High-frequency monitoring of access to food has become especially important during the recent COVID-19 pandemic. Food access in Nigeria, and across the globe, has significantly worsened since the start of the pandemic due to significant disruptions to food supply chains and widespread loss of income. Poor access to food can have both short- and long-term impacts on health and wellbeing and is thus an important targeting criteria. Furthermore, many simple food security indicators have been shown to be extremely sensitive to income and price shocks, so changes in such indicators can be used as rough proxies for changes in monetary poverty and other multidimensional metrics of wellbeing.
Measurement of food access during crises increasingly relies on a consensus of different indicators. However, during the recent pandemic, there is a growing list of surveys that rely on a single experiential indicator of food access—the Food Insecurity Experience Scale (FIES). Part of the reason for the extensive reliance on this indicator is its inclusion as an official measure for tracking progress towards Sustainable Development Goal 2 (zero hunger), and the fact that nearly 100 countries have either incorporated the FIES into their national statistics or are in the process of doing so as of 2019.
Despite the growing popularity of the FIES, the evidence validating experiential indicators has been mixed[1]. Data from the Nigerian Living Standards Survey (NLSS), collected immediately prior to the COVID-19 pandemic[2], afford us the opportunity to compare the FIES to other common food access metrics and monetary poverty in the specific but important case of Nigeria. We demonstrate that the FIES does not appear to correctly identify the food-insecure population in the country.
First, a large share of the population identified by the FIES as being food insecure is not monetarily poor. Figure 1 reports the share of the population that is food insecure by consumption decile. There are roughly equal shares of the population in each consumption decile that are identified by the FIES as having poor food access, which includes the richest income deciles that likely suffer from very little food insecurity.[3] Importantly, the same figure demonstrates that the Food Consumption Score (FCS), which is one of the primary indicators used by WFP to target food assistance, primarily identifies the poorest households as having poor or borderline food access.[4]
Figure 1. Poor or borderline FCS values are much more prevalent in the lower deciles of the monetary consumption distribution, but this is less clear for severe food insecurity as per the FIES
Additionally, spatial variation in the FIES was also not aligned well with poverty and other indicators of food access. Figure 2 demonstrates that food insecurity using the FIES was more prevalent in the south than in the north.[5] However, the same figure demonstrates that monetary poverty and poor food access using the FCS was significantly more prevalent in the north of the country than elsewhere. Importantly, a wide range of other sources corroborate the pattern demonstrated by both monetary poverty and the FCS, where poor food security is far more prevalent in the in the north of Nigeria than elsewhere.[6]
Figure 2. Food insecurity is more prevalent in the south according to the FIES, while the opposite is true for the FCS
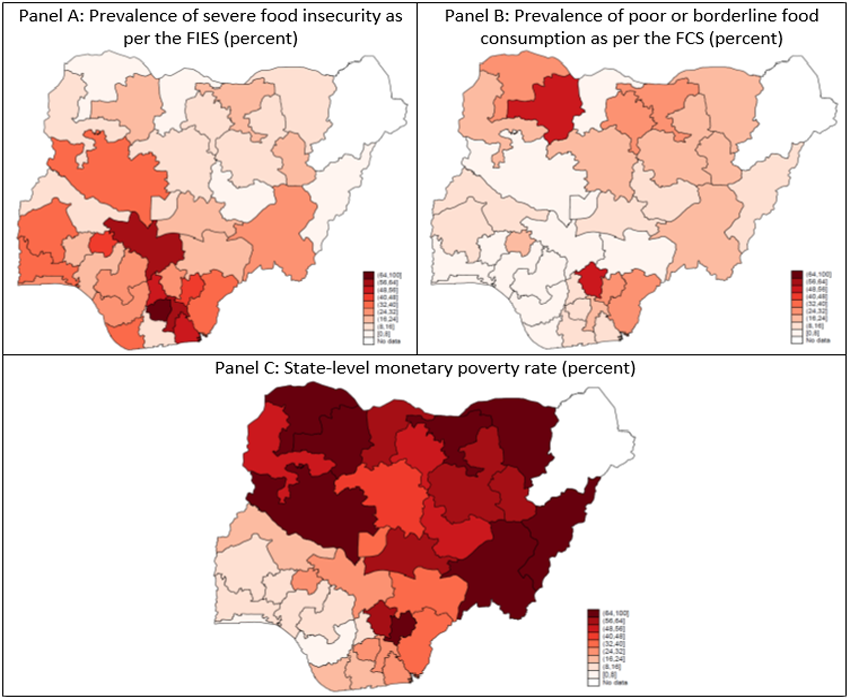
Unfortunately, it is unclear exactly why the FIES is not aligned with other measures of wellbeing and food security. One possibility is that many of the questions on which the FIES is based are subjective, such as whether households worried about food consumption, and might have a tenuous link to actual food consumption. However, Figure 3 demonstrates that the striking north-south differences appeared in all of the FIES’ constituent questions, and not just the more subjective questions that prior research has demonstrated might be difficult to interpret. More investigation is going to be needed to determine precisely why the FIES does not match other indicators.
