It’s amazing to see what technology can do these days! Satellites provide daily images of almost every location on earth, and computers can be trained to process massive amounts of data generated from them to produce insightful analysis/information. This is just one of the demonstrations of artificial intelligence (AI). AI can go beyond just reading images captured from space, it can help improve lives overall.
For urban governance, machine learning and AI are increasingly used to provide near real-time analysis of how cities change in practice – for example, through the conversion of green areas into built-up structures. By teaching computers what to look for in satellite images, rapidly expanding sources of satellite data (public and commercial), together with machine learning algorithms, can be leveraged to quickly reveal how actual city development aligns with planning and zoning or which communities are most prone to flooding. This provides insights beyond the basic satellite snapshots and time-lapse visualizations that can now be readily generated for any areas of interest.
But the barriers to applying these technologies can still seem daunting for many cities around the world. It’s not always clear how exactly to analyze this massive amount of satellite data, nor how to get access to it.
Using machine learning with satellite data to understand changing land use
An ongoing collaboration between the World Bank’s Governance and Land & Geospatial Teams, together with the Development Economics Research Group, is helping connect emerging technology and machine learning to key development stakeholders in Ho Chi Minh City (HCMC), Vietnam. Specific types of machine learning algorithms “teach” computers to automatically detect and classify different types of land cover and land use across space and time, and then generate compelling insights, analytics and visualizations. In this type of machine learning, which is called supervised machine learning, computers are trained what to look for in a satellite image based on reference, or training data. This data consists of examples that can be collected “on the ground” through surveys, or even from existing classifications or from data collected from comparable cities. Based on this data, the machine predicts the spatial distribution of these types of land cover and land use, and this prediction can then be analyzed and translated into smart city management.
While this type of geo-analysis is becoming more pronounced (including in Bank reports), the HCMC initiative combined three features for applied innovation. First, the starting point of the work was to focus on using free imagery and geospatial tools (from NASA, the European Space Agency and Google), rather than going for a “blue chip” or “black box” consultant report. Second, a hands-on review and training was conducted for government officials and students. Finally, the results of this analysis were overlaid with administrative data such as Zoning Boundaries, and linked to HCMC Smart City and Open Data initiatives.
per district (Ho Chi Minh city)
(source: Goldblatt et al., under review)
HCMC’s built-up land has rapidly expanded in the past 20 years. Administratively, HCMC covers just over 2,100 km2, about 6 times the size of Washington, DC. The classification produced by the machine learning approach suggested that, in parallel to a rapid population growth that occurred between 2000 and 2015, the city`s built-up land cover has expanded dramatically, with some of its two dozen districts showing more than 70% increase in built-up land cover. These trends were also expressed in the amount of light emitted at night from the city, which was highly correlated with its economic development.
While “for-free” satellite imagery (i.e. imagery that is made public by governments and other public institutions) is not always the ideal solution for every application, using publicly available satellite data allowed us to demonstrate the potential power of applying machine learning approaches to analyze satellite images, and to illustrate some of the key parameters for successful AI applications with satellite data: obtaining high-resolution satellite imagery, high-quality reference data, and basic off-the-shelf machine learning algorithms. The approach was designed as an entry point for further case studies, including those using commercial high-resolution imagery. "For-free” imagery is available globally and can be used to map countries and regions, but their trade-off is their relatively low spatial resolution, which is not sufficient for some applications that require a much higher granularity.
Various sources of satellite data differ in their spatial (the size of the smallest identifiable object on the ground), spectral (their sensitivity to signals beyond what the eye can see), and temporal (revisit time) resolutions. For example, NASA’s Landsat satellites collect data in a spatial resolution of 30m, while the newer European Space Agencies (ESA) Sentinel satellites collect data in a spatial resolution of 10 meters. While these resolutions are sufficient for land cover mapping, they will not capture, for example, construction sites or zoning violations. These applications require higher-resolution data that is collected by commercial satellites (in a spatial resolution of 15cm- 30cm) or increasingly, by Flycams and Unmanned Flying Vehicles (UAV), which provide data in resolutions down to a couple of centimeters. The best approach would then be to combine all these public and commercial data-sources, by using “for-free” imagery to identify “hot spots” that require a more “zoomed in” bird-eye-view, and target the “for-fee” data to these areas.
In Ho Chi Minh City, mobile apps with geospatial data are used to enforce zoning governance
In late 2017, the city launched a website and smartphone application that allows the public to view the cadastral map and the designated planning/zoning of some districts of the city. The complete version of this project is expected to be launched by the end of 2018. The city’s leadership noted that the objective of this transparency was to improve urban-planning governance, and that the platform would set the groundwork for the city to build more data layers such as construction permit, land information and develop other applications to manage construction, underground space, and buildings on the surface.
At this stage, HCMC zoning information still needs to be assessed manually. However, insights generated by machine learning approaches can be combined and overlaid with zoning data to identify inconsistencies and potential violations. In addition to getting bottom-up reports from inspectors and citizens, planning authorities can also now identify ‘suspicious” zones that have been subject to rapid change as well as inconsistencies with zoning data.
