I recently gave a talk at the American Association for the Advancement of Science about Big Data and Analytics and why it matters for development. Unlike other speakers who warned about risks associated with big data - when too much is known about too many people without their consent - I discussed the problem of data gaps and data poverty in the developing world. The challenge of measuring of poverty is different because if we don’t have the data, we can’t know whether we’re making progress in fighting this stain on our collective moral conscience.
The World Bank for example, monitors poverty in 155 countries, but we only have recent poverty estimates for half of them, and those estimates are already 5 years old. This is problematic for an institution whose stated goal is to eliminate extreme poverty over the next 15 years. How will we know when we get there?
As we’re learning from our staff and others working in data-starved environments - high frequency and real-time data from a variety of new sources can be really useful. We can use digital signals to see what's happening in a country at a micro-level to target interventions like the distribution of food, medicine, and other essential services. For example, in areas where mobile penetration is high, mobile airtime and the geographic distribution of pre-paid credits can signal in real-time that incomes are fluctuating and where it’s happening. Similarly, the movement of mobile phones across geographies reveals migration patterns that are useful in tracking the spread of diseases like Ebola. While this data is not perfect, it's often the best we have and an entirely new source of intelligence.
But beyond the data buzz, its important that we subject data to hypotheses to ensure our theories are sound and ‘data proxies’ are reliable. We need to test theories before acting on them. One challenge is that N is not equal all. Tim Harford reminds us that correlations can be misleading and are often just wrong. In several examples above, the patterns we observe in call detail records are not related to all people who live in a specific geography but people with phones. So we need to recognize these limitations and acknowledge what data does and doesn’t t tell us.
Then there is the challenge of data noise. As Nate Silver reminds us most big data is just noise and that noise is increasing must faster than the signal. He writes, “there are so many hypotheses to test, so many data sets to mine--but a relatively constant amount of objective truth.” Formulating good questions is always difficult but even more critical when parsing through very large data sets and looking for meaningful correlations. In a recent publication, " Big Data in Action for Development," the World Bank Group collaborated with Second Muse, a global innovation agency, to explore big data's transformative potential for socioeconomic development. The report develops a conceptual framework to work with big data in the development sector.
The emergent field of big data analytics and data science is a combination of statistics, computer science and social science. The first two without the third can lead to spurious and often dangerous conclusions. Imagine a health intervention that completely misses vulnerable populations because they don't own GPS-enabled smart phones. We have learned from Google’s flu trends that big data sets need to be regularly ground-truthed and subjected to scrutiny.
As someone who works with large data sets within a global development institution, I'm a firm believer in the power of real-time data. But our analytics must be backed by credible hypotheses and sound social science. It’s important to be clear about what data does and doesn't tell us. Only then can we move from data to knowledge and from intelligence to actionable insight.
The World Bank for example, monitors poverty in 155 countries, but we only have recent poverty estimates for half of them, and those estimates are already 5 years old. This is problematic for an institution whose stated goal is to eliminate extreme poverty over the next 15 years. How will we know when we get there?
As we’re learning from our staff and others working in data-starved environments - high frequency and real-time data from a variety of new sources can be really useful. We can use digital signals to see what's happening in a country at a micro-level to target interventions like the distribution of food, medicine, and other essential services. For example, in areas where mobile penetration is high, mobile airtime and the geographic distribution of pre-paid credits can signal in real-time that incomes are fluctuating and where it’s happening. Similarly, the movement of mobile phones across geographies reveals migration patterns that are useful in tracking the spread of diseases like Ebola. While this data is not perfect, it's often the best we have and an entirely new source of intelligence.
But beyond the data buzz, its important that we subject data to hypotheses to ensure our theories are sound and ‘data proxies’ are reliable. We need to test theories before acting on them. One challenge is that N is not equal all. Tim Harford reminds us that correlations can be misleading and are often just wrong. In several examples above, the patterns we observe in call detail records are not related to all people who live in a specific geography but people with phones. So we need to recognize these limitations and acknowledge what data does and doesn’t t tell us.
Then there is the challenge of data noise. As Nate Silver reminds us most big data is just noise and that noise is increasing must faster than the signal. He writes, “there are so many hypotheses to test, so many data sets to mine--but a relatively constant amount of objective truth.” Formulating good questions is always difficult but even more critical when parsing through very large data sets and looking for meaningful correlations. In a recent publication, " Big Data in Action for Development," the World Bank Group collaborated with Second Muse, a global innovation agency, to explore big data's transformative potential for socioeconomic development. The report develops a conceptual framework to work with big data in the development sector.
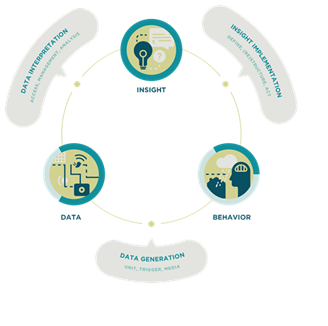
As someone who works with large data sets within a global development institution, I'm a firm believer in the power of real-time data. But our analytics must be backed by credible hypotheses and sound social science. It’s important to be clear about what data does and doesn't tell us. Only then can we move from data to knowledge and from intelligence to actionable insight.
Join the Conversation