How to do Implicit Association Test?
Implicit Association Tests (IATs) are being increasingly used in applied micro papers. While IATs can be found off-the-shelf, designing your own IAT may allow you to get at respondents’ implicit attitudes towards something more contextual. We added a custom IAT to a survey of commuters in Rio de Janeiro, and here we'll go over the practical steps involved. For our project, we wanted to measure male and female commuters’ implicit attitudes towards women riding the subway on the co-ed car relative to women riding the women’s-only car. The idea was to quantify the stigma women may face for not using gender-segregated spaces.
What is an IAT?
The idea behind an IAT is to measure a respondent’s implicit attitudes. While asking how they feel about something returns a person’s explicit attitude and may be affected by response biases, the IAT aims to reveal implicit attitudes. A series of stimuli (words and/or images) is presented to each respondent, who must sort them into two categories. The key assumption underlying any IAT is that the stronger the association a respondent makes between two concepts, the faster they are to make these associations.
Each individual IAT includes several training rounds, a stereotypical (“easy”) paired test, and a non-stereotypical (“hard”) paired test. At the top of the screen are the two categories in which stimuli need to be sorted with a keystroke to the right or left (cf. Figure 1). A stimulus can be words or images, presented in the middle of a monitor. A standard, off-the-shelf gender-career IAT would include the following rounds:
- Two initial training rounds, where respondents practice sorting stimuli into two categories of the same concept: respondents categorize words (e.g., parents) into career versus family (1a). They then categorize male and female names by gender (Figures 1b).
- The "easy” paired test uses stimuli from a combination of the first two lists. Respondents must categorize concepts on the same side that are stereotypically associated: In Figure 1c, men and career is on the left, and women and family is on the right.
- Another training round, in which the respondent practices swapping right and left for one category.
- The "hard" paired test: the respondents see the same stimuli but must place categories on the same side that are not stereotypically associated (women and career; men and home – Figure 1d).
It is important to note that the IAT measures a “gut reaction,” not behavior, which may be a product of both implicit attitudes and explicit decision-making. As Betrand and Duflo (2016) point out, while some studies find a that individual IAT scores correlate meaningfully, on average, with hiring ( Rooth 2010, Reuben 2014), grading ( Alesina et al 2018, Carlana 2018), voting ( Arcuri et al 2008, Raccuia 2016), and clinical decisions ( Green et al 2007), in other studies, they have not ( Oswald et al 2013). At the individual level, the score is not deterministic of that person’s actions.
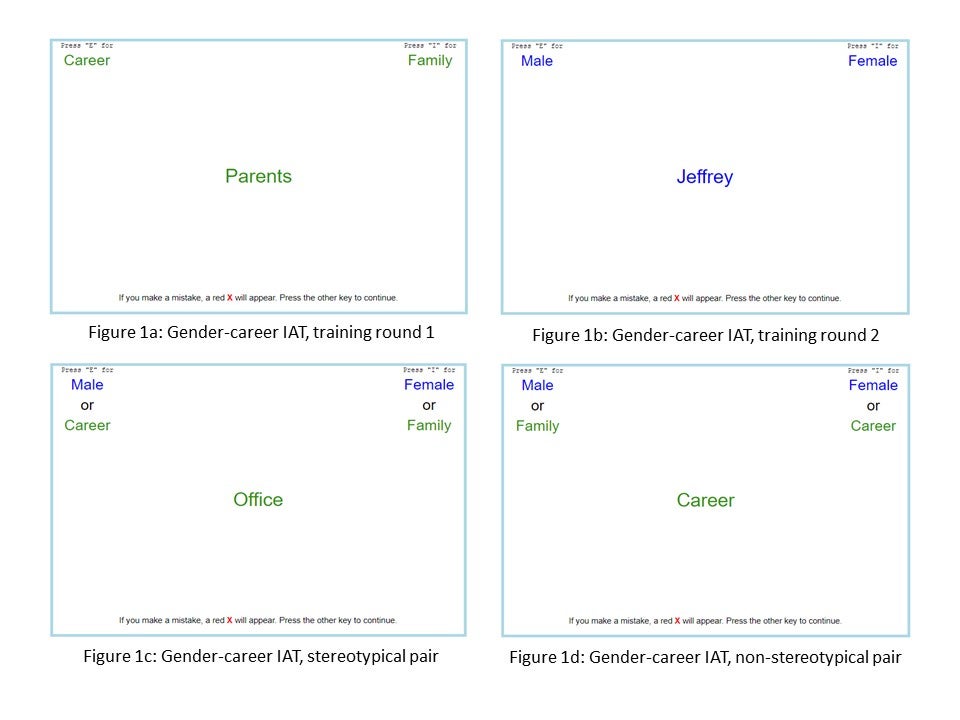
Design a tailored IAT
In our work on women's-only transit cars in Rio we wanted to test whether commuters associate women riding on the co-ed car with greater openness to sexual advances. In practice, we test the relative strength of association between women riding the co-ed car or the women’s-only car and words implying sexual openness or provocation. These are the main steps we took to design them:
