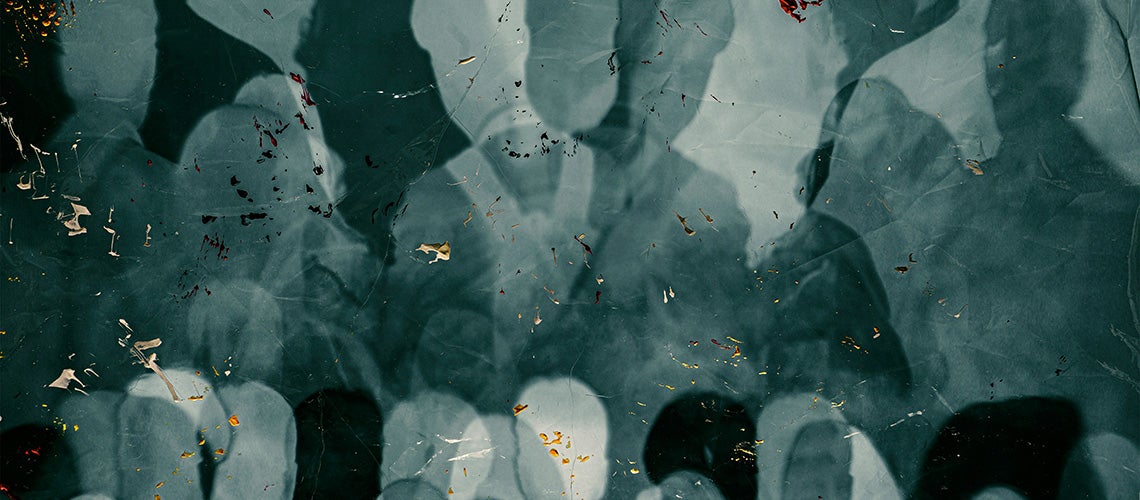
The use of algorithms is increasingly common, from the media content we are presented online to our creditworthiness. However, too often, these algorithms not only replicate, but actually amplify bias, with discriminatory consequences. While commercial incentives have driven an explosion in the private sector’s use of machine learning (ML), governments have been slower to explore how it can mitigate inequality of outcomes between social groups.
A recently developed Cost-Benefit Analysis (CBA) tool informs justice intervention selection through comprehensive data collection, resource allocation, and measurement of societal benefits, particularly for marginalized groups. There is exciting and increasing potential for this approach to enhance policy decision-making, social sustainability, and public participation in policy processes.
An AI-driven cost-benefit analysis tool
Incorporating both direct and indirect economic costs and benefits includes accounting for the economic impact of violence, deterrence, and dynamics of trust in law enforcement. CBA commonly compares a policy or program’s average benefits with associated average costs. However, these approaches may conceal important information about which social groups benefit the most net of costs.
The current version of the CBA tool accounts for inter-social group inequality in developing effective and sustainable policies. The tool was applied to legal aid justice policy, requiring comprehensive measurement of justice process impact. This included:
- Moving beyond the traditional cost-benefit conceptual framework of utilizing averages.
- Identifying social group or population-specific variations (e.g., individual actor attributes, political structures, institutions).
- Identifying how justice processes differ across groups or populations.
- Distributing costs and benefits according to the identified variations.
- Using empirically informed statistical techniques to gain new insights from data and maximize the impact for beneficiaries.
The tool allows for cost-feasibility analysis (comparing the costs of the project against the budget), cost-savings analysis (comparing the costs of the project against the savings generated from avoided crimes), cost-effectiveness analysis (comparing the costs of the project against the number of units of output, such as the number of crimes prevented) and CBA (comparing the costs of the project against the overall benefits––avoided crimes and other benefits such as enhanced safety). The tool also overcomes existing CBA shortcomings by including components that account for variation.
Figure 1: Visualization of the Cost-Benefit Analysis Tool
As shown in the figure above, the available version of the tool (comprising Modules 1 through 3) handles several macro variables such as inflation, allows for the analysis of multiple contexts, and accounts for data biases. Importantly, it enables the heterogeneity of costs and benefits to be captured and the best approach for group-specific outcomes to be identified across different contexts and justice process policy types. The proposed future development of the tool (Modules 4 through 6) will interact to enhance, through ML, user support (data imputation, anomaly and correlation detection), and improve CBA variable and value predictions through feature engineering and feature selection processes.
Potential for development policy and operations
AI-driven accounting for heterogeneity allows international development assistance, net of costs, to better target poverty. The joint United Nations–World Bank report Pathways for Peace highlights the risk of violence associated with social group-specific exclusion from access to justice and security. The World Bank has developed tools for analyzing governance and justice reform in alignment with poverty reduction and shared prosperity goals.
The potential of AI-driven CBA to enhance policy and programmatic analysis alongside more traditional development impact evaluations or poverty and social impact analyses is significant. AI-driven CBA overcomes the limitations of average benefits (specifically, average treatment effects) employed by traditional CBA by employing a more comprehensive economic framework that accounts for context, variance in policy effect across individuals and communities, and AI-enabled empirical insights.
Policy change informing equitable inter-social group outcomes, particularly where the evidence of equity can be quantified and communicated, can enhance not only outcomes but the legitimacy of the process that achieved them. These elements of social sustainability, delivered via objectively equitable policies, interventions and outcomes produced by ML-driven CBA, significantly advance the World Bank’s twin goals of ending extreme poverty and boosting shared prosperity on a livable planet.
Join the Conversation