivity growth. Reaching that critical mass is a key constraint — in simple terms, learning a new skill without your friends is hard! A deep literature studies technology adoption in agriculture. Despite some good effort, results are tepid — yield growth has stagnated in many regions.
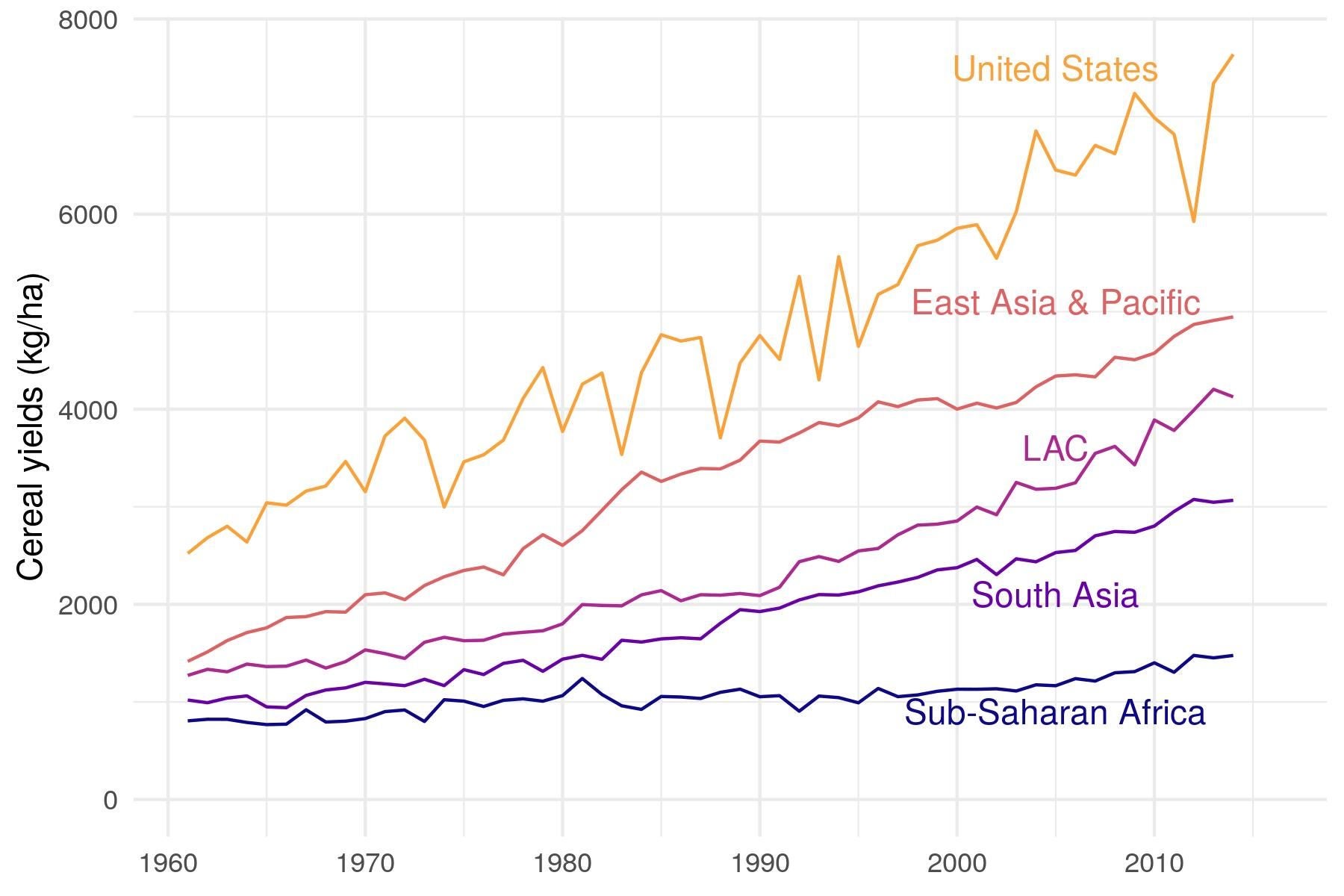
In the information age, a world of advice is at our fingertips — the recent explosion of sourdough production in the United States is strong evidence of the availability, even if not the value, of information. This expansion has benefited developing countries as well: in our field of research, smallholder farmers have rapidly adopted mobile phones not just to connect with friends and family, but also to access information on new agricultural technologies. But this motivates an important question: are farmers learning anything from these extension services development economists keep wanting to study and talk about?
Answering this question is hard — as readers of this blog are familiar with, the easiest way to estimate the impact of extension services on learning would be to randomize them across communities. Such randomization is rarely feasible, as globally many countries have rapidly scaled national agricultural extension services, limiting remaining scope for randomization. This motivates difference-in-differences approaches: can we estimate the impact of the expansion of agricultural extension services without randomization? But writing a careful difference-in-differences paper is hard, as we've learned recently there are … so … many … pitfalls! What should we do?
There is a lot to like about the new Gupta, Ponticelli, & Tesei (2020) working paper. First, they shed light on the role of extension services in increasing agricultural production, leveraging the expansion of coverage under call centers. Their “treatment” of interest is cell phone tower construction (which enables smallholders to call into call centers for agricultural advice), and their primary outcomes of interest are use of the agricultural extension services, adoption of modern agricultural inputs, and farmers’ productivity.
Second, the way they set up their difference-in-differences strategy provides a textbook example of how to establish the credibility of difference-in-differences estimates of causal effects. In the rest of this blog post, we describe the five key steps this paper takes to this end.
