In a recent working paper, we use receipt data from Rwanda to show that increasing domestic mask supply was a cost-effective way to slow the spread of COVID-19. Export restrictions at the onset of the pandemic meant countries with low domestic mask production faced stark supply constraints. Shortly after the first case in Rwanda The Government of Rwanda gave licenses and a value-added tax exemption to some garment manufacturers to produce certifiably high-quality masks . As fiscal spending accelerated during the pandemic, evaluating the impact of such tax holidays became particularly important for governments. As part of our ongoing research partnership with the tax authority, we mobilized tax administration data to shed light on the impacts of this policy.
Couple of things to note about the context of this study is that 1/ Rwanda enforced a mask mandate relatively early in the pandemic (April 2020), and 2/ subsequently, compliance was close to universal. We return to this as we interpret our results.
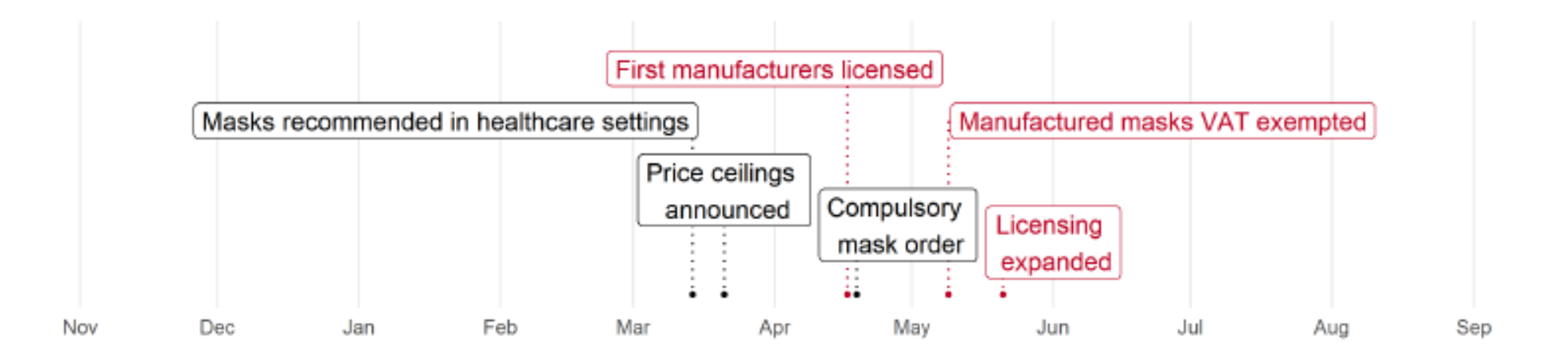
A little more exposure.
As a convenient excuse for not working on this paper (among other papers and many more excuses!), we blogged about exposure designs. We combine this estimation strategy with difference-in-differences in our context using textile (non-mask) trade networks to predict exposure to domestically produced masks. The idea is simple: if firms source garments from mask manufacturing sub-Districts they are also more likely to source masks from manufacturers than firms who have no trade connection to mask manufacturing sub-Districts. This is precisely what we observe — mask exposure through garment sourcing strongly predicts purchases of high-quality manufactured masks. In Figure 2, we can see the variation in mask exposure across sub-Districts!
Okay, so we use garment trade as a source of as-good-as-random variation in exposure to mask manufacturing; is the implicit assumption of exogeneity reasonable? We assess the robustness of our exogeneity assumption in three ways. First, we check for confounders and verify that garment sourcing does not predict sub-District characteristics, and re-run our analysis including these controls. Second, we show that garment sourcing does not predict sub-District trends in outcomes. Third, we use an alternative measure of regions’ mask manufacturing that controls for regional characteristics; this “doubly robust” analysis (adjusting our measures of both mask manufacturing and garment sourcing using controls) does not affect our results. Finally, we note that our design does not involved any staggering (pppphewwww) so we get a pass on that (past blogs discuss when we don’t). However, we acknowledge that testing for parallel trend is insufficient for DID with a continuous treatment and additionally show that sub-Districts’ characteristics predict neither mask manufacturing intensity nor mask manufacturing exposure. With this in hand, we are ready to roll onto estimation and results!