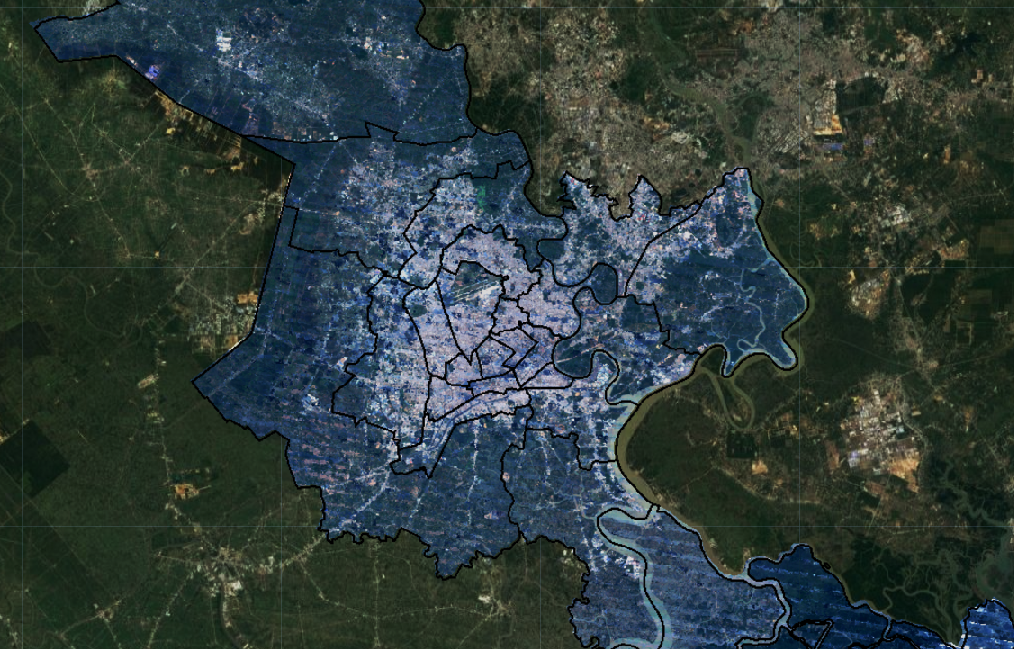
Technology has made it easier to access and process satellite imagery
The traditional model of using satellite imagery was getting a very small subset of it on a Compact Disk and analyzing it on a local computer. But increasingly, this data is brought to the cloud, avoiding the need to download large out of date volumes of satellite data. Cloud based platforms, such as Google Earth Engine, also include machine learning algorithms that can be utilized to analyze millions of scenes in a global scale. This has revolutionized the workflow of satellite data AI.
Obviously, AI will never replace human validation or effective governance on the ground. But satellite data, together with machine learning, can provide a bird’s eye view of what is happening on the ground and what requires further attention. Cities like HCMC can take smart steps to harnessing this technology by first using free data and learning by doing. Sharing and communicating these results with a broader set of stakeholders in and out of government will trigger those solutions that promise to make the biggest difference in people’s lives.
If applications demand it, cities can then acquire access to commercial for-fee data and work to share data and insights among different stakeholders. To ensure value, city leaders will need to articulate the practical questions these new technologies address, and determine which combinations of satellite imagery providers, reference data, and machine learning tools will provide the most corresponding value for money over time. This new wave of technology will allow making smarter decisions thus smarter cities.
Selected Resources References
Big Data Satellite Imagery Reference Link: http://landscape.satsummit.io/ (o pen resource that provides an ov erview of satellites and data products aimed at helping non-experts explore and harness Earth Observation capabilities toward sustainable development)
Big Data UAV Imagery Reference Link: https://uav-development.github.io/ (o pen resource that provides overview of UAV capabilities and examples of UAV applications in development )
Google Earth Engine, https://developers.google.com/earth-engine/edu ( overview of Google Earth Engine, a platform to run ML on Landsat and Sentinel imagery)
Google Earth Engine Timelapse Application: https://earthengine.google.com/timelapse/
( generates Landsat timelapses for any city of interest from 1984 to present)
Goldblatt, R., Deininger, K. and Hanson, G. (under review). Using Publicly Available Satellite Data for Urban Research: Mapping Built-up Land Cover and Land Use in Ho Chi Minh City, Vietnam: A Systematic Comparison between Landsat and Sentinel Satellites. ( elaborates on reference data application for training)
Linked References
Growth Out of the Blue: http://blogs.worldbank.org/endpovertyinsouthasia/measuring-south-asia-s-economy-outer-space.
Ho Chi Minh City launches website, app for land-use planning:
https://tuoitrenews.vn/news/society/20171202/ho-chi-minh-city-launches-website-app-for-landuse-planning/42942.html
HCMC Google Earth Timelapse Link: https://earthengine.google.com/timelapse/#v=10.8231,106.62966,10,latLng&t=3.25
Join the Conversation