Figure 3. Food insecurity appears to be more prevalent for all 8 FIES questions in southern Nigeria, when presenting results at the zone level
Despite the inability of the FIES to identify the vulnerable populations in levels, it is possible that the measure can do better at detecting changes in food access. Nigeria’s economy and its people are suffering significant losses through the COVID-19 crisis. The health crisis has been compounded by the sharp drop in the price of oil, on which Nigeria’s economy and public finances heavily depend and recent projections suggest that this dual crisis could leave an additional 10 million Nigerians in poverty by 2022. Consistent with this projection, Figure 5 demonstrates that the FIES is able to detect a worsening of food access in Nigeria in high-frequency data collected throughout the COVID-19 crisis. However, given the discrepancies noted above, it is unclear whether the increase in poor food access is being driven by households that actually have poor food access.
Figure 4. The prevalence of moderate and severe food insecurity as per the FIES has risen in 2020
Given significant discrepancies between the FIES and other indicators of wellbeing in Nigeria, particularly in the regional concentration of poor food access, high-frequency monitoring of food access should consider other metrics as well. In particular, the FCS, and other metrics of dietary diversity, tend to be particularly sensitive to income and price changes and are potentially a better option to measure food access in a crisis. Additionally, information on dietary diversity should be used in conjunction with other indicators from the high-frequency surveys and other types of data to best triangulate the degree to which food access is declining , and the potential causes of the decline. In particular, employment changes in the same high-frequency surveys can further identify regions and households with sudden changes in income, and food price databases can further help to identify regions where food supply chains have been significantly impacted by the pandemic.
This blog was updated on December 1 to further clarify that these findings are based on Nigerian data.
[1] Here are a number of articles collected by FAO that investigate the relationship between the FIES and household characteristics.
[2] The comparison uses data from the Nigerian Living Standards Survey (NLSS), collected between September 2018 and October 2019. The survey includes modules necessary to estimate the FIES, other common food access metrics (e.g., the Food Consumption Score), and monetary poverty.
[3] Households are classed as severely food insecure if their ‘raw score’ – the sum of all 8 questions in the FIES module – is 7 or 8. This is a tenable approach if the data satisfy the basic requirements of the Rasch model, which is the case for the 2018/19 NLSS, where the infit statistics for each of the 8 FIES elements range from 0.85 to 1.15 and the Rasch reliability statistic is 0.77.
[4] Poor or borderline food access corresponds to those with FCS values less than or equal to 42.
[5] These results do not appear to stem solely from using the raw score instead of the Rasch model to calculate the prevalence of food insecurity. When applying the Rasch model separately at the zone level with the RM.weights package in R, severe food insecurity still appears to be more widespread in the South South and South West zones than in the North East and North West zones.
[6] Additional sources include Famine Early Warning System Network’s (FEWS NET) Integrated Phase Classification (IPC) Food Security Projections and the WFP situation reports.
Join the Conversation