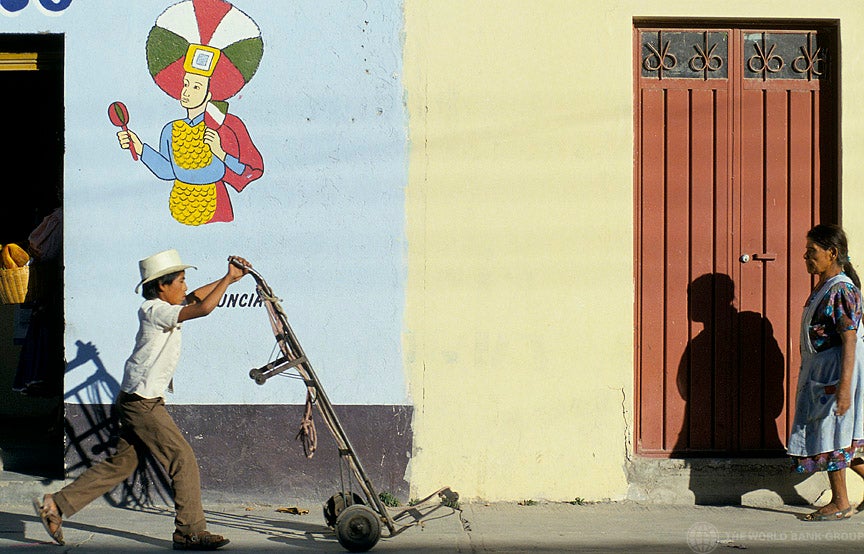
Advancing toward the World Bank’s twin goals of ending extreme poverty and boosting shared prosperity requires knowing who the poor are and where they live. That’s why the World Bank has put considerable effort over the years to improve the collection of survey data and the development of modeling approaches that would allow for accurate measures of poverty. Reaching the most granular levels of geographic aggregation can significantly improve policymaking.
The recent publication of Guidelines to Small Area Estimation for Poverty Mapping caps more than two decades of experience with poverty mapping by the World Bank since the launch of an innovative method combining census and survey data to study the spatial dimensions of poverty. The guidelines build upon the lessons learned from experience and seek to guide readers on the best methods available for a variety of data landscapes. They also provide details on the advantages and disadvantages of these methods, as well as coding examples that users can follow on their own.
The idea behind small area estimation for poverty mapping is relatively simple. With the use of survey data, we can create a model for the relationship between a measure of household welfare—such as per capita household expenditure—and a set of characteristics of the household, its members, and its location. Making use of the estimated model’s parameters, we are able to use the same characteristics in the country’s population census to simulate per capita household expenditures (or incomes) for every single household. From the generated census welfare values, we can calculate any welfare indicator.
The key aspect behind small area estimation is obtaining sufficiently precise estimates of the welfare indicators of interest while minimizing the degree of bias introduced by modeling. By having a granular understanding of the evolution of poverty over time, policymakers can more easily identify lagging areas and pockets of chronic poverty that may require special focus (Fig. 1).
Figure 1: District-level poverty in Vietnam over the years
Source: Vietnam poverty assessment
The seminal methodology introduced in 1998 was later improved to produce less noisy estimates. The evolution was made possible by refinements proposed in 2003, known as the ELL method, and complemented by a stand-alone PovMap software application launched in 2006. These developments provided improvements in precision and facilitated, in subsequent years, the production of small area estimates of poverty indicators in many countries around the world. These early maps fed into crucial policymaking and brought forth the importance of location-based policies, with applications across the world, including in high-income countries.
After ELL, other refinements to the methods have been proposed. One important one was the implementation of Empirical Best (EB) estimates in 2010. The method allowed for considerable improvements over ELL estimates in efficiency, measured in terms of mean squared error (MSE) for each target area.
While Empirical Best estimates are becoming the gold standard for poverty mapping, they are not always feasible, since population census microdata that is suitable to be linked with data from a household survey are not always available. Sometimes census data are available only in aggregated form, or microdata is available but outdated—census data is often collected only every ten years. Such cases require alternative modeling approaches, which are the focus of two chapters of the guidelines. One of them is the area-level method, proposed in 1979, which allows researchers to model poverty rates directly as a function of area-level characteristics.
Despite more than two decades of World Bank work in the area, poverty mapping remains a young research field, with many emerging issues that have not yet been tackled. While we can only speculate on how the field may evolve, much of the recent work focuses on the production of accurate estimates with more frequency. Machine learning (ML) approaches, which often rely on satellite imagery, have become a popular alternative to traditional small area estimation methods. These methodologies are not exactly new, but the quality and amount of data now available are unprecedented. In the wake of the COVID-19 pandemic, governments used the methods to inform their targeting efforts to reach those most in need. Machine learning methods—such as gradient boosting—model poverty rates directly as a function of area-level characteristics, and the guidelines provide evidence that these may be a solid alternative when census microdata are unavailable.
The guidelines seek to provide practitioners with evidence on the pros and cons of the methods that may be applicable in different scenarios. They also bring forth advice on implementation, providing countries with key information to help them support their most vulnerable citizens.
Join the Conversation