Where should telecom providers place their towers and what frequencies should they use?
How can governments best calculate commodity imports to ensure food security?
How can communities better manage areas at risks of floods?
These are just some of the questions that organizations around the world try to answer by using open government data — free, publicly available data that anyone can access and use, without restrictions. Yet around the world, much government data is yet to be made available, and still less in machine-readable [1]formats. In many low and lower-middle income countries, finding and using open data is often challenging. It may take a complicated request process to get data from the government, and the data may come in the form of paper-based documents that are very hard to analyze. A new study looks to better understand how organizations in low and lower-middle income countries utilize machine-readable open data.
In producing the study, the Center for Open Data Enterprise, supported by the World Bank, interviewed dozens of businesses and nonprofit organizations in 20 countries. The organizations were identified through the Open Data Impact Map, a public database of organizations that use open data around the world, and a resource of the Open Data for Development (OD4D) Network. Over 50 use cases were developed as part of this study, each an example of open data use in a low or lower-middle income country.
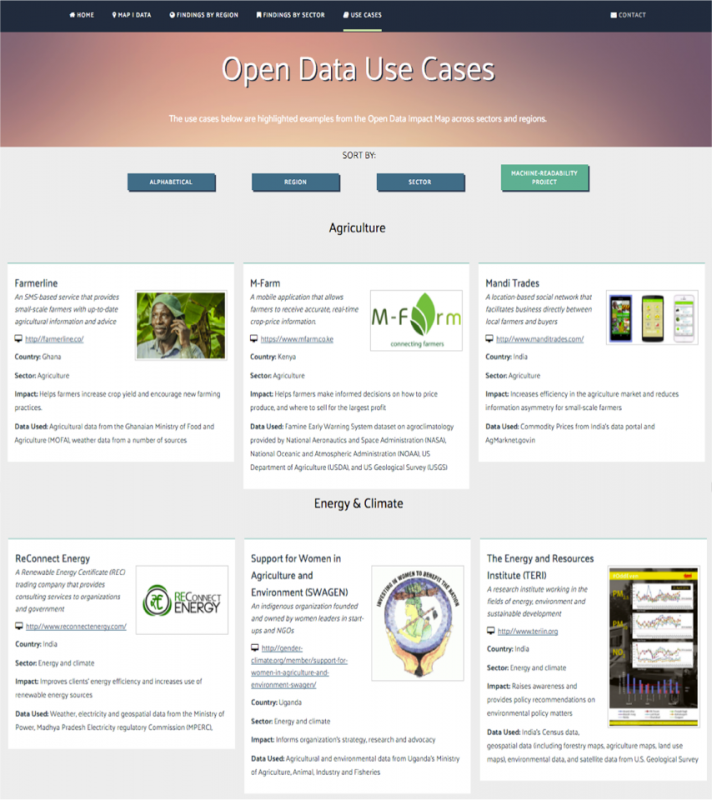
Findings
Organizations use machine-readable data for a number of applications across countries as:
- A resource in the development of web and mobile products and services. Organizations create digital applications that present data in accessible ways. For instance, one agribusiness company in Ghana automates the translation of weather data and commodity prices into simple phrases that are texted to farmers in their local languages. Many organizations conduct predictive analytics and forecasting. For example, one Indian geospatial analytics company uses machine-readable geospatial and agricultural data to predict crop acreage and yields.
- A way to optimize organizational decision-making. Several organizations use machine-readable open data to inform their strategy and investments. Census, household and income surveys in particular are critical to many for targeting populations and markets. It is especially useful when disaggregated by sex, age, location and household income.
- Evidence for research and policy recommendations. Research institutions from Moldova to Zambia use machine-readable data as critical evidence to conduct analyses and support policy recommendations on issues ranging from regional and national economic development, poverty and economic integration, to health and democracy initiatives.