- There is such a thing as Right or Wrong. Each categorization task must have a clear correct answer that is easy to recognize quickly. This is very important, as the IAT relies on differences in the speed in “gut reactions”. If a respondent takes too long on a stimulus, that observation is dropped from the score (Greenwald et al 2003). Being able to compute an error rate also becomes key in testing and trimming out outliers (more on this below).
- Stimuli. For the first set of stimuli, we chose pictures of the women’s car in which the pink “women’s car” labels were prominent. We confirmed in pre-piloting that our commuter sample were familiar with this image and the policy it indicates. We also made sure that the women’s-only car stimuli only differed from the co-ed car stimuli on that one dimension. We chose pairs of photographs that had similar crowding, lighting, and angle.
- Categories. Similar steps are required in selecting the words used to identify the association concepts, or categories. It is important that these words belong to similar levels of language (e.g., formal vs informal) such that differences in reaction times can be attributed to differences in concepts. Also important is to eliminate high-level vocabulary that not all participants might easily understand, and words that are easy to misread on screen and avoid offensive words.
- Identify benchmarks. Like many observational measures, the IAT could proxy for other things that differ from the implicit attitude we are interested in measuring. For example, our women’s-only car IAT could simply be picking up the general perception that women should stay at home. Two things can help rule out competing mechanisms.
- Design a companion IAT to test an alternative hypothesis: in our case, we devised an IAT to measure association between women on the women’s-only car and safety.
- Add a standard off-the-shelf IAT to your instrument: In our case we used the gender-career IAT.
- Finally, it is a good idea to survey explicit attitude measures for comparison. We included agreement questions such as “women on the co-ed car are more likely to accept advances” and “women are partly at fault if harassed on the co-ed car”.
Set up the IAT tool
We implemented the IAT instruments with the easy-to-use software developed by Meade (2009). The software which calculates the main outcome of interest (D-score) following a standard methodol ogy. The full “openness to advances” IAT is shown in Figure 2: it first proceeds with training rounds in which respondents categorize words like “certinha” (“prissy”) and “provocante” (“provocative”) into “likes advances” (“gosta de cantadas”) / “does not like advances” (“nao gosta de cantadas”) (Figure 2a), and then categorize pictures of the women’s and co-ed cars (2b). In the “easy” paired trial (2c), the categories “likes advances” and “co-ed car” are listed on the right, and “does not like advances” and “women’s car” on the left. Then participants take the “hard” paired trial (2d) in which the categories are “mismatched”.
Pilot
As with any survey tool, piloting is essential. There is no science here, unfortunately—we look forward to readers’ suggestions to improve this.
- If respondents are, on average, faster to understand one group of stimuli than another, or make more mistakes on it, this is a sign that the IAT design may not be working well. This might be due to a poor choice of words or stimuli, or simply poor understanding of what the local context and stereotypes are.
