The World Bank’s Development Data Group has made substantial progress in the amount and quality of data it provides. The World Development Indicators provides an open collection of over 1,400 high-quality indicators in open formats and API services. Many more indicators and datasets are available in the open data catalog. And tools are now available to access these datasets in R, Python, and STATA. Increasingly and where possible, the Bank also publishes the code for its research and products.
But despite these improvements, many of the systems and processes “behind the curtain” have remained much the same. Large bespoke proprietary systems, many first deployed decades ago, continue to provide critical data management infrastructure, but they don’t necessarily play well with modern tools such as R Studio or python. Important processes such as data validation are often administered manually, and workflow monitored via email. As demand for data grows and diversifies, we are starting to question if the processes designed around early editions of, say, the World Development Indicators or International Debt Statistics (nee Global Development Finance) will continue to sustain us in the years ahead.
Conversations with our international colleagues in the UNSD working group on open data suggested that other statistical organizations are facing similar challenges. And so, with colleagues at Statistics Sierra Leone and the National Administrative Department of Statistics (DANÉ) in Colombia, we started to discuss approaches that might address our collective priorities. At the top of our list: the ability to easily scale to address both anticipated and unforeseen demands; compatibility with today’s data science platforms; and built-in transparency and interoperability. As such, we were most interested in approaches that employ open standards and technologies, but with as few IT requirements as possible. At the same time, we needed solutions that could adapt to (or at least co-exist with) each organization’s existing business processes for an intermediate period.
While we are still considering a range of options, one promising approach is Frictionless Data: an open-source combination of data management specifications and tools that emphasizes simplicity while retaining the power to scale with complexity. We partnered with consultants at Datopian to explore how the Frictionless approach toolkit might work for statistical producers, especially those involved in the Sustainable Development Goals. The resulting research report, supported by the Trust Fund for Statistical Capacity Building, is now available under a Creative Commons license, and we hope it will be especially useful to national statistical offices (NSOs) looking to improve their data management pipelines.
Key Takeaways
While the report documents a range of findings for all three organizations, a few points stood out from the World Bank’s perspective:
- First: although the three organizations in the working group are in very different situations regarding their use of technology, they face remarkably similar challenges around sharing of data and implementation of standards. While it’s often tempting to embrace the “latest and greatest” technology (think cloud computing or machine learning), this finding suggests that “better technology” may not be the best approach. A light-weight, open, technology-independent framework would also be easier for many organizations to adopt and build upon regardless of their existing stack, leading to better data sharing.
- Second: the issues that the research paper speaks to are highly relevant to the Development Data Group’s “farm-to-table” approach to data management, which focuses on the needs of users throughout the data value chain. For this approach to work, users should have clear information about each stage of data production and use. And that requires a framework that can easily document individual datasets and indicators as they pass between organizations, teams, and systems—and aggregate from individual indicators into integrated datasets—without losing precise details about the chain of custody. The Frictionless Data approach seems well suited to this purpose.
- Finally, the extensive interview process during the research phase was especially helpful. It forced us to describe our workflows not only for the benefit of the report’s authors but for ourselves, as shown in the diagram below. It turns out that few if any team members have a current and complete line of sight across the complex data pipelines of key products, which by itself suggests a need for simpler and more transparent approaches.
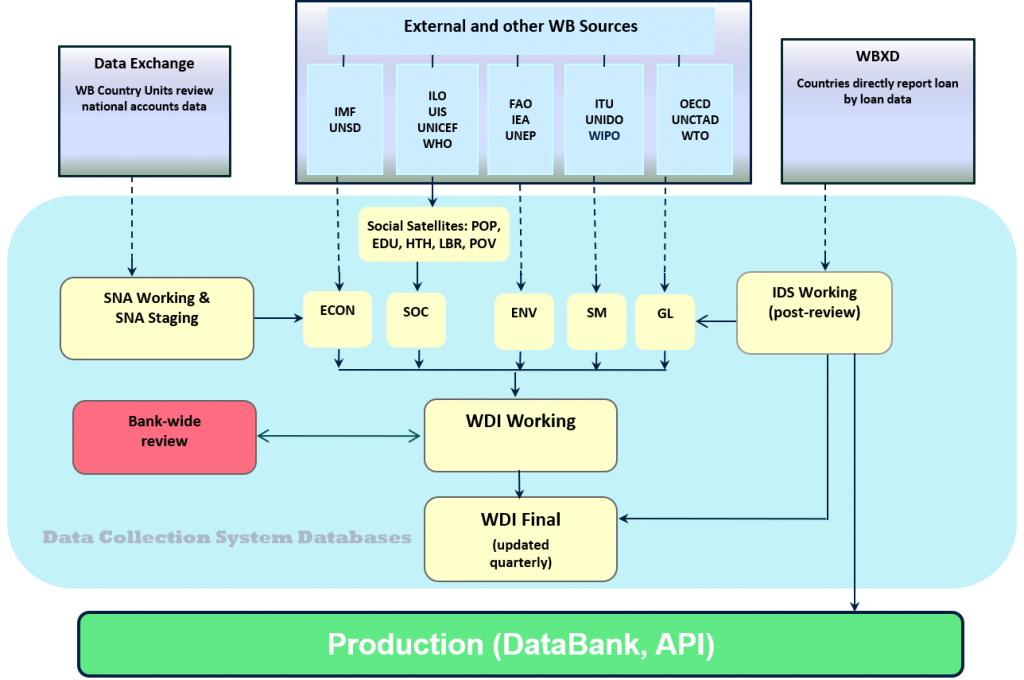
Next Steps
The research paper provides a series of recommendations and suggested next steps for each organization. The Data Group is still discussing how to proceed on these, but we are committed to moving forward. Some early ideas for first steps include:
- Adding “frictionless” practices to upstream components of the World Development Indicators, which already draws from a range of primary data sources, many of which are external.
- Using the frictionless approach to improve and automate data validation, looking more closely at something like goodtables.io (part of the frictionless toolkit) as a possible approach.
- Integrating the frictionless approach into the ongoing learning and capacity building we do for our staff and consultants.
Once we see how the frictionless approach works in the early stages of the WDI, we can look at migrating the approach to other product lines and “downstream” stages, such as the open data API and the data catalog. We are also interested to see how we can continue our collaboration with DANÉ and Statistics SL, as well as with other NSOs and international statistical organizations.
For more information, read the full report discussed in this blog post.
Join the Conversation