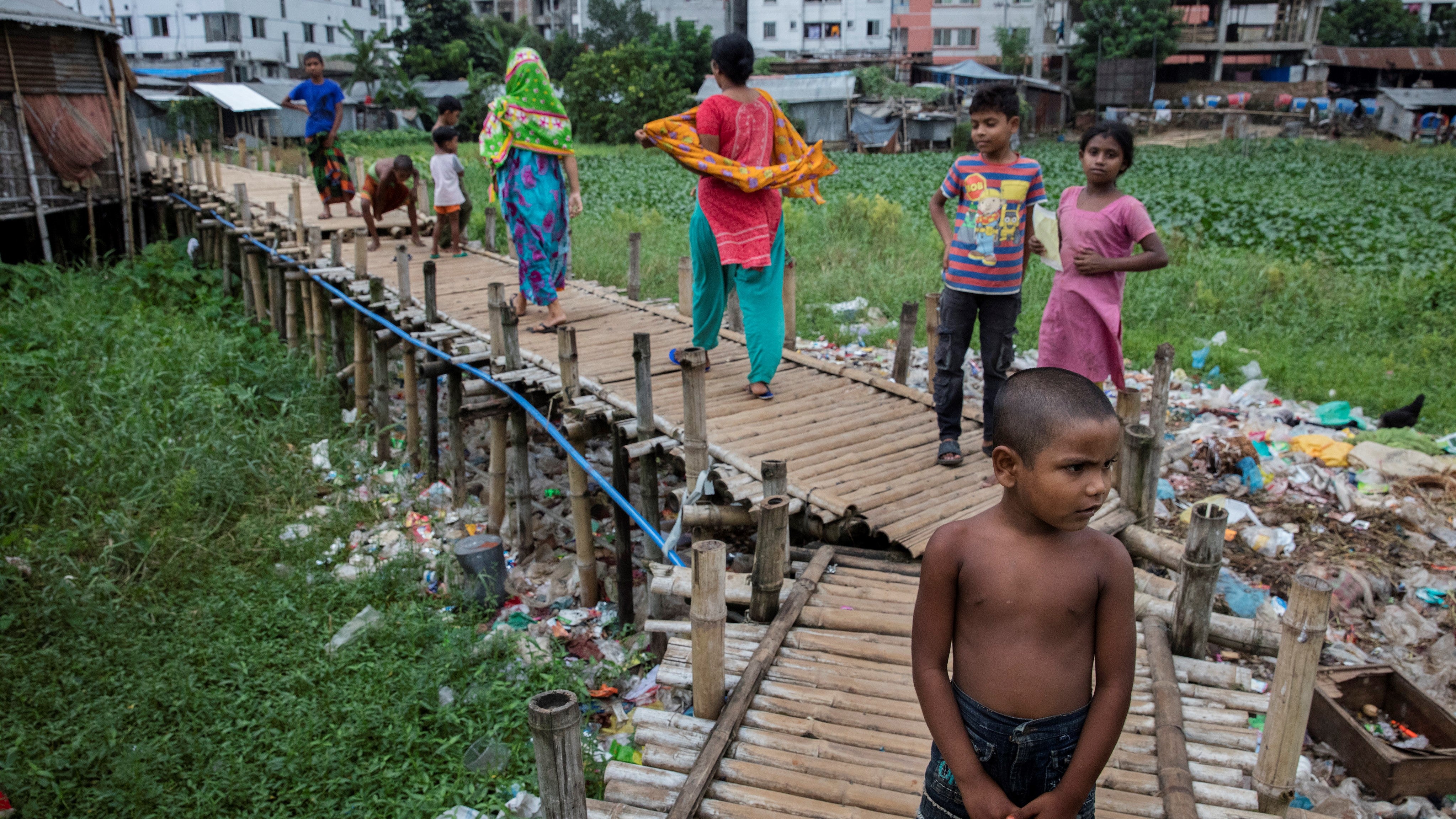
At the time of writing, there are over 9 million confirmed cases of COVID-19 worldwide. Governments are racing to curb its spread, in part by ramping up social distancing policies. Many non-essential businesses are closed, and citizens have been asked (or ordered) to stay home—all with the goal of saving lives and livelihoods.
Lockdown policies like these are central to fighting the novel coronavirus. But policy makers are having a hard time answering key questions about their efficacy. For example, have impacts differed over time and across district boundaries? And crucially, are vulnerable groups less able than others to comply with social distancing regulations?
Data offers some insight. Specifically, consent-based location data generated from smartphone apps. GPS location data has a variety of useful applications, from contact tracing during pandemics to measuring average commute times.
To get a better sense of how social distancing policies are working, we partnered with the technology firm Cuebiq to analyze privacy-protected GPS location data in developing countries. In Jakarta, Indonesia, for example, we analyzed data sourced from over 80 mobile apps and more than 275,000 app users who consented to sharing their location. Here’s what we discovered.
Richer households show greater reduction in mobility
Following Indonesia’s nationwide stay-at-home order on March 15, mobility in Jakarta began to slow significantly. When a state of emergency was declared on March 23, it led to an even sharper decline in activity.
But do less economically advantaged households stay at home for as long as higher-income ones? To find out, we looked at users in the wealthiest 10% of neighborhoods (what we term “high-wealth users”) and the poorest 10% of neighborhoods (known as “low-wealth users”). On average, wealthy or not, users stayed home 2.5 hours longer than usual, increasing from 13 to 15.5 hours per day. However, once we looked at individual wealth categories, a new picture emerged. Low-wealth users only stayed home 11% longer than before the stay-at-home order, whereas high-wealth users did the same for 20% longer.
We also looked at how many neighborhoods these groups visited each day, based on detailed administrative boundaries within the city. Once again, low-wealth users trailed their high-wealth counterparts. Economically advantaged residents reduced the number of neighborhoods they visited by 25%, eight percentage points higher than that observed for lower-income people.
Targeting policies based on coping capacities
These patterns are not unique to Indonesia. Recent analysis suggests the U.S. cities of Seattle, Los Angeles, Detroit, and Chicago saw similarly sharp declines in mobility in the first half of March, with wealthier households staying home sooner than their lower-income counterparts. Researchers comparing U.S. counties arrived at similar conclusions.
The findings for U.S. and Indonesian cities underscore a simple fact: lockdown policies affect citizens differently. Vulnerable groups find it more challenging to comply with social distancing measures for a wide variety of reasons, including limited household savings, a weak or nonexistent social safety net, incomes that depend on face-to-face contact, crowded living arrangements, and poor access to basic services. Many of the most vulnerable are left with the terrible choice of staying home and forgoing an income, or going to work but doing so at potentially great physical risk.
Smartphone location data, with the right privacy safeguards, allows us to derive actionable insights to inform COVID-19 responses to better protect urban dwellers against risk. It can help us more effectively target information campaigns, provision of services, and safety improvements for public transportation, among other things. For example, by identifying bus routes with higher passenger numbers, location data can help decision makers direct resources to routes that need more support in keeping passengers safely distanced. Additionally, and perhaps above all, location data can help policy makers determine how, when, and where to lift lockdown measures.
By understanding differentiated policy impacts across groups and areas within a city, this type of analysis can open the door to broader conversations about complementary measures needed to protect the health and livelihoods of citizens, particularly the most vulnerable.
Jakarta is just one example, and our team is conducting similar analyses for other areas of the world. Stay tuned!
Join the Conversation