As extreme weather events intensify and global health and economic crises threaten the progress in eliminating poverty and in achieving the Sustainable Development Goals (SDGs), the need to measure the impacts of shocks on households is greater than ever. Household surveys, a key source to track progress towards SDGs for more than a third of its 234 indicators, play an important role in understanding the impact of data-driven policy responses.
Yet, national statistical offices (NSOs) in low- and middle-income income countries struggle to meet this ever-growing data demand. Response rates to household surveys decline; lengthy questionnaires contribute to respondent fatigue, hence lower data quality; and coordination fails within the overburdened statistical systems. COVID-19 further exposed the vulnerability of household surveys as almost all countries stopped the data collection in May 2020. NSOs also face a complex landscape of new and seemingly competing sources of data as data collection technologies evolve. A bold vision is needed to transform household survey systems to achieve the SDGs .
In a new research article by the World Bank’s Living Standards Measurement Study (LSMS) and the United Nations Statistics Division, under the aegis of the Inter-Secretariat Working Group on Household Surveys, we identified eight technical priorities for household surveys in the next decade. The priority areas were chosen based on three primary criteria: (1) areas that have been proven to be successful or have a great potential to make a medium-term impact; (2) areas that both build a strong data foundation and expand the frontier for research and development; and (3) areas that are more likely to benefit low- and middle-income countries, where improvements are needed the most.
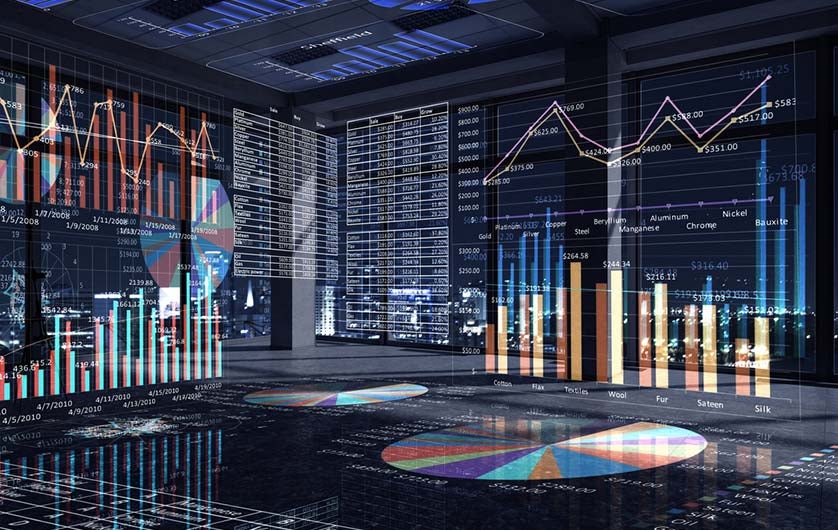
-
Enhance integration of household surveys with both traditional and new data sources
Integrating survey data with censuses, geospatial data, administrative data and other sources can increase both the policy relevance and cost-effectiveness of survey data production. The success depends on having harmonized concepts and definitions across different data sources, improving access to other data sources on the part of survey data producers, fostering data interoperability by design, and maintaining data confidentiality. There is also a need for establishing a total quality framework for data integration such that we can distinguish and quantify errors arising from non-traditional data sources versus those emerging during the integration process.
-
Design and implement more inclusive, respondent-centric surveys
Declining unit and item response rates diminish the quality of household surveys. The reliance on proxy respondents while collecting individual-level data is a related area of concern. These challenges can be tackled by transforming respondents into collaborators and co-producers, and adopting fieldwork protocols that maximize the rate of self-reporting among adults that provide personal information.
-
Improve sampling efficiency and coverage
Continuous improvements to sampling frames and the adoption of innovative sampling techniques are key. For frames, these might include geocoding census records, leveraging high-resolution satellite data when the census frame is outdated, and building an integrated sampling frame to improve coverage.
For populations that are difficult to reach, oversampling and network sampling have been used to improve coverage while machine learning models could assist with more targeted sampling. With advances in electronic data collection, responsive and adaptive sampling designs can save costs through real-time design decisions during data collection.
-
Scale up objective data collection methods to address measurement errors
Policy analysis based on household survey data will be biased if there are systematic measurement errors that may be driven by recall bias, strategic misreporting, and social desirability bias, among others. Methodological research over the last decade has advocated for direct measurement tools to increase the accuracy and scope of survey data collection while also reducing respondent burden. These include GPS technology for plot area measurement, DNA fingerprinting from crop variety identification, and low-cost testing kits for water quality assessments, among others.
Although procuring these tools can be costly—which may make a full-scale adoption of direct measurement difficult, the adoption can be limited to a sub-sample and within-survey imputation approaches can be pursued to derive imputed estimates for the sampled units that are not subject to direct measurement.
-
Strengthen capacity for CAPI, phone, web, and mixed-mode surveys
The halting of face-to-face surveys during the COVID-19 pandemic has exacerbated the need to advance phone, web, and mixed-mode surveys. While face-to-face surveys will continue to be relevant, there is a need to strengthen remote data collection, specifically in low- and middle-income countries. For instance, using phone and web surveys together with face-to-face surveys would enable quicker responses to data needs during emergencies or in their aftermath.
-
Systematize the collection, storage, and use of paradata and metadata
Paradata are being collected by advanced economies as a byproduct of the data collection process, including keystroke records and GPS-tracking of interviewer location. Unfortunately, the use of paradata to improve data quality is generally scarce in lower-income countries. In the future, better research and experiment are needed on the use of paradata collected as part of CAPI and CATI systems implemented in lower-income contexts.
Metadata such as the date of interview, identifiers for replacement households, and reasons for replacements also play an essential role in monitoring the progress and quality of data collection, as well as in conducting ex-post research such as on interviewer effects.
-
Expand capacity for machine learning and artificial intelligence
AI, machine learning, and predictive analytics can improve efficiency in every step of survey operations. For example, machine learning can automatically code open-ended responses. Classification Trees can build a predictive model with existing information from surveys and administrative data, making sampling of rare population groups more efficient. The use of AI and machine learning has also been central in applications that integrate household survey data with new data sources, such as high-resolution satellite imagery or call detail records.
However, applications of AI and machine learning in household surveys are still concentrated in countries with more advanced statistical systems. Building capacity in machine learning and AI for NSOs of lower-income countries should be a priority.
-
Improve data access, discoverability, and dissemination
Household surveys for development reach their greatest value if they are made available for use and reuse. Looking forward, data producers should aim to publicly disseminate household survey datasets in a timely manner. Providing secure access to confidential survey microdata should also be considered to promote further use and research.
A bold vision is needed to transform household survey systems to achieve the SDGs.
National statistical offices need support
Survey data are an important tool for policy design, implementation, and evaluation. NSOs in lower-income economies cannot improve household surveys without the engagement and support from policymakers and development partners. Building capacities in a variety of data skills, fostering a culture of experimentation, investing in ICT infrastructure to enable technological innovation, and financing are all important ingredients for countries to successfully implement activities along the above eight technical priorities.
Lastly, the Inter-Secretariat Working Group on Household Surveys is here to help.
Join the Conversation