- Comparing average mistake rates and average response time across all IATs serves as a good benchmarking exercise, especially when off-the-shelf instruments are added. The rate of mistakes on the off-the-shelf IATs was within an acceptable range, and higher for harder matches relative to easy ones (panels a and b, Figure 3). It was also reassuring to see that error rates on our IATs were on the same order of magnitudes as those on the off-the-shelf ones (panels c and d, Figure 3).
- It is useful to pilot different stimuli and concepts. We tried different versions of our tailored instruments to pilot weaker vs stronger concept words for reputation. Panels c and d of Figure 3 show the relative rate of mistake of those two versions on easy (stereotypical) vs hard (non-stereotypical) matches. Respondents’ rate of mistakes reverses in relative terms across our versions, signaling that the stronger language version performed better at getting at the expected stereotypes.
- Screening and adapting for literacy rates of your target population is important. Most of our sample were literate, but those who were not had to be excluded. In other contexts, researchers have adapted the IAT to use all picture stimuli. The pilot can help you account for this expected failure rate and budget for your field work, as it amounts to throwing out potentially large shares of your data.
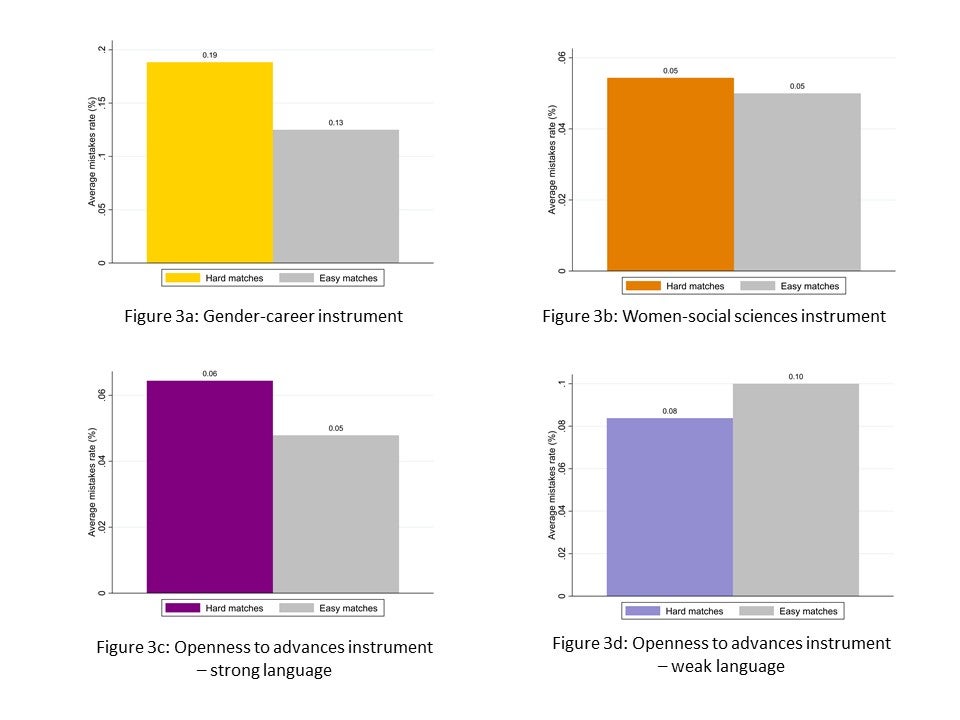
What we found
We find that Rio commuters associate “openness to advances” more with the co-ed car than the women’s-only car. This is true for male and female commuters. We also find that this association is stronger than the association between safety and the choice of car (our benchmark IAT). The stated opinion questions also echo these results: almost half the sample agrees that “women on the co-ed car are more likely to accept advances,” and one in five agrees that “women are partly at fault if harassed on the co-ed car.”
For robustness, we control for the gender-career IAT score in our analysis. This does not affect our results from our tailor-made IATs – suggesting that our IATs are picking up on views specifically about the women’s only car in this context.
Finally, you can try out a number of standard IATs and see your scores at projectimplicit.org. We recommend this as a first step before designing your own IAT!
Join the Conversation