During the past 20 years, a growing body of research has shown that socioemotional skills (SES) can determine improvements in academic success and educational transitions, cognitive development, participation in risky behaviors, engagement in healthy decisions, and labor market outcomes. In my area of work, which relates to psychology-based interventions that aim to reduce school-based violence of adolescents in vulnerable contexts, SES and emotion regulation are either the main outcomes or mechanisms that we measure to determine whether the interventions that we study are successful.
Most evidence on SES is obtained through self-reported questionnaires. However, self-reporting can entail the risk of reporting bias. As first pointed out by Egana del Sol (2016), this issue becomes more noticeable when we use self-reports to collect information to evaluate programs that are designed to improve SES because, in this case, it isn’t possible to assess the direction of the bias. For example, it is possible that individuals who have participated in an intervention might downplay their skills on a questionnaire because the program made them more aware of the degree of their skills, and they might feel that they haven’t yet reached a proficient enough skill level. On the other hand, intervention participants might overreport their skills in order to prove that they have learned something from the intervention. One way to address the self-reporting bias in SES data is controlling for social desirability scores. Another alternative is to collect objective measures of SES, that is, data that is not based on self-reports from participants of the study.
I have partnered with brilliant coauthors to identify alternative and innovative tools to measure SES and emotion regulation in the field. These tools include task-based games and artificial intelligence (AI)-powered emotional detection algorithms, which can be used in school contexts with minimal infrastructure. Below I describe what tools we have used so far as well as their advantages and disadvantages.
Measuring emotion regulation using electroencephalograms (EEG)
In Dinarte-Diaz and Egana-DelSol, 2022, we conducted a novel and exploratory analysis of emotion regulation as a possible mechanism behind an after school program’s effects on the participants’ behavior and academic performance. We argue that the ability to consciously govern one's emotional and physical responses to stimuli—otherwise known as emotion regulation—interrupts negative automatic responses that lead to violence and, consequently, fosters more deliberate decision-making and behaviors. To monitor emotion regulation, we studied the intervention's effects on two neurophysiological biomarkers, arousal and valence, which the psychology literature treats as proxies for “alertness” and “emotional self-regulation to positive or negative stimuli,” respectively.
We used electroencephalogram (EEG) recordings to measure emotional state at rest (i.e., no stimuli) as well as responses to positive and negative stimuli. We relied on the portable Emotiv EPOC headset, which is an advanced, cost-effective, and reliable tool that can be used in the field in real-life contexts.
To take our measurements, we established a lab-in-the-field setting (See Figure 1 as a reference). For each participant, we first set up the headset (see this tutorial for more details). Second, we asked the participant to look at a computer screen depicting a black cross in the center of a gray background for 30 seconds while we used EEG to estimate the measurements of the biomarkers at rest directly via the participants' scalps. Then, we showed participants some images from the Geneva Affective PicturE Database (GAPED). These images served as the external positive and negative stimuli that we used to measure how students’ brains responded after being exposed to them. Then, we relied on a simple computational neuroscience model of emotions (Egana del Sol, 2016) to capture the two neurophysiological markers arousal and valence.
Figure 1. Lab-in-the-field set up for EEGs.
Although it is relatively easy to use EEGs in the field, the process is complicated in other ways. For example, field staff who run the experiments should have an electrical engineering background. It also takes time to learn how to attach electrodes to the participants’ scalps, which is an important limitation when it is necessary to collect data in a short period of time at a location like a school. Interestingly, we also learned that the EEGs do not attach properly to dirty hair, so we had to reschedule a couple of sessions with students who had PE before coming to our lab!
