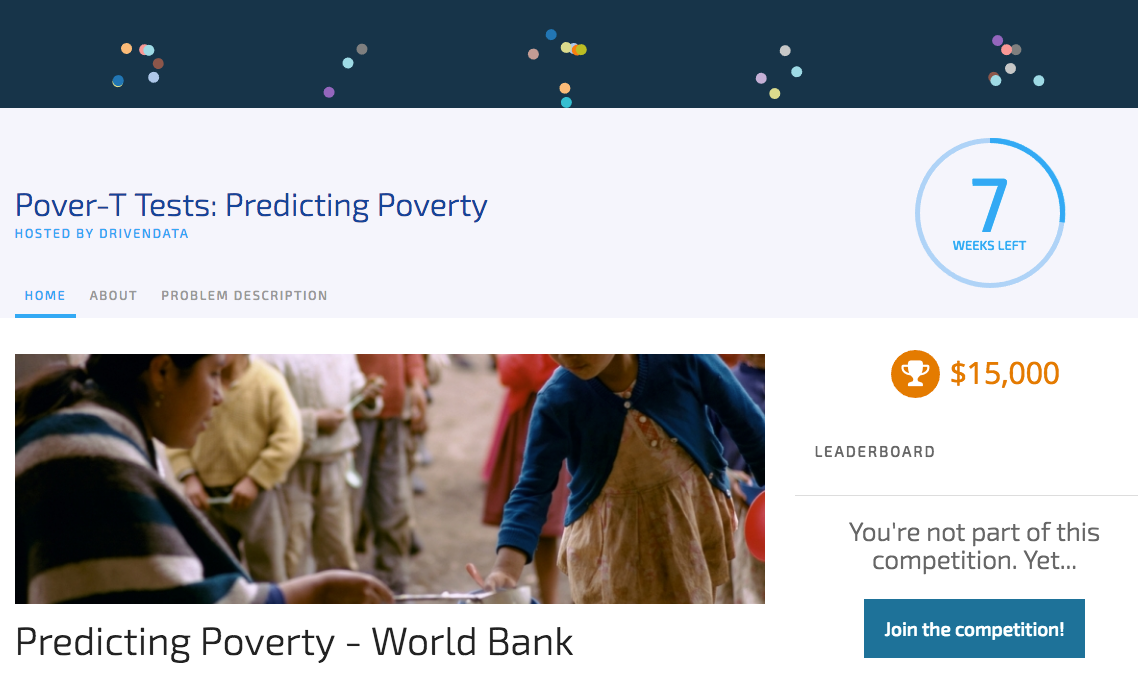
If you want to reduce poverty, you have to be able to identify the poor. But measuring poverty is difficult and expensive, as it requires the collection of detailed data on household consumption or income. We just launched a competition together with data science platform Driven Data, to help us see how well we can predict a household’s poverty status based on easy-to-collect information and using machine learning algorithms.
The competition supplies a set of training data with anonymized qualitative variables from household surveys in 3 countries, including the “poor” or “not poor” classification for each observation.
The challenge is to build models which can accurately classify households from a different set of test data (with the poor/not poor classification removed!) for the same 3 countries, and then submit them for scoring. Performance is measured by the mean log loss for the 3 countries which tells us how accurate the classification models developed are.
Prizes are $6,000; $4,000; and $2,500 for the top 3 performing entries, plus a $2,500 bonus prize for the top-performing entry from a low- or lower-middle income country. The deadline for entries is February 28th 2018.
You can read the full problem description and enter the competition here, and see the Driven Data team’s “benchmark solution” based on a random forest classifier.
Good luck - we look forward to seeing your solutions!
Join the Conversation