This is the 13th in this year’s series of posts by PhD students on the job market.
The adoption of new technologies relies on first acquiring information about them. Will this technology make me better off? Frictions to obtaining information are a key barrier to higher agricultural technology adoption in developing countries (Magruder, 2018). Two common channels of information about new technologies are peers and formal outreach, such as through extension programs. These two sources of information have a huge data gap. Peers often have limited experience with a new technology, having tried it on perhaps a single plot during a single season before providing their recommendation. By contrast, extension programs advocate technologies which are often first rigorously tested by sources such as universities or national agricultural research institutes (Leeuwis, 2015), and thus have more observations about a technology’s efficacy. Despite this data gap, prior field experiments show that recommendations from peers induce just as much adoption as recommendations from extension agents (Krishnan and Patnam, 2014; Takahashi, Mano, and Otsuka, 2019). This implies that social learning, where recommendations are backed by very limited experience, is more effective per observation than the more thoroughly vetted recommendations by extension agencies.
What is the mechanism behind this? Perhaps more importantly for policy makers, is there something special about information from peers that cannot be replicated, or can traditional information provision be modified to be more impactful? My job market paper formalizes one possible mechanism, which I label context uncertainty.
What is context uncertainty?
Technologies often have heterogeneous returns. In agriculture, this can be due to a myriad of reasons, such as soil content, weather patterns, or even access to a non-fraudulent version of the product itself. When we receive a recommendation from a peer, their signal is implicitly paired with information about their context. I likely know a fair amount about how different my friend’s soil is to my own. Thus, when I get a recommendation from them, I know how to adapt it to my own context. For example, if rainwater catchment worked well for my friend, and I have drier land, I can infer that rainwater catchment will be at least as beneficial for myself.
By contrast, when I receive a recommendation from an extension agent, I’m not given information about the conditions of the test plot or lab conditions. (The extension agent may not know themselves!) Even though it worked well during testing, I don’t know how to adapt that signal and infer whether the technology will work well on my own land.
If we take into consideration the uncertainty created by not being able to adjust for context, we can think of the total uncertainty in a recommendation as:
Total Uncertainty = Sampling Error + Context Error.
A rational individual will weigh signals not just based on sampling error, but total uncertainty. If they are risk averse, like many small and marginal farmers, that will cause them to adopt less when total uncertainty is higher, like with the recommendations from extension agents.
Testing Context Uncertainty
Isolating context uncertainty to identify it is difficult. I do so by designing a lab-in-the-field experiment with 1,571 small and marginal farmers in Odissa, India. The experiment consisted of a mobile game about agriculture, where the participant’s decision was to decide how intensively to adopt a hypothetical technology to maximize yield.
Before making their decision, they received information from forty fictional characters in the game who tested the technology last season. They do so using a 7 point Likert Scale, reported using emoji. These characters had one of two types of land: the blue type or the orange type. All characters of the same type have the same context, and thus have the same underlying return to the new technology. However, two characters of the same type may still report different signals due to idiosyncratic risk due to sampling error, i.e. misapplication.
The participant is told that he is, himself, the orange type. He is also told the exact difference between types: blue types always report two units sadder on the emoji scale than orange types. This is paired with a story about a sample technology, explaining that the technology isn’t any worse, it is just less valuable to blue types.
So, where is the context uncertainty? Some fictional characters will provide a signal but the participant won’t know their type. They appear as gray. Gray characters have an equal chance of being blue or orange. Context uncertainty arises here because the participant should adjust signals from blue characters but not for orange characters. He will not know which to do for gray characters.
Each participant plays multiple rounds of the game, and I randomize round order and technology name across participants. In the two rounds used to test my main hypothesis, I vary context uncertainty by changing the percentage of gray characters, but keeping the distribution of signals the same.
