Cities act as escalators out of poverty, as Edward Glaeser demonstrates. And yet, this very function drives poorer immigrants into urban regions – in search of jobs or access to better public services. More than half the world’s population now lives in urban areas , but most social assistance programs in developing countries have traditionally concentrated on the rural poor, with the bulk of past efforts on proper identification and targeting. The pandemic has shone a spotlight on the plight of the urban poor. When the pandemic hit, in the face of harsh lockdowns, the urban poor faced sudden shocks to their income and consumption. Governments in several countries were caught unprepared, with many lacking data on important questions – who are the urban poor? where do they live? how best to find them, and help them?
Finding the poor and those vulnerable to poverty is never an easy exercise. However, in response to the COVID-19 crisis, the World Bank began collecting data on the urban poor, private establishments, monitoring of households, and deployment of vaccines by using several innovative techniques. Data collection in densely populated urban regions, often where informal employment is widespread, and access to public services is an important element of consumption, is especially challenging. Additionally, data requirements can be burdensome when swift and targeted action is vital. Even when recent data exist, insufficient geographical disaggregation can hinder their use (i.e., sampling at the regional or district level) or add constraints on access to the data (i.e., the confidentiality of census data).
Joining forces with machines
Thanks to high-resolution geospatial data, we found an alternative process to map urban vulnerability and poverty within cities. By showing machine images of poor settlements seen from outer space, and then, iteratively, testing its capabilities to reduce the scope for error, we can teach the computer to recognize poverty within cities. In data-scientist terms, this implies designing a supervised machine learning algorithm that is based on the use of training data. Such data consist of samples collected by local experts either through a direct assessment on the ground and/or via analysis of very high-resolution satellite imagery. The algorithm then builds a model by analyzing the “signature” of these samples – a model that then “predicts” the attributes of the remaining datasets based on the classes used in the sample data.
Figure 1 describes this process visually. This involves the collection of data (step 1) consisting of polygons delimitating certain types of urban settlements – residential (poor/informal, middle, or high-income), commercial, or industrial. These are analyzed by the algorithm (step 2) to build a model to predict (step 3) the urban settlement typology for the rest of the city. Of course, different elements of geospatial data can be fed to power the algorithm, including, for instance, polygons of building footprints, characteristics of transport networks, tree cover, public spaces, waste dumpsites, and the like.
Figure 1. Supervised machine learning workflow
Garbage in, garbage out
While the process is straightforward, the availability of high-resolution data for such estimations is vital for these innovative techniques to be deployed for analysis. Data sources such as Digitize Africa and Google Open Buildings have helped revolutionize these approaches. The data allows for high scalability, covering the majority of the African continent, with very precise vector data (polygon of each individual building) spanning both urban and rural areas. The method to analyze the data is tried and tested – the code for the analysis is available to the public at large on Github, having been used extensively by World Bank Group task teams and other institutions. The data allows for high efficiency: the analysis of vector data (polygons) instead of imagery significantly reduces the computing power required to run the analysis, allowing anyone with a standard computer to carry out the process.
Making this work in practice
Geospatial identification of the urban poor (aided by machine learning) can be a crucial input for rapid targeting of resources. In Mozambique, a combination of census and satellite imagery was used to identify (and then subsequently provide resources to) those who were facing economic hardships following COVID-19. Of course, these techniques can also be used to help the semi-urban and rural poor – as illustrated by the Novissi digital platform in Togo. The value of some data for policy-making increases exponentially when combined with other data, such as on hazard risk, or access to public services, or the nature of urban expansion. See the recently published Burundi Urbanization Review report for an example of how multiple facets of vulnerability across neighborhoods were identified. Figure 2 demonstrates the granularity of the information available to local and national policymakers.
Figure 2. Granular and multi-faceted data – Bujumbura, Burundi
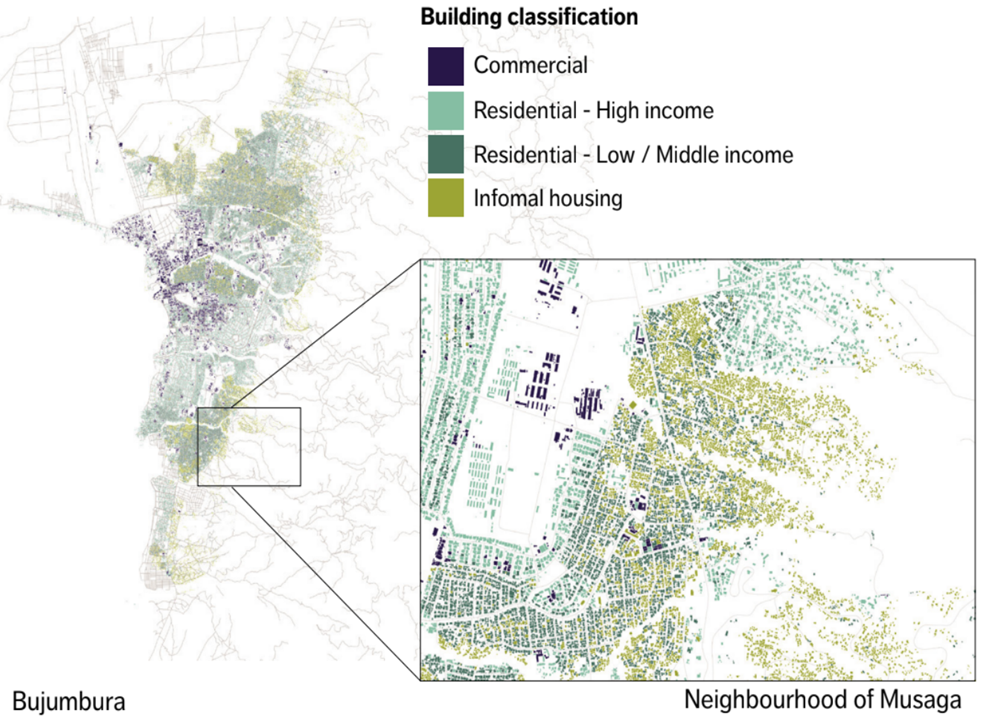
The way forward
With the growing demand for this innovative technology, the World Bank is scaling up this approach in cities and countries in other regions, such as West Africa and the Balkans. We also explore the integration of complementary data, often from open sources, to increase the accuracy of the built-up classification algorithm – always aiming to ensure high scalability. This work will also potentially benefit from the support of the European Space Agency Global Development Assistance (GDA) program, through which international financial institutions can access fit-for-purpose agile earth observation knowledge development. Through this program, ESA provides earth observation products and services, often bespoke, which are developed by European industries to respond to pressing and urgent questions and needs for cities and countries around the world.
There are many challenges that such technologies could be deployed to solve and with each, there comes an opportunity to innovate, scale and solve problems together.
Related links:
- Subscribe to our Sustainable Cities newsletter
- Follow @WBG_Cities on Twitter
Join the Conversation