Is the funk of the Job Market seminar season getting to you? Apparently you are not alone, as recent tweets from prominent development economists suggest :)
While we may not be able to help with some lose-lose scenarios, we pulled together a few off-the-shelf solutions to the most Gotcha!? questions we heard during this last JM season at World Bank DIME / Research Group.
What do we mean by "Gotcha!?”
Lots of general, difficult to answer questions get thrown out at seemingly every paper in every seminar. It’s often hard to interact with these questions without serious preparation — they’re very general, so how should one target the response? Good news is, preparation helps a lot! Other good news is, these are questions that actually are important to answer in a paper to improve the quality and interpretability of the research findings and will definitely crop up in the peer review process. So here's a tiny crash course in Gotcha!?
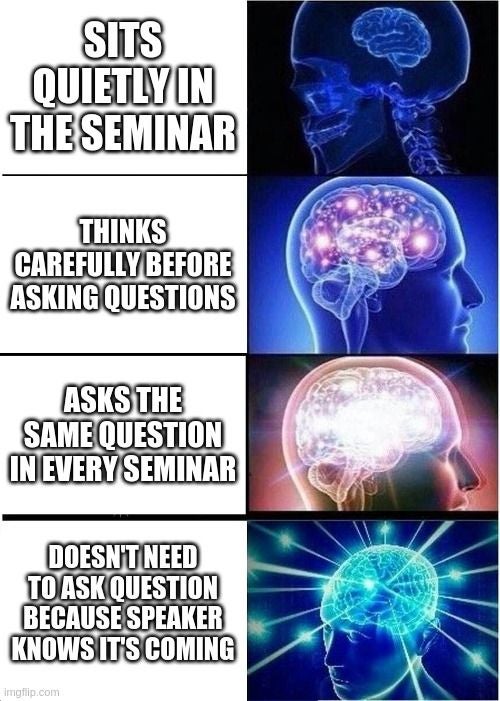
Why should I believe your treatment is exogenous?
Most empirical papers today are estimating the impact of some “treatment” assumed to be as-good-as-randomly assigned on some outcome, using a mix of research designs (event study, regression discontinuity, instrumental variables, …). The difficulty here is that the identifying assumption (exogeneity of the treatment assignment) cannot be tested directly. What to do?!
The most common approach taken to test the exogeneity assumption is placebo checks: testing whether variables that should not have been affected by treatment appear to have been affected. Finding meaningful placebo effects provides evidence that our “as-good-as-random” assignment may not be a viable assumption. These placebo checks have different common names for different research designs: “balance tables” for experiments, “parallel trends” for difference-in-differences/event studies, or even just “placebo checks” for regression discontinuity. Even tests of differential attrition follow this approach (i.e., does treatment predict attrition?)! More generally, this humorously titled paper points out that such an approach is useful for any research design, and much more robust than alternative “control for everything and see how the estimate changes” approaches. Having performed a broad range of these placebo checks is good preparation for a range of questions about specific violations of exogeneity: just put the confounder (or a noisy proxy for it) of concern on the left hand side!
Isn’t this just estimating a LATE? What does this tell us about ATE?
It’s rarely the case that the effect of the “treatment” of interest is estimated for a representative sample. When we use our estimate to ask what the impacts of a scaled up version of the program would be, we implicitly assume that the individuals impacted in our estimation sample (often called “compliers”) have the same “treatment effects” as the true subpopulation of interest: those impacted by the scaled up version of the program. These two groups of individuals may differ from the population at large on both characteristics that we can observe (e.g., gender) and some we can’t (e.g., latent ability).
More good news! Characteristics of the individuals who contribute to our estimated treatment effect can also be estimated (see this approach, for example, for instrumental variable models), and compared to characteristics of any subpopulation. Going one step further, this paper (among others recently) proposes a flexible machine learning approach to estimating heterogeneity in treatment effects. Under the assumption that variation in treatment effects is fully explained by observable characteristics (which may be reasonable in some contexts), this approach can be used to estimate average treatment effects for any subpopulation! In other cases, unobservables may drive important heterogeneity in treatment effects; in these cases, outcomes of non-complier individuals can be informative about how selection affects our estimates.
SUTVA
Often times, programs impact not only beneficiaries, but also “spillover” onto non-beneficiaries. When someone says “SUTVA”, they’re almost certainly talking about these spillovers: if they exist, then estimates of program impacts now combine not only the direct impacts of the program, but also the difference in spillovers onto beneficiaries and non-beneficiaries.
Typically, disciplined speculation (potentially with help from a model) can isolate the types of spillovers we should worry about, and suggest approaches to estimate each spillover. In a recent paper of ours, we compiled a list of spillover questions we’d received and addressed them one-by-one. First, concern about general equilibrium effects arose, where prices would be impacts by the program we studied. We used both data on price trends (to look at magnitudes of impacts) along with some economics to interpret how these effects would impact our estimates. Alternatively, recent experimental work has randomized treatment assignment at community level or higher (remember worm wars?) to estimate within community spillovers. Second, as our paper was about agriculture, concern about within household spillovers arose; we therefore directly estimated impacts of one plot being treated on the household’s other plots. In both cases, restrictions (on the channel, prices, or the scope, within household) make it possible to estimate spillovers.
Clustering
Many times, program assignment is determined at a unit above individuals — targeted communities or districts may be selected to receive the program we’re evaluating. In these cases, traditional econometric tools for inference become complicated, as both program assignment and counterfactual outcomes may be correlated within communities. Inevitably, someone will pop the question: did you cluster your standard errors? Oh no?!
Fortunately, there’s been recent developments on the theory behind how to appropriately cluster your standard errors. In particular, this paper (discussed previously on this blog) formalizes how your experimental design, your sampling design, and what exactly you’re estimating (e.g., ATE for full population or just the sample you happened to select for your experiment?) all determine the correct choice of how to cluster. Further, while traditional cluster robust inference required large samples, new corrections make it feasible with as few as 10 clusters, while randomization inference and bootstrap methods remain viable options. However, complications remain with secondary data without random assignment (e.g., using event study or conditional on observables designs), with best practices evolving. Conley errors (we mentioned these very briefly on a previous blog, and are committing our future selves to blog more on these soon…) are one approach to robust inference with spatial data.
Don't worry, we gotcha !!!
Lastly, remember: playing nice is required for better, more constructive seminars
Also, please send us your comments with your favorite gotcha questions and we will try to build this up (Gotcha!? 2.0!) and populate the technical topics of this blog accordingly.
Join the Conversation