- A tool for advocacy on government spending, elections, and programs. For example, organizations use public. For example, one nonprofit in Ukraine uses spending data to monitor government finances and programs. Another in Nigeria uses budget data to develops infographics for citizens. Yet another provides a tool to monitor contracts, including for the extractive industries in various countries. Across regions, organizations are training journalists to use government data in their reporting, and monitor elections using open electoral commission data.
Most of the data used is not (yet) machine-readable.
While all the organizations in our study used machine-readable data as in their work, half of them told us that the majority of the data they need is still only available in PDFs, images, paper reports, or as website text. Over three quarters of the organizations stated formats were a barrier to data use. This is especially the case when working with large, historic and geospatial datasets. For example, organizations most benefit from geospatial data when it is highly detailed and available in shapefiles, GeoJSON, or CSV - formats that can be utilized by a computer - rather than in image form as it is too often provided. Similarly, census data is especially valuable when it can be accessed in bulk and is available in CSV or other machine-readable formats.
Open source softwares, and the trainings to use them, are critical for working with data that is not machine-readable.
Open source software in particular - tools that are free and have open licenses - are a valuable resource for converting data into more useable formats. Organizations described using a variety of open source or custom-built software to both convert and analyze data. Examples include Tabula for data extraction, postSQL to create a database, and qGIS for geospatial analysis. Many use OpenStreetMap, an openly licensed, crowdsourced global map, to use and share geospatial data they are unable to obtain directly from government sources.
Many spoke also of how valuable they have found trainings on ways to convert information in PDFs or scanned documents into data in machine-readable formats. Several nonprofits, including Data El Salvador, Publish What You Pay and Code for Pakistan conduct regular trainings with journalists, students and other nonprofit organizations to teach them how to convert data using open source tools. However, it is very resource- and time-intensive to convert data into machine-readable formats. The process can lead to data errors, unstructured data is particularly difficult to convert, and time-sensitive data loses value in the time needed for the conversion process.
Organizations often look to networks rather than the source for machine-readable data.
Businesses and nonprofits often look to their professional and personal networks to find information, including machine-readable data. Once one organization has converted the data, they share it through a range of informal channels - from simply emailing files and exchanging data on a USB drive to uploading data on a Github page. This process prevents duplication of efforts, but presents reliability issues, as provenance and licenses for data can get lost as the data is passed on, especially if there is no clear metadata or documentation. Not all organizations are capable of validating data they acquire this way.
Next steps for machine-readable open data
A number of studies, including the Open Data Impact Map, have shown how publicly available government data can be used for a wide variety of applications across sectors. While access to data in any format is undoubtedly valuable, publishing data in formats that cannot be easily analyzed considerably restricts the ability of organizations to make data-driven decisions. Most organizations find that only some of the data they need is available in machine-readable formats, and often need to do substantial work to put the other data they need in a usable form.
For open data to have the greatest value, machine-readability must become a key goal for data providers. Government agencies that release data should move to provide key datasets (such as demographic and geospatial datasets) in machine-readable formats at the source. There is a demand for data in formats that can enable greater, more accurate and efficient analysis that is yet to be met. In the meantime, organizations should continue to raise awareness about the open source tools available (as well as their limitations), and conduct trainings with journalists, nonprofit organizations, businesses, students, and government users themselves. As more governments provide data in more usable formats, the applications of open data will continue to grow.
The Machine-Readability Project, supported by the World Bank, was conducted by the Center for Open Data Enterprise in collaboration with Open Data Watch.
For more information, contact Audrey Ariss at audrey@odenterprise.org
Non-digital material (for example printed or hand-written documents) is by its non-digital nature not machine-readable. But even digital material need not be machine-readable. For example, consider a PDF document containing tables of data. These are definitely digital but are not machine-readable because a computer would struggle to access the tabular information - even though they are very human readable. The equivalent tables in a format such as a spreadsheet would be machine readable.
As another example scans (photographs) of text are not machine-readable (but are human readable!) but the equivalent text in a format such as a simple ASCII text file can machine readable and processable.
Note: The appropriate machine readable format may vary by type of data - so, for example, machine readable formats for geographic data may differ from those for tabular data.
Join the Conversation