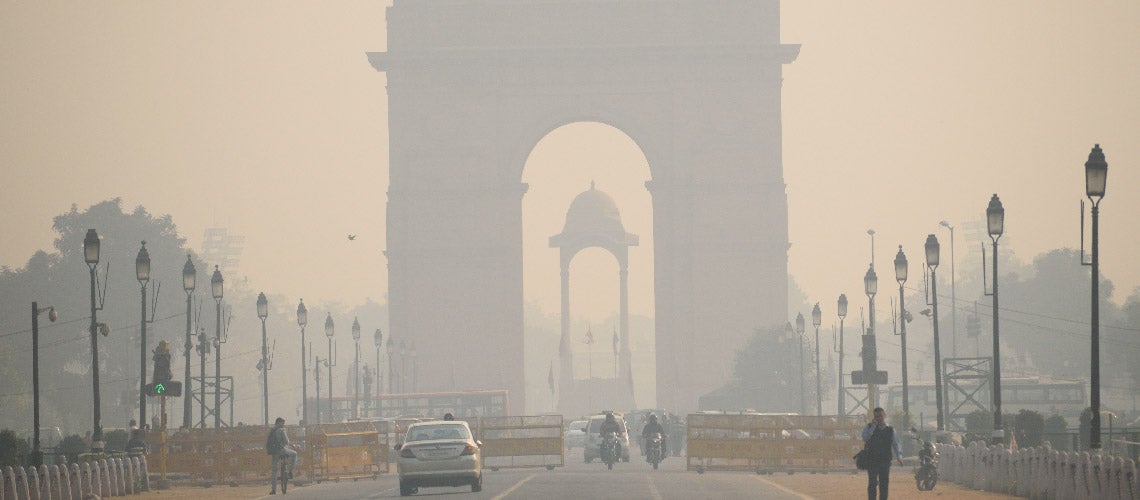
Lockdowns implemented to reduce transmission of the novel coronavirus have brought economic activity to a grinding halt in most parts of the world. Consequently, there is an urgent need for policies that mitigate the impact on overall economic growth and the livelihoods of the poor and vulnerable. Measurement of economic activity at granular spatial and temporal scales is critical in contexts that involve limited budgets and require rapid policy responses.
However, available data that might guide policy responses are sparse on both of these dimensions.
Remote-sensed data has the potential to be a valuable source of information that alleviates these data constraints. Figure 1 below displays emissions before, during, and after the lockdown in China’s Hubei province.
Figure 1: Changes in NO2 Emissions in China’s Hubei Province
While image composites like these are compelling, they can sometimes be misleading.They need to be adjusted for trend, seasonal, and day-of-week effects, while particular care needs to be taken with respect to cloud cover. And to be reliable, the measures need to be averaged over coarser spatial scales than other remote-sensed measures. There are also likely to be various factors driving emissions within a country. For example, in major cities, traffic may be a leading cause; in industrial towns, emissions from factories and mines may be responsible; and in rural areas, emissions are caused by crop burning and forest fires. Lastly, incorporating the influence of wind, rainfall, and temperature will help provide a clearer signal.
Figure 2 shows preliminary estimates that account for most of these caveats for nine major cities across the world. (These estimates do not account for factors related to weather, but we adjust for unmeasured seasonal effects). Across these nine cities, there were large and statistically significant reductions in nitrogen dioxide (NO2) emissions following the lockdowns, ranging from 40% to 70%. In Manila, for example, NO2 emissions fell on average by 65.7%. For most cities analyzed, we do not find a statistically significant drop in emissions for the corresponding days in 2019 (labeled as “placebo lockdown” in Figure 2), which serves to strengthen the credibility and robustness of our estimates of the impacts of the lockdowns in 2020.
Figure 2: Emission Reductions across Major Cities
As with other remote-sensed data, it is important to calibrate the measurements with ground measurements of emissions, as well as to the indicators relevant to the analysis—specifically, in this instance, to reductions in economic activity. We have several reasons to be hopeful. First, our statistical estimates show significant reductions in emissions on Sundays (when businesses are typically closed) in several major cities and strong reductions on Fridays (i.e., the Muslim prayer day) in Tehran, Riyadh, and Cairo. In addition, emissions are consistently elevated in colder seasons across all cities.
Second, as Figure 3 shows below, NO2 emissions are correlated (R2 = 0.46) with night light measures across districts in India. Controlling for district-level features that do not change over time, a sample of 666 districts that covers the entire country confirms the strong positive correlation between the two variables. A 10% change in night lights’ radiance is associated with a 1.6% change in NO2 emissions (an elasticity of 0.16). Third, as Figure 2 shows, the impacts of the lockdowns themselves are large and significant for each of the cities—all of which had imposed strict lockdowns. While this is promising, it is important to note that more work needs to be done to better understand the relationship between NO2 emissions and economic activity in different contexts.
Frequent monitoring and analysis of emissions—both during and after the removal of lockdowns—can provide timely and comparable data, at subnational scales. Further, this data may be complemented with granular sources of information—including data from household surveys, poverty maps, food prices, phone surveys, and social media—to provide a more comprehensive assessment of developments across time and geographies. This will support the implementation and monitoring of the policy responses to the COVID-19 crisis, which can help protect livelihoods and strengthen the economic resilience of the poor and vulnerable.
Figure 3: Relationship between NO2 emissions and night lights’ radiance across Indian districts (2019)
Join the Conversation