Using AI-based algorithms to measure emotion regulation
Although the EEG tool is relatively easy to transport to the field, it still requires specialized field staff that are not necessarily easy to find. For this reason, in Dinarte-Diaz, Egana-DelSol and Martinez (2022), we borrowed a tool from the scientific field of affective computing (AC) to collect measures to proxy for emotion regulation. The AC tool we used to proxy emotion regulation was Reactiva, a smartphone application based on an AI-powered algorithm co-developed by Egana del Sol in collaboration with Affectiva, a spinoff of the MIT Media Lab’s Affective Computing Group. Similar to Egana del Sol (2016)’s protocol, we constructed arousal and valence indices at the onset of positive and negative stimuli.
How does Reactiva work? In a nutshell, first, we gave each participant a tablet on which we played emotional-laden videos from GAPED to produce the stimuli. While participants were watching the videos, Affectiva’s technology observes and identifies key landmarks on the face via the front camera of the tablet. The machine learning algorithm then analyzes the pixels to classify the facial expressions using Facial Action Coding Systems (FACS). The combination of these facial expressions is then mapped out and the emotion predictors calculate the likelihood of an emotion. The success rate of these algorithms in predicting emotional states is around 75–80%. This tool requires a strong internet connection, high quality equipment (tablets with good front cameras), and rooms with excellent lighting.
Figure 2. Collecting emotion regulation using Reactiva
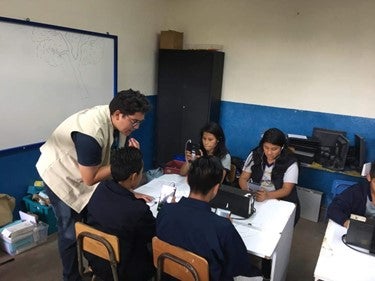
Task-based games to measure SES
In Dinarte-Diaz, Egana-DelSol and Martinez (2022), we also collected data on SES using task-based games embedded in a software called SoftGames. Danon et al. (2018) developed this application in coordination with the Center for Economic Research in Pakistan (CERP). We collected three SES that were relevant to the intervention that we were evaluating: perseverance, self-control, and risk-taking behaviors. Below I define the skill and briefly describe the game we used to measure the skill.
Perseverance is a continued effort to do or achieve something despite difficulties or obstacles. To proxy for this trait, we estimated a measure of short-term persistence using the Additions Game. In this game, participants are given a tablet that shows a set of additions that are easy or difficult to solve. After each round, the participants are asked to choose the difficulty level of the next round. The outcome is measured as a dummy that equals 1 if a participant persists after failing in round 1, which was intentionally programmed to be difficult to see if the participant would continue to the second round.
Self-control is a trait defined as the tendency to avoid acting suddenly without first considering about the consequences of an action. We estimate this trait using the Go-NoGo task-based game, which measures the player's ability to inhibit an “inappropriate” response determined by the Go-NoGo rule. Specifically, the participant is presented briefly with a square on the screen. If the square is not black, the participant must touch the screen as quickly as possible (the “Go” rule). If the square is black, then the respondent must refrain from touching the screen (the “noGo” rule). A total of 72 trials are presented. The score is the number of times a participant responds correctly to the “NoGo” stimulus.
Risk-taking behavior consists of any consciously controlled or unconscious behavior with a perceived uncertainty about its benefits or detriment to the well-being of oneself or others. To measure this trait, we used the Balloon Analogue Risk Task (BART). Participants were asked to maximize the number of points they can earn by blowing up a balloon. While they earned points for every pump, they could also lose all of their points if the balloon popped. The outcome was measured as the mean number pumps for a balloon that did not pop: The greater the score, the less risk-averse is the individual.
Based on our experience, the main disadvantage of collecting data using these games is that an intensive piloting is necessary, especially when the data will be collected from a diverse sample. In our case, we dealt with students between the ages of 10-16 years, so instructions had to be age-appropriate. It is also important to identify appropriate incentives for the participants, particularly in schools that do not allow monetary incentives.
I am always eager to test other methods and tools to collect objective measures of non-cognitive skills that are easy to bring to the field or to school settings and that can also support the evidence base. Please feel free to reach out if you have any more tools or ideas to suggest!
Join the Conversation