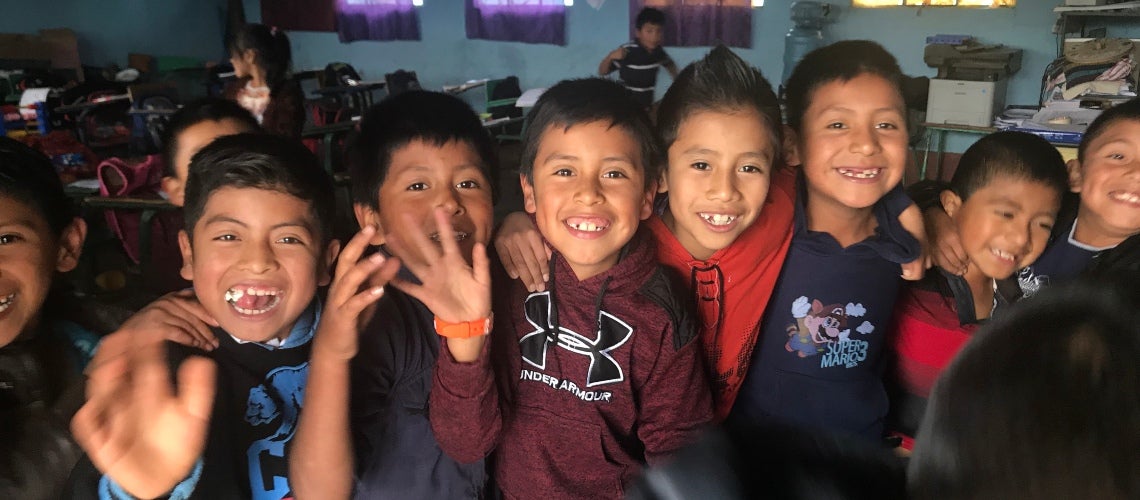
In Guatemala and Honduras, education is legally compulsory through ninth grade, but nearly 40% of sixth graders drop out before getting there. This is the reality for many middle and low-income countries, where educational attainment continues to fall short of aspirations. For young people who drop out prematurely, global evidence suggests that, on average, they will earn less and experience more social and economic challenges than their peers with more years of completed education. School dropout is a complex phenomenon, driven by multiple factors that pull and push youth out of school over time.
To even begin formulating effective dropout prevention strategies, policymakers need to be able to answer a surprisingly difficult question – who is most likely to drop out? This question may appear relatively easy to answer. One might assume dropouts occur only in particularly disadvantaged or dysfunctional schools, or among students with particular characteristics. However, dropouts are often spread across schools and not readily identifiable by single characteristics, reflecting the complexity of the issue. For example, in Guatemala, over half of sixth grade students who drop out in the transition to lower secondary are spread across 70% of the country’s primary schools, and while 50% of students who score in the lowest quartile of a sixth-grade standardized exam drop out, so do 20% of those who score in the highest quartile.
For Guatemala and Honduras, investments made in setting up information management systems over recent years are now yielding returns by answering this dropout prediction problem. In both countries, student and school-level data is now digitalized in networked administrative databases, including unique student identifiers that allow tracking students over time, and, in the case of Guatemala, that can be directly linked to standardized test data. As part of ongoing technical assistance, the World Bank worked with the Ministry of Education of Guatemala and the Secretary of Education of Honduras to make use of their administrative data to estimate prediction models of dropout in the transition from primary to lower secondary school.
These models are based on a growing body of research that mostly utilizes the rich administrative data available in many U.S. school systems to predict who will drop out several months to several years before dropout occurs. Over 30 U.S. states currently have in place some form of “early warning” system based on dropout prediction, ranging from a collection of indicators drawn from administrative data to complex machine learning algorithms.
In a recently published paper, we show that using linear regressions and basic prediction concepts, we are able to accurately predict approximately 80% of the students in the final grade of primary who dropped out within the next year in Guatemala and Honduras, performing at comparable levels to models used in the U.S. (freely accessible working paper version here). Because these prediction models are based on data routinely collected in many information systems and relatively simple analytical techniques, they are feasible to implement in many country contexts. By providing an accurate means of targeting, these models could substantially reduce the misallocation of program resources. In a simple simulation of a modest dropout prevention program, targeting students based on these models rather than targeting poor municipalities or high-dropout schools in Guatemala or Honduras could reduce misallocation of resources by 30 to 80%.
Accurate prediction is only a first step towards building an early warning system, and a natural question immediately arises – what to do with the predictions? Experiences from early warning systems across the U.S. and other countries highlight several important considerations: clearly communicating the meaning of the predictions and avoiding negative labeling of students; defining who should receive what information and who is responsible for taking what actions; empowering local school officials to identify and implement relevant, customized dropout prevention measures; and taking an iterative approach to facilitate learning from initial pilots. As part of our ongoing engagement in Guatemala, the World Bank education team has taken these lessons to heart in supporting the Ministry of Education to develop its own early warning system based on these prediction models.
This approach holds promise for other countries that have invested in building reliable management information systems and that are seeking ways to address their dropout challenges. Given the growth in large administrative datasets, real-time information, and rapid advancements in machine learning, prediction models will become more accurate over time. In addition, an important line of future research would be to use data to not only better predict who drops out but also to better understand why.
Join the Conversation