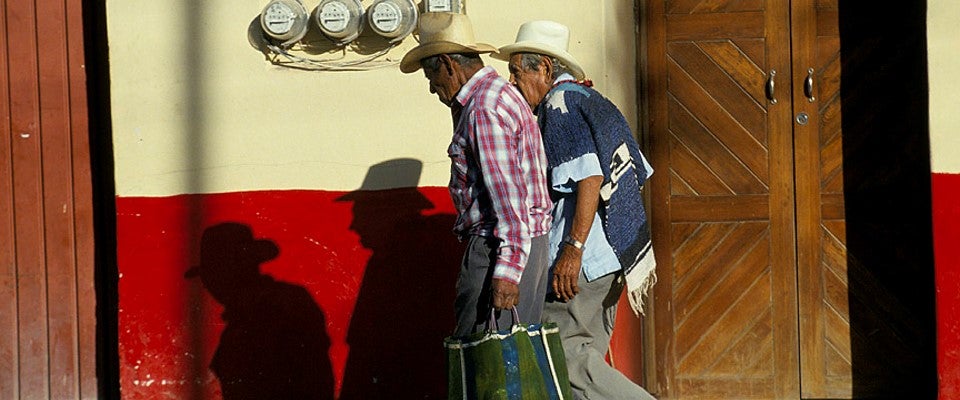
Data is expensive to collect and maintain, and as emphasized in the 2021 WDR, we could better use the limited data we have. So, what is to be done and how?
Small area estimation (SAE) is an old technique that the World Bank helped popularize 25 years ago to generate more granular estimates from data. These traditional techniques are rapidly being updated due to the availability of new forms and sources of data and improved computing power. One important frontier of this revolution is data integration, which combines multiple sources of data to obtain more useful measures than one gets from a survey alone. Harnessing data integration should broadly improve the Bank’s analytics and, by extension, help promote sustainable development.
Traditionally, small area estimation has been conducted by combining survey and census data. But we now have plenty of data, including the widespread free availability of predictive geospatial indicators that can potentially be used instead of census data. Current census data is still the best choice, but censuses are often old and occasionally ancient. Could geospatial data at the village level be the second-best option when the most recent census is old? If so, data integration could potentially generate more accurate and precise estimates for any indicator from any survey that can be linked with geospatial data .
Does Integrating Geospatial Data Improve Estimates?
Our newly released working paper shows that integrating survey and geospatial data significantly improves on survey estimates, in terms of precision and accuracy, of monetary poverty rates in Mexican municipalities. This is a follow-up to earlier work that shows similarly encouraging results for non-monetary poverty in Sri Lanka and Tanzania and female labor force participation in urban Mexico. In 2015, Mexico conducted a large inter census with 5.8 million households, which the national statistics office combined with the 2014 survey to produce official municipal poverty estimates. This provides an excellent benchmark measure of “truth” to try to match by combining the same 2014 household survey with geospatial data. In this case, integrating survey and census data raised the correlation between the sample and the inter census benchmark from 0.8 to 0.86 in sampled municipalities. This is a more significant increase than it may seem, as each correlation point is important when finding the poorest areas. More precise estimates come with increased accuracy, roughly equivalent to increasing the survey size by about a factor of about 2.4. Since surveys routinely cost at least a million dollars to field, and predictive geospatial indicators are freely available, applying small area estimation techniques routinely has the potential to add hundreds of millions of dollars of value.
While the geospatial estimates do well in the Mexican case, estimates from the 2010 census are even more accurate, with a correlation of 0.9, indicating that it would have been better in this case to stick with five-year-old census estimates than update them with current geospatial indicators. Current geospatial estimates may be better than five-year-old census estimates in contexts where spatial patterns of poverty are changing faster. More research is needed to understand it better.
Methodologies are rapidly evolving, but for now, we feel most comfortable with a model that predicts household per capita income based on geospatial variables matched at the village level. The resulting model is then used to simulate predictions of welfare and poverty using geospatial data for the whole country, which helps fill in the spatial gaps in the sample. A key feature of this model, called the empirical best predictor (EBP) model, is that it uses the survey data as a prior estimate that is updated, in a Bayesian sense, using model predictions.
Should we continue to use linear models or move to more sophisticated tree-based machine learning methods?
These newer methods generate more accurate predictions by better accounting for non-linearities and interactions in predictions, and we expect they will become increasingly common. But for now, tree-based methods are still difficult to understand and explain to non-data scientists. In addition, many new methods lack an established method for estimating uncertainty, which is critically important to know how confident we should be in the estimates. In contrast, the linear EBP model benefits from its roots in linear regression and a well-developed statistics literature that agrees on how to estimate uncertainty. In addition, the linear models value parsimony more than tree-based methods, making them easier to understand and explain.
Which welfare measure to use to target poor villages?
A recent well-known paper used data on assets from 59 countries to predict wealth in 159 countries, which was then used to target emergency cash transfers in Togo. While this is exciting and innovative work, there are key differences between wealth and official measure of poverty based on consumption or income that are not always fully appreciated. Wealth is not always a great proxy for official poverty measures, especially in rural areas and among the poorest. In the Mexican data, the correlation between the asset index with the official poverty measure is only 0.6 across sampled municipalities. This is much worse than the 0.8 correlation when just using the survey data – even though the survey is not considered representative at the municipal level -- let alone the 0.86 obtained when adding geospatial indicators in a linear EBP model, and the 0.9 when using the 2010 census estimates. This isn’t a knock on the wealth estimates, which after all never intended to estimate income or consumption-based poverty. The wealth measures do have the advantage of being trained on more data and therefore use more sophisticated machine learning methods. But as long as the Bank continues to treat income and consumption as the official measure of monetary poverty, it’s better to target interventions based on simpler predictions of these official measures rather than fancier predictions of wealth.
Should the model predicting income or consumption be specified at the household or target area?
Both can work well, and there is currently no consensus in the literature on which works better. However, a recently published paper concludes that the household model is biased, and recommends using area-level models if possible. This concern that household models containing village-level predictors are biased seems inconsistent with current practice. It is very common for researchers to include village, county, or state level averages as independent variables in regressions (two of the countless published examples are here and here). It’s also common to include area-level averages in small area estimation models, which can improve the accuracy of both the predictions and the estimated confidence intervals. But, the paper also shows that models relating household consumption to village characteristics give biased predictions, after a particular sample is selected, due to a mismatch in the means between the sample and the population.
Our new paper finds that this same mismatch is present in area-level models and leads to the same bias, and furthermore is negligible in most practical cases. In addition, if the bias is considered prior to drawing the sample (a more appropriate method), then it disappears in all methods. This is consistent with our empirical results, which show that the household level model generates slightly more accurate predictions than the area-level model in the Mexican context, because it can incorporate geospatial data at the village and municipal level. The household-level model has the advantage of using more spatially disaggregated data, and there is no reason to believe that household-level models suffer from any more bias than area-level models.
There’s a lot more work to do in this area, to experiment with different statistical methods for predicting levels and confidence intervals, different indicators derived from satellites and other non-traditional data, and tools to make data integration easier for clients and the general public. But with every new published paper on this topic, it’s becoming clearer that techniques to integrate different types of data can be usefully applied now to help us better understand the socio-economic landscape of the world around us .
Join the Conversation