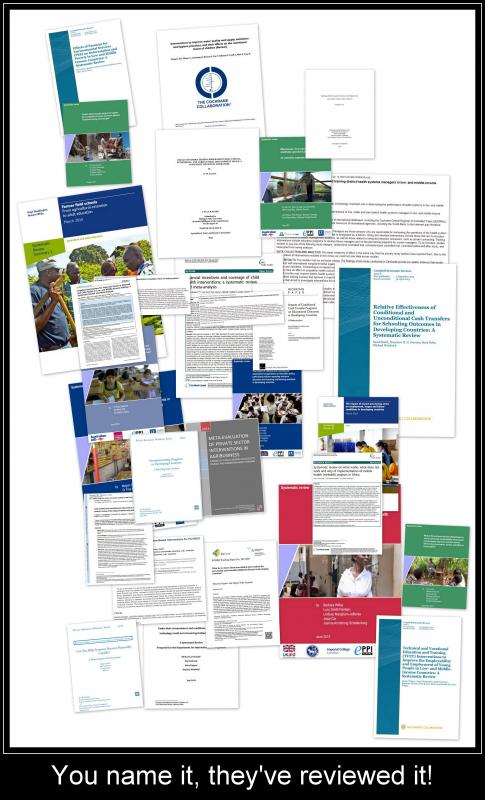
But how definitive are these systematic reviews really? Over the past two years, we noticed that there were multiple systematic reviews on the same topic: How to improve learning outcomes for children in low and middle income countries. In fact, we found six! Of course, these reviews aren’t precisely the same: Some only include randomized-controlled trials (RCTs) and others include quasi-experimental studies. Some examine only how to improve learning outcomes and others include both learning and access outcomes. One only includes studies in Africa. But they all have the common core of seeking to identify what improves learning outcomes.
Here are the six studies:
- Identifying Effective Education Interventions in Sub-Saharan Africa: A Meta-Analysis of Rigorous Impact Evaluations, by Conn (2014)
- School Resources and Educational Outcomes in Developing Countries: A Review of the Literature from 1990-2010, by Glewwe et al. (2014)
- The Challenge of Education and Learning in the Developing World, by Kremer et al. (2013)
- Quality Education for All Children? What Works in Education in Developing Countries, by Krishnaratne et al. (2013)
- Improving Learning in Primary Schools of Developing Countries: A Meta-Analysis of Randomized Experiments, by McEwan (2014)
- Improving Educational Outcomes in Developing Countries: Lessons from Rigorous Evaluations, by Murnane & Ganimian (2014)
What’s going on? In a recent paper, we try to figure it out.
Differing Compositions. Despite having the same topic, these studies don’t study the same papers. In fact, they don’t even come close. Out of 227 total studies that have learning outcomes across the six reviews, only 3 studies are in all six reviews, per the figure below. That may not be surprising since there are differences in the inclusion criteria (RCTs only, Africa only, etc.). Maybe some of those studies aren’t the highest quality. But only 13 studies are even in the majority (4, 5, or 6) of reviews. 159 of the total studies (70 percent!) are only included in one review. 74 of those are RCTs and so are arguably of higher quality and should be included in more reviews. (Of course, there are low-quality RCTs and high-quality non-RCTs. That’s just an example.) The most comprehensive of the reviews covers less than half of the studies.
If we do a more parsimonious analysis, looking only at RCTs with learning outcomes at the primary level between 1990 and 2010 in Sub-Saharan Africa (which is basically the intersection of the inclusion criteria of the six reviews), we find 42 total studies, and the median number included in a given systematic review is 15, about one-third. So there is surprisingly little overlap in the studies that these reviews examine.
What about categorization? The reviews also vary in how they classify the same studies. For example, a program providing merit scholarships to girls in Kenya is classified alternatively as a school fee reduction, a cash transfer, a student incentive, or a performance incentive. Likewise, a program that provided computer-assisted learning in India is alternatively classified as “computers or technology” or “materials.”
What drives the different conclusions? Composition or categorization? We selected one positive recommendation from each review and examined which studies were driving that recommendation. We then counted how many of those studies were included in other reviews. As the figure below shows, the proportion varies enormously, but the median value is 33%: In other words, another review would likely have just one third of the studies driving a major recommendation in a given review. So composition matters a lot. This is why, for example, McEwan finds much bigger results for computers than others do: The other reviews include – on average – just one third of the studies that drive his result.
At the same time, categorization plays a role. One review highlights the provision of materials as one of the best ways to improve test scores. But several of the key studies that those authors call “materials,” other authors categorize as “computers” or “instructional technology.” While those are certainly materials, not all materials are created equal.
The variation is bigger on the inside . Systematic reviews tend to group interventions into categories (like “incentives” or “information provision” or “computers”), but saying that one of these delivers the highest returns on average masks the fact the variation within these groups is often as big or bigger than the variation across groups. When McEwan finds that computer interventions deliver the highest returns on average, it can be easy to forget that the same category of interventions includes a lot of clunkers, as you can see in the forest plot from his paper, below. (We’re looking at you, One Laptop Per Child in Peru or in Uruguay; but not at you, program providing laptops in China. Man, there’s even heterogeneity within intervention sub-categories!) Indeed, out of 11 categories of interventions in McEwan’s paper, 5 have a bigger standard deviation across effect sizes within the category than across effect sizes in the entire review sample. And for another 5, the standard deviation within category is more than half the standard deviation of the full sample. This is an argument for reporting effectiveness at lower levels of aggregation of intervention categories.
Source: McEwan (2014)
What does this tell us? First, it’s worth investing in an exhaustive search. Maybe it’s even worth replicating searches. Second, it may be worthwhile to combine systematic review methodologies, such as meta-analysis (which is very systematic but excludes some studies) and narrative review (which is not very systematic but allows inclusion of lots of studies, as well as examination of the specific elements of an intervention category that make it work, or not work). Third, maintain low aggregation of intervention categories so that the categories can actually be useful.
Finally, and perhaps most importantly, take systematic reviews with a grain of salt. What they recommend very likely has good evidence behind it; but it may not be the best category of intervention, since chances are, a lot of evidence didn’t make it into the review.
Oh, and what are the three winning studies that made it into all six systematic reviews?
- Many Children Left Behind? Textbooks and Test Scores in Kenya, by Kremer, Glewwe, & Moulin (2009)
- Retrospective vs. Prospective Analysis of School Inputs: The Case of Flip Charts in Kenya, by Glewwe, Kremer, Moulin, and Zitzewitz (2004)
- Incentives to Learn, by Kremer, Miguel, & Thornton (2009)
Future work. Can someone please now do a systematic review of our systematic review of the systematic reviews?
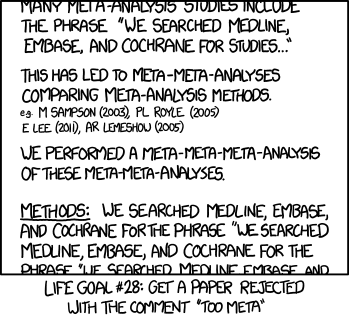
Credit: xkcd
Update (March 3, 2015): This post is part one of a two-part series. The second part is here.
Join the Conversation