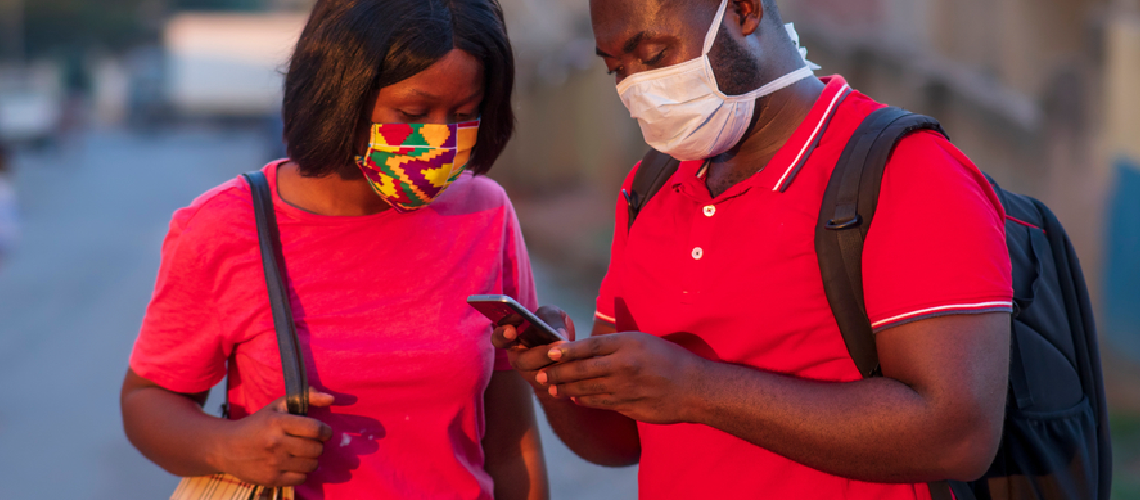
In an effort to stem the unprecedented spread of the deadly COVID-19 virus, many Sub-Saharan African countries locked down at the start of the epidemic along with governments around the world. Amid a spike in cases now, new lockdown measures have been imposed in many countries. While such measures helped to substantially slow the spread, we need to think about how lockdowns can be better planned to ensure that they limit damage to households and economies. This blog shows one way, examining mobile phone data and using Agent Based Modeling to inform a ‘smarter’ response.
The context
In March 2020, as cases started to appear in Sub-Saharan Africa, the World Bank team collaborated with a local Mobile Network Operator (MNO) in one country with the goal of producing useful analytics for COVID-19 policies. The MNO aggregated their mobile phone data (Call Detail Records) into indicators using one of the models for privacy-conscientious use of mobile phone data where individual level data is not shared. Instead, data is provided at the administrative unit level and small aggregated values are masked. These indicators showed how mobility patterns have been changing in response to government policies around the lockdown.
How can mobile phone data help?
On an average day, people may leave their homes to go to work, school, or shop. Such journeys entail traveling within the neighborhood, crossing district borders, or even traveling across the country. As people travel, those that are sick could infect others. Understanding mobility can provide insights into how a disease might spread. Cell phone data can be used to measure this mobility based on changes in the towers from which calls are made.
As part of the broader work of the World Bank COVID-19 Mobility Analytics Task Force, the team worked to develop open source code that (1) produces the set of mobility indicators; (2) cleans the indicators to ensure high quality; and (3) visualizes them on a dashboard. We calculate a baseline of mobility prior to the lockdown, based on the typical movement in February and early March 2020, and measure how mitigation measures affect this mobility.
Mobile phone data shows impact of nationwide lockdown on population movement
The first thing we learned from analysis of the mobile phone data is that the imposition of a nationwide lockdown led to large changes in mobility across the country.
Figure 1: Percentage Change in Number of Trips Between Wards
There is an initial jump in movement after the announcement of the lockdown, followed by a drastic decrease of 40% once the lockdown was imposed (Figure 2). The initial jump reflects patterns that were reported around the world, where subsistence workers in urban centers, fearful of their income sources dwindling, travelled back in large numbers to their hometowns and villages in rural districts.
Mobility changes differ greatly from one district to another
However, there is tremendous geographic heterogeneity. The largest decrease of over 80% is seen in a district with a busy land border crossing. Other districts experiencing the greatest decline in mobility were urban centers, where we saw a reduction of about 65%. Rural areas saw much smaller changes in mobility compared to urban areas, as measured by the cell phone data (Figure 4 compares an urban district containing the capital with a rural district).
Knowledge on mobility is critical for modeling and can inform the government’s policy response
Information on mobility dynamics can feed into decision making around how to control the spread of the virus. We developed an Agent Based Model (ABM) which simulates how the virus could spread across and between districts using census and DHS data to characterize individuals. ABM is a computational approach that focuses on modelling dynamic interactions between individuals and their environment, which helps to capture the heterogeneity in risk based on many different factors. Detailed mobility data is essential to inform the model simulation of how those populations move and to where.
The ABM produces likely trajectories of how the virus could spread under different scenarios and policies, and how respective levels of movement restrictions could limit the spread of the virus (Figure 6). By combining data on mobility, demographics and risk, the model tells us which districts could potentially display the highest risk of transmission, and how this might change depending on the policies implemented. To produce these analyses, the World Bank team worked in conjunction with the national government’s COVID-19 Research Group and Imperial College London.
These analyses can help to inform the government’s approach to easing lockdown, in particular, around a more localized approach where possible. Given the large economic costs of a nationwide lockdown, these data provide useful insights into how restrictions could be lifted carefully in a spatially disaggregated way that could help reduce the economic costs while still maintaining measures in the highest risk areas. This is only one strategy in the arsenal needed to effectively fight the COVID-19 pandemic.
Future work with Mobility Data
We are further refining the work, building on broader consultations across sector Ministries and strengthening collaboration with the inclusion of a second telecom provider. Once the data and models are in place, it becomes possible to apply them in new ways as the situation changes, such as to now consider vaccine prioritization.
One of the most powerful aspects of this type of research is that with the right data, it can be replicated in other contexts. This work is part of a larger initiative under the World Bank COVID-19 Mobility Analytics Task Force, which is working with telecom partners to scale use of aggregated mobility analytics and modeling in Sub-Saharan African countries. The indicators produced by the task force were already used by a team in The Gambia to study the impacts of restrictions on mobility, and they are also being applied in other countries. We are still far from the end of this crisis, and we must leverage all available data in an ethical and responsible way, especially in contexts with limited resources, to support efforts to protect peoples’ health as well as the long period of economic recovery ahead.
The research has been funded with UK aid from the UK government through the ieConnect for Impact program; with support from the Trust Fund for Statistical Capacity Building III (TFSCB-III), which is funded by the United Kingdom’s Foreign, Commonwealth & Development Office, the Department of Foreign Affairs and Trade of Ireland, and the Governments of Canada and Korea; as well as support from the Research Support Budget in the Development Economics Vice-Presidency. We thank Mukami Kariuki and her team for support with this project and Yi Rong Hoo, Leonardo Viotti, Robert Marty, Sebastian Wolf and Andrea Quevedo for research assistance.
Join the Conversation