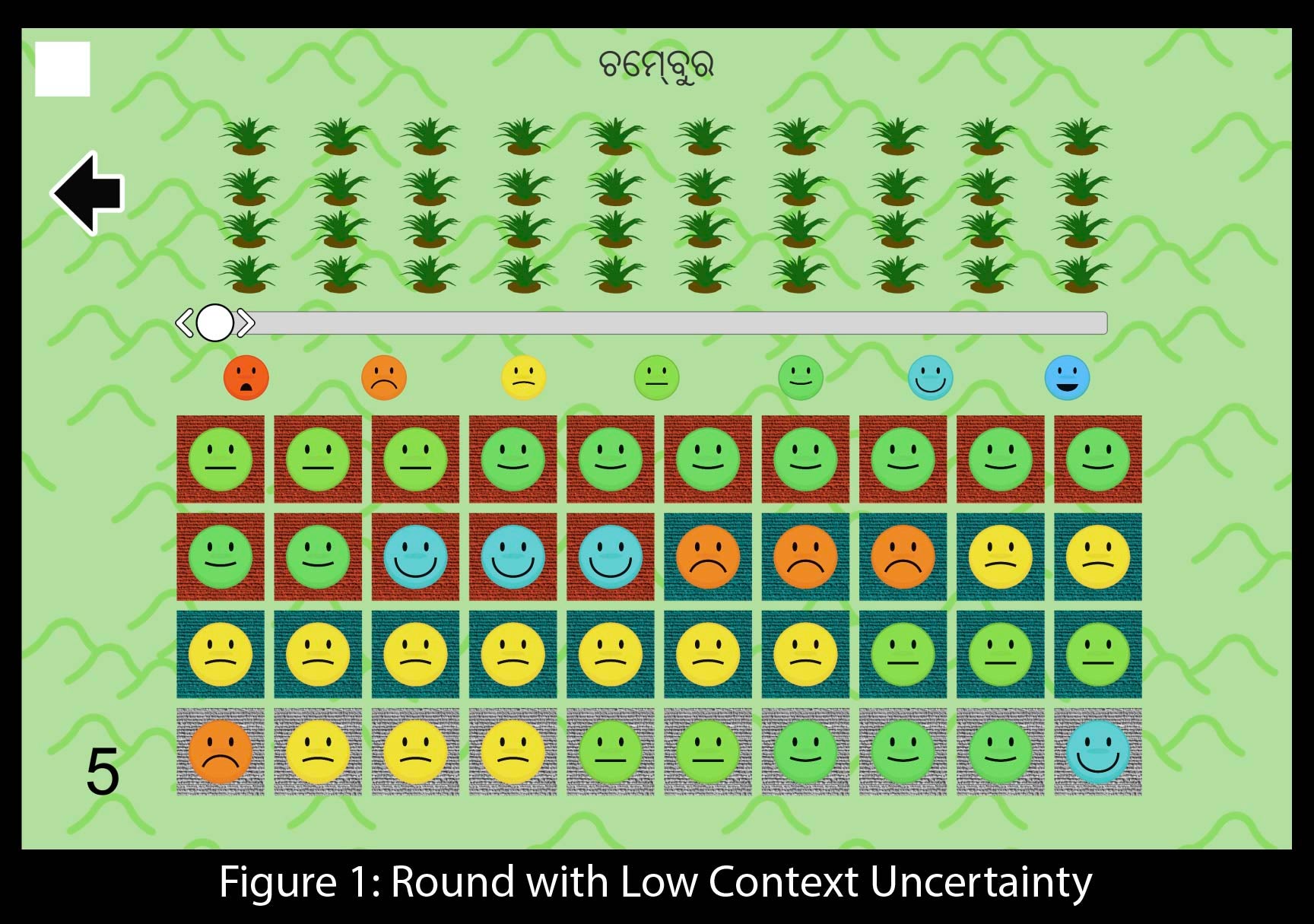
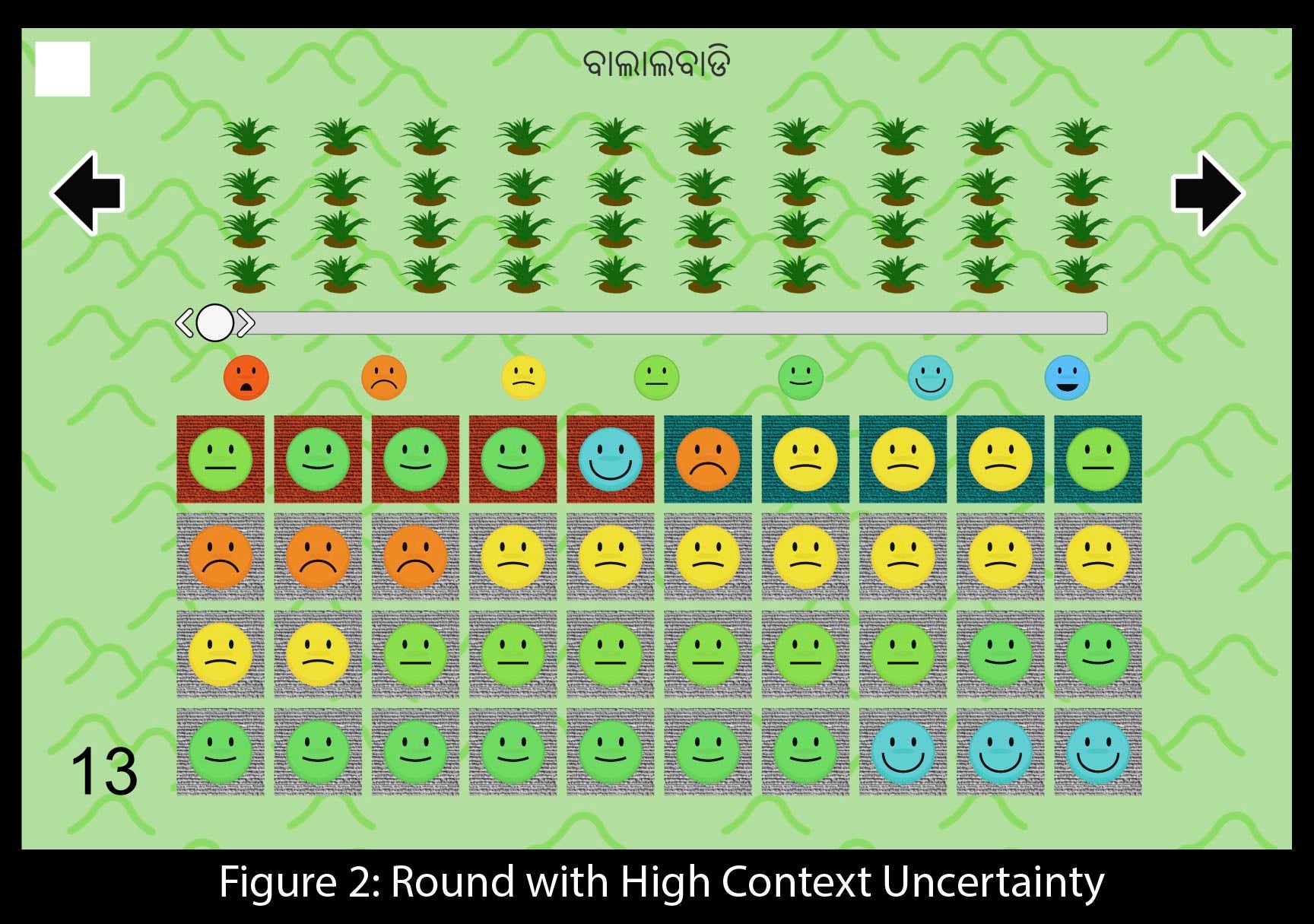
Results and External Validity
Using this design, I find that farmers adopt a technology less when there is higher context uncertainty. This coincides with survey evidence I gather from the same farmers. Over 40% of participants report concerns having to do with heterogeneity as a reason why they value a peer’s recommendation more than that of an extension agent. I also asked farmers about the last recommendation they received from an extension agent. Just under 38% of respondents reported that they did not put more weight on this recommendation for reasons having to do with heterogeneity, such as soil, inputs, technology quality, or a gap in training compared to test conditions. These responses were also correlated with observed context uncertainty aversion within the lab experiment.
Implications
Prior research has already explored a variety of designs for extension programs. A common thread across many of them, most notably the push towards demonstration plots, is the reduction of context uncertainty. By understanding the mechanism driving these results, future programs can hopefully be directly tailored towards reducing context uncertainty. Further, though providing low basis risk insurance at scale can be costly, providing it to a small subset of households who agree to local early adopters has the potential to generate positive information externalities for the entire community.
Hossein Alidaee is a Ph.D. Candidate in Economics at the Kellogg School of Management, Northwestern University. More details about his research can be found on his website.
Join the Conversation