Estimation
Our estimation procedure is simple: we regress our three outcomes of interest (log buyer prices of masks, mask quantities purchased from manufacturers as a share of total mask quantities purchased, and COVID-19 infections) on our measure of exposure to mask manufacturing, controlling for sub-District fixed effects and month fixed effects. We implement an event study design, which generalizes difference-in-differences — we interact our measure of exposure to mask manufacturing with month fixed effects, allowing us to estimate the monthly impacts of mask manufacturing relative to the months prior to its introduction.
Quantity goes up, Prices fall!
We find pretty large, persistent effects of exposure to mask manufacturing on prices (black time series, Figure 3): scaling our estimates at the national level implies an 8.8% fall in mask prices as a result of licensing. This result is aligned with simpler descriptive observations on the price time series (it represents half of the aggregate fall in mask prices following the introduction of mask manufacturing), which brought us comfort our exposure design did not just spit out some magic results! Finally, we note that these price differences persist; in the paper, we interpret these differences as driven by persistent difference in trade costs between sub-Districts, and we provide back-of-envelope calculations using estimates from gravity regressions that support this notion.
While we also find large increases in mask quantities purchased from mask manufacturers as a result of exposure to mask manufacturing (purple time series, Figure 3), these differences fade after Rwanda enforced national mask quality standards in June 2020. Recall that the mask mandate went into effect in April, just as the first wave of domestic mask manufacturing were rolled out. What this means is that, in districts with low exposure to formally-traded masks, people still had to wear masks; as in other countries, they bought lower-quality masks, produced informally in local markets or at home. As the licensing was expanded to all garment manufacturers in June, these districts with originally low exposure to mask manufacturing start buying more high-quality, formally-traded masks. But recall price differences persisted past the licensing expansion: the ban on the sale of low-quality masks combined with a well-enforced mask mandate lowered the elasticity of demand for domestically manufactured masks.
COVID-19 infections also went down!
We were then able to track the impact of exposure to mask manufacturing on COVID-19 infections, using paracetamol purchases from the receipt data as a proxy. This approach was our only hope to measure COVID-19 infections at small-scale geographies before testing became widespread. Scaling our reduced-form estimates by the average of exposure (shown on the map, Figure 2) we find that domestic mask manufacturing reduced COVID-19 infections in June 2020 nationally by 31% (orange time series, Figure 3), or a 12% decrease in monthly infection growth. This highlights the cost of trade frictions: despite the later expansion in licensing in those sub-Districts with lower exposure to mask manufacturing, reduced access to certified masks persistently increased the level of COVID-19 infections.
Mask quality matters
Our interpretation of these results in a context with a well-enforced mask mandate is that mask quality drove these persistent differences in the level of COVID-19 infections across sub-Districts with high and low exposure to mask manufacturing. The persistence of the price differences combined with the fact that the differences in both quantity of domestically manufactured masks and paracetamol purchases fade out shortly after national mask quality standards are applied lend support to a quality rather than a quantity channel. In other words, it’s a bit hard to reconcile a quantity channel leading to a fall in infections in low exposure sub-Districts as mask prices did not fall in those sub-Districts.
In summary, high-quality masks prevent COVID-19 infections, and promotion of domestic mask manufacturing effectively increased access to certifiably high-quality masks. Now, let’s interpret our magnitudes. Our policy impacts on COVID-19 infection growth and mask purchases suggest using a high-quality mask reduced monthly COVID-19 infection growth by 37% at early stages of the pandemic! This is similar to estimates from the United States and Bangladesh — high-quality masks are an important non-pharmaceutical intervention across a diverse range of contexts. We can then directly compare the costs of promoting domestic manufacturing of high-quality masks to the averted COVID-19 infections — the policy cost $10 per averted COVID-19 infection, an order of magnitude smaller than health costs.
Join the Conversation