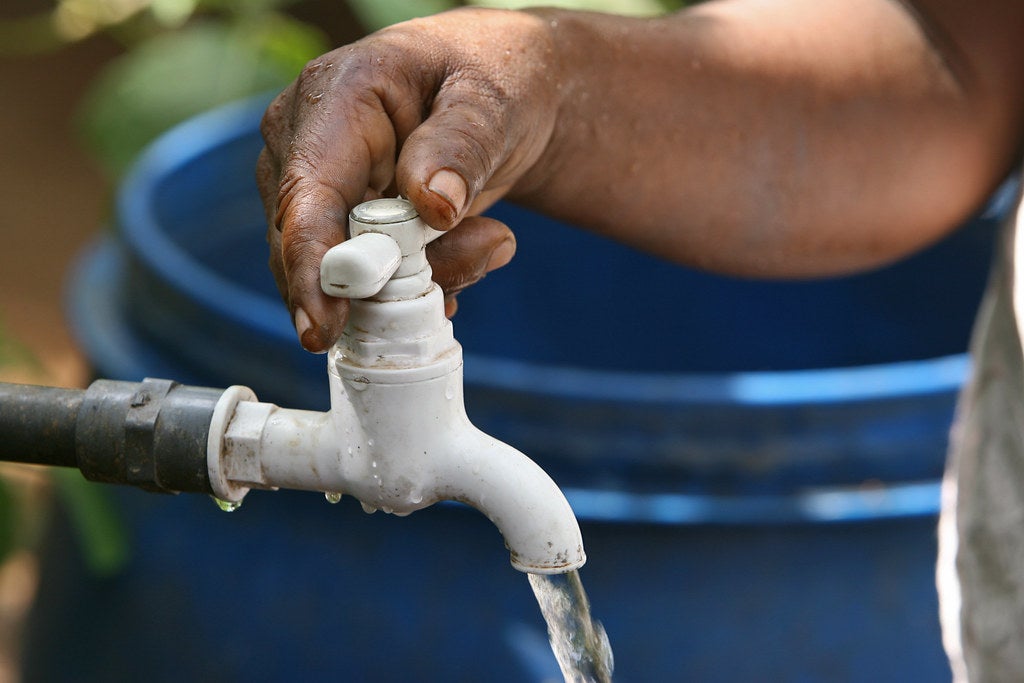
Expanding access to safe drinking water in low and middle-income countries is a key human development priority, with targets set at national and global levels. As such, ensuring access to safe water, sanitation and hygiene for all is also one of the Sustainable Development Goals.
Information on drinking water quality is key to monitoring progress towards achieving global and national targets. Therefore, it is important to accurately measure quality of drinking water accessed by households and individuals and to determine if drinking water is free from biological and chemical contamination.
An increasing number of countries (over 50 to date) have now integrated objective water quality testing in national household surveys to monitor access to safely managed drinking water services. This approach enables the collection of representative information for general household populations, with the potential to disaggregate results by different geographic and socioeconomic groups.
The ability to link water quality information to the wealth of information collected in household surveys facilitates research, as well as the identification of effective interventions to improve access to safely managed drinking water services.
However, integrating water quality testing in household surveys requires additional financial resources and specialized technical assistance, and can increase burden on statistical agencies, especially in resource-constrained contexts. For example, E. coli testing in the field requires equipment, consumables and dedicated training for field staff on aseptic techniques, incubation and interpreting results.
Filling the gaps with data integration and machine learning
In our recent study, “Addressing gaps in data on drinking water quality through data integration and machine learning: evidence from Ethiopia”—a collaborative work between the World Bank Living Standards Measurement Study (LSMS) team and the Joint Monitoring Programme (JMP) of the World Health Organization and UNICEF—we proposed an approach to fill data gaps in drinking water quality.
Let’s unwrap our methodology step by step.
The idea is that while it may not be logistically and financially possible to implement water quality testing in every household survey, data obtained from a recent survey can be integrated with publicly available geospatial data on rainfall, temperature, proximity to the nearest market and roads, among others, and in turn used to train a machine learning model to generate reliable insights on drinking water quality in years when no surveys were conducting tests on the ground.
The country selected as a case study for our research was Ethiopia. In 2016, when the latest data on water quality was collected as part of the Ethiopian Socioeconomic Survey, about 68 percent of households had access to drinking water from improved sources, such as piped sources, protected wells and springs. However, over half of those improved sources were contaminated (Figure 1).
Using water quality testing data from the third wave of the Ethiopia Socioeconomic Survey (ESS3) in 2016, our study, “Addressing gaps in data on drinking water quality through data integration and machine learning: evidence from Ethiopia”, examined the performance of a range of commonly used machine learning algorithms to predict E. coli contamination in the households’ drinking water sources.
The study developed a predictive model for contamination of drinking water sources by integrating socioeconomic survey data with geospatial data sources on the basis of household GPS locations. It compared a few commonly used classification algorithms including GLM, GLMNET, KNN, Support Vector Machine, and two decision tree-based classifiers: Random Forest (RF), and XGBoost. RF performed the best across most metrics, with XGBoost becoming a close runner up.
The study also examined the performance of different groups of predictors variables, namely household demographic and socioeconomic attributes, water service particularities and geospatial variables, on the performance of the algorithms and applied the predictive models to other waves of the ESS, in 2013/14 and 2018/19.
Overall, predictions for ESS3 (2015/16 ESS) were comparable to the actual data under different scenarios. The study finds that a model that has all prospective predictor variables is found to have a strong discrimination ability (Area under the curve (AUC) 0.91; 95% Confidence Interval (CI) 0.89, 0.94). Model performance was poor when type of water source was the only predictor (AUC 0.80; 95% CI 0.77, 0.84).
However, augmenting water source variables with selected household-level socioeconomic predictors, but excluding geospatial variables, resulted in a performance comparable to the full model (AUC 0.89; 95% CI 0.86, 0.91).
The model with only geospatial predictor also achieved a performance that was comparable to the full model (AUC 0.91; 95% CI 0.88, 0.93). The geospatial variables are also key predictors of contamination in the full predictive model (Figure 2).
Figure 2. Variable Importance
Overall, three key take-away messages emerge from our study:
-
Machine learning approaches can be used to develop a model and fill the gap that might arise due to the challenges of implementing a water quality testing.
-
A georeferenced household survey with objective water testing and basic data on socioeconomic attributes, integrated with geospatial data sources, can be used to develop reliable predictive models for drinking water quality.
-
Provided that the data from a recent survey with objective water quality testing exist, predictive machine learning models relying exclusively on geospatial variables may also suffice for understanding variations in risk of E. coli contamination in drinking water sources and generating water quality risk maps.
Join the Conversation