Editor’s Note: This blog is part 2 of a blog series (read part 1 here). This blog aims to contribute to an on-going conversation as to how a set of converging cloud, AI, and satellite and aerial imaging technologies can be harnessed in an end user-centric fashion to strengthen the local governance of public interest infrastructure, land, and natural resources. These types of GovTech decision-support platforms increasingly deliver fit for purpose capability, and significantly reduce user complexity and cost.
Satellite Imagery Analysis for the People
Until now, AI classifications have been out of reach for most local government stakeholders across both the developed and developing world. Leveraging AI techniques has meant bringing in specialized cooks and hardware, and these barriers mean that the exposure and adoption of this technology is significantly reduced, even when free imagery is available.
To lower this barrier, Microsoft, through its AI for Earth program — a USD $50 million, five-year commitment to put AI technology in the hands of those committed to protecting the planet — teamed up with the World Bank to develop a user-centric platform focused on giving local users access to AI algorithms. The platform enables users to train the deep learning algorithms together with freely available imagery, and/or imagery with a fee, on land cover mapping tasks. Beyond standard built up surfaces, green space, and water classifications, users can implement their own classification schemes (e.g., recently cleared land versus parking spaces). After a user provides a few “ground truth” labels in a local area, the deep learning model can then be trained to classify the whole area.
Users can interactively correct mistakes in the AI platform until they are satisfied with the performance, a human-in-the-loop AI method recently described in a AAAI 2020 paper. Once the land cover data is ready, it can be delivered in many formats: from Powerpoint presentations, geospatial data portals, or analytics in open source geographic information system (GIS) software (such as QGIS). By classifying land coverage across imagery from different time periods, we can also provide a quantitative view of places that have changed in classification.
The screenshots below show the Microsoft AI for Earth Land Cover Mapping platform applied to the Nhà Bè District in Ho Chi Minh City. With an area of just over 100 square kilometers, it is one of the city’s most rapidly developing districts. Its low-lying topography, with exposure to rains and tidal exposure also make it vulnerable to flood. The platform was applied using 2017 and 2019 for-free and for-fee imagery. Local stakeholders were able to determine across both time periods the land use classification data.
While the AI PhDs may argue about ways to improve the estimates at the margin, the platform for the first time provided local stakeholders with a tangible sense of what AI as applied to different types of satellite imagery actually means in practice.
Landing page for the AI for Earth Land cover mapping applications
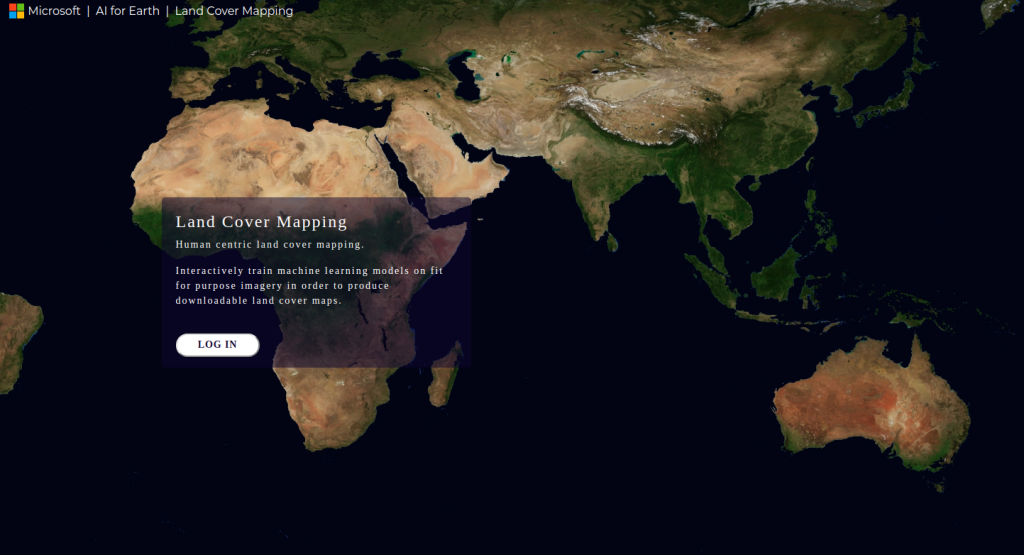
Application interface showing Pleadies 0.5m resolution satellite imagery from 2019 over the Nha Be District of Ho Chi Minh City. Users can run an AI model, provide labeled data by clicking on the map, and download results and summary statistics over broader areas.
Real World Implementation
Amid the hype about disruptive technologies, the challenge of better connecting local users with real world use cases across the world remains. This challenge is best solved by achieving bottom-up engagement with the new disruptive technologies. While experts and consultants can advise local governments with the help of new technologies, true change comes when a local user is actually engaged and empowered with the technology.
The platform was field tested by users in Vietnam and Myanmar. As part of a recent AI conference in Ho Chi Minh City and local stakeholder engagement, we took stock of where the initiative has come and where it expects to go:
Radical Workflow Simplification: Existing AI + GIS initiatives are complex. For example, tools such as ArcGIS and Google Earth Engine expose extremely powerful abstractions for working with geospatial data and, as such, require a correspondingly large amount of training to use effectively. This has limited value when connecting with people and processes “on the ground”. Once local users are engaged, complexity can be added. However, the first step is to convince local users that they will ultimately be the cooks that bring together the imagery, labels, and algorithms.
Value is Revealed in Local Use-Cases: Basic land use classifications are helpful in most any context. But true engagement comes from linking to a use case that resonates locally, such as identifying recently cleared lands in protected or zoned areas. Part of this requires a process of local discovery and engagement, coming from progressively empowering local users with tools they can take ownership of. This is a starting point to assess if imagery or algorithms are fit for purpose, and a critical stepping-stone for any further initiatives.
From Free to Fee Imagery: Beyond NASA’s Landsat, the free Sentinel imagery series at 10 meters from the EU’s Copernicus program provides a useful base layer for land classification using AI for Earth. But a growing menu of restricted access and commercial imagery may be needed for more refined applications. The most constructive role the World Bank can play is to help inform local users to what is on the ground, and how to facilitate access as part of their interactive front-end platforms, such as through repositories and APIs.
Algorithmic Improvements: The platform can seamlessly integrate improvements in land cover mapping from an algorithmic standpoint. However, more work is needed to facilitate corrections submitted by large numbers of independent users. The two desirable properties of the platform are that 1.) contributions made by individual users should always improve performance of end product (land cover data) and 2.) contributions made by individual users should improve the performance of the end product at a faster rate than individually labeling each pixel.
The use of machine learning for land cover mapping now offers sub-national clients the opportunity to interactively engage and benefit from a new frontier set of technologies. It puts local stakeholders firmly in the driver’s seat in terms of offering ground truth data but empowers them by connecting them with a world of new satellite imagery and cloud-based algorithms.
Join the Conversation