Quasi-experimental variation in “treatment”
The key assumption in a difference-in-differences design is “counterfactual parallel trends”: absent treatment, units receiving treatment would have experienced the same changes in outcomes that units receiving control actually experienced. In this paper, this means that, absent cell tower construction, communities receiving new cell towers would have experienced the same increases in farmers’ productivity as communities not receiving new cell towers. In general, this assumption is more credible when we don’t think that treatment would have been assigned based on potential changes in outcomes.
To support this assumption, this paper leverages the roll out of new cell phone towers through the Shared Mobile Infrastructure Program. In this context, the “counterfactual parallel trends” assumption requires that construction of cell phone towers did not target areas that would have otherwise experienced relatively high growth in farmers’ productivity. To make this assumption more credible, this paper does three things:
- They explain what was targeted (low ruggedness, high population density), and control for these characteristics.
- They use locations with planned cell phone towers as a control group for locations with actually constructed towers, which is more likely to be a valid control group than locations never considered.
- They interact the roll out of new cell phone towers with whether extension services are offered in the local language.
In sum, having a clear explanation of why we might expect the rollout of treatment to be “as-good-as-random” (with respect to changes in outcomes that would have happened absent treatment) makes the difference-in-differences design more credible.
Event study for outcomes observed at high frequency
In most difference-in-differences papers, most outcomes are observed in low frequency surveys, which makes the event study graphs from data rich settings a source of envy. However, it’s often the case that a subset of outcomes are observed at high frequency. Examples of this include where administrative data covers an intermediate outcome of interest, or when remote sensing data is viable.
In this paper, the call centers themselves provide a source of data on smallholders interactions with agricultural extension, as the call centers record the subdistrict of origin, the time, and the topic of each call. The high frequency of this data makes an event study design (discussed previously on this blog here and here) possible — the frequency of calls to the call center can be compared in months just before and just after the construction of cell towers. This enables flexible testing of the parallel trends assumption by comparing changes in the frequency of calls before tower construction in treatment and control communities. Although this flexible test is not possible for all outcomes, implementing it for a subset of outcomes improves the credibility of the design.
Placebo event study
Testing for parallel trends before treatment provides one approach to testing the difference-in-differences assumption. However, an alternative approach is to provide a placebo test: identifying a set of communities where treatment should have no effect (or at least a very small effect). Common examples of this include using initial plans that were never implemented (although this can also be used to construct a more comparable control group), or use alternative definitions of treatment (e.g., placebo thresholds in a difference-in-regression discontinuities design).
In this paper, the call centers offered advice in the 22 official languages in India, but many rural households communicate in non-official languages. This paper therefore proposes that use of the call centers should be much higher in communities where official languages are spoken, compared to communities where non-official languages are spoke. It finds exactly that — they estimate the treatment effects are much larger on use of the call center, technology adoption, and farmers’ productivity in communities where official languages are spoken, and that effects all but vanish in communities where non-official languages are spoken. As this placebo check is consistent with the prior that call centers have limited impacts on communities without language overlap, but is not consistent with the prior that cell tower construction targeted communities that would have experienced growth in productivity even without tower construction, it improves the credibility of the design.
Parallel trends for outcomes and baseline balance for covariates
Even when outcomes are observed at low frequency, tests of parallel trends are still possible when multiple waves of data are available before treatment begins. In practice, this involves comparing changes in outcomes that occurred before treatment began, in communities that eventually became treated relative to communities that were not treated. This is likely to be feasible in contexts where data is collected at regular intervals, such as census or nationally representative household surveys. However, even if one passes this test, it is also more believable that communities will continue to experience parallel trends if communities are observably similar.
In this paper, both changes in key outcomes before tower construction and characteristics of communities are compared for treated and control communities. As mentioned above, it finds that low ruggedness and high population density predict tower construction, consistent with how tower construction was targeted. However, other characteristics and changes in key outcomes before tower construction fail to predict treatment. The combination of parallel pre-trends in key outcomes and pre-treatment balance in key characteristics both improve the credibility of the difference-in-differences assumption.
First stage and intermediate outcomes
Even with a myriad of placebo tests, it’s always possible that the estimated effects of treatment were caused by an unusual shock to outcomes. In this case, tower construction may have coincided with an unusual shock to farmers’ productivity. However, an unusual shock to multiple outcomes at once in a manner consistent with a well specified theory of change is much less likely. Demonstrating precise impacts on first stage and intermediate outcomes along the theory of change, with decreasing precision as more downstream outcomes are considered, is typically what one would expect if the treatment caused the observe changes, and is less likely to be consistent with an unusual shock. In addition, a good check is to verify that magnitudes of impacts on downstream outcomes seem reasonable given estimated impacts on upstream outcomes.
In this paper, the key theory of change is that cell tower construction reduces households’ costs of calling into call centers, households ask for advice on modern agricultural inputs, they adopt these inputs on their plots and in turn see increases in production. Consistent with this, they estimate impacts on calls to call centers, adoption of high yielding varieties, and increased farmers’ productivity. While estimates are extremely precise on upstream impacts (calls to call centers), precision decreases (while remaining statistically significant) on more downstream impacts (adoption of high yielding varieties, productivity). Together, these patterns improve the credibility of the estimates, as they are harder to dismiss as driven by unusual shocks.
Summary
In sum, these approaches are all helpful for making the research design in difference-in-differences papers more credible. However, new work has suggested some non-robustness in difference-in-differences designs that deviate from the canonical model: where all units are untreated in a pre-period, and a subset of units are treated in a post-period (note that this paper falls under this canonical model). However, these approaches complement rather than substitute the new machinery, much of which develop approaches to estimation that break down multi-period panels with multiple waves of treatment into a series of simpler cases. Stay tuned for a future blog post on these new approaches!
Join the Conversation