To some, artificial intelligence is a mysterious term that sparks thoughts of robots and supercomputers. But the truth is machine learning algorithms and their applications, while potentially mathematically complex, are relatively simple to understand. Disaster risk management (DRM) and resilience professionals are, in fact, increasingly using machine learning algorithms to collect better data about risk and vulnerability, make more informed decisions, and, ultimately, save lives.
Artificial intelligence (AI) and machine learning (ML) are used synonymously, but there are broader implications to artificial intelligence than to machine learning. Artificial (General) Intelligence evokes images of Terminator-like dystopian futures, but in reality, what we have now and will have for a long time is simply computers learning from data in autonomous or semi-autonomous ways, in a process known as machine learning.
The Global Facility for Disaster Reduction and Recovery (GFDRR)’s Machine Learning for Disaster Risk Management Guidance Note clarifies and demystifies the confusion around concepts of machine learning and artificial intelligence. Some specific case-studies showing the applications of ML for DRM are illustrated and emphasized. The Guidance Note is useful across the board to a variety of stakeholders, ranging from disaster risk management practitioners in the field to risk data specialists to anyone else curious about this field of computer science.
Machine learning in the field
In one case study, drone and street-level imagery were fed to machine learning algorithms to automatically detect “soft-story” buildings or those most likely to collapse in an earthquake. The project was developed by the World Bank’s Geospatial Operations Support Team (GOST) in Guatemala City, and is just one of many applications where large amounts of data, processed with machine learning, can have very tangible and consequential impacts on saving lives and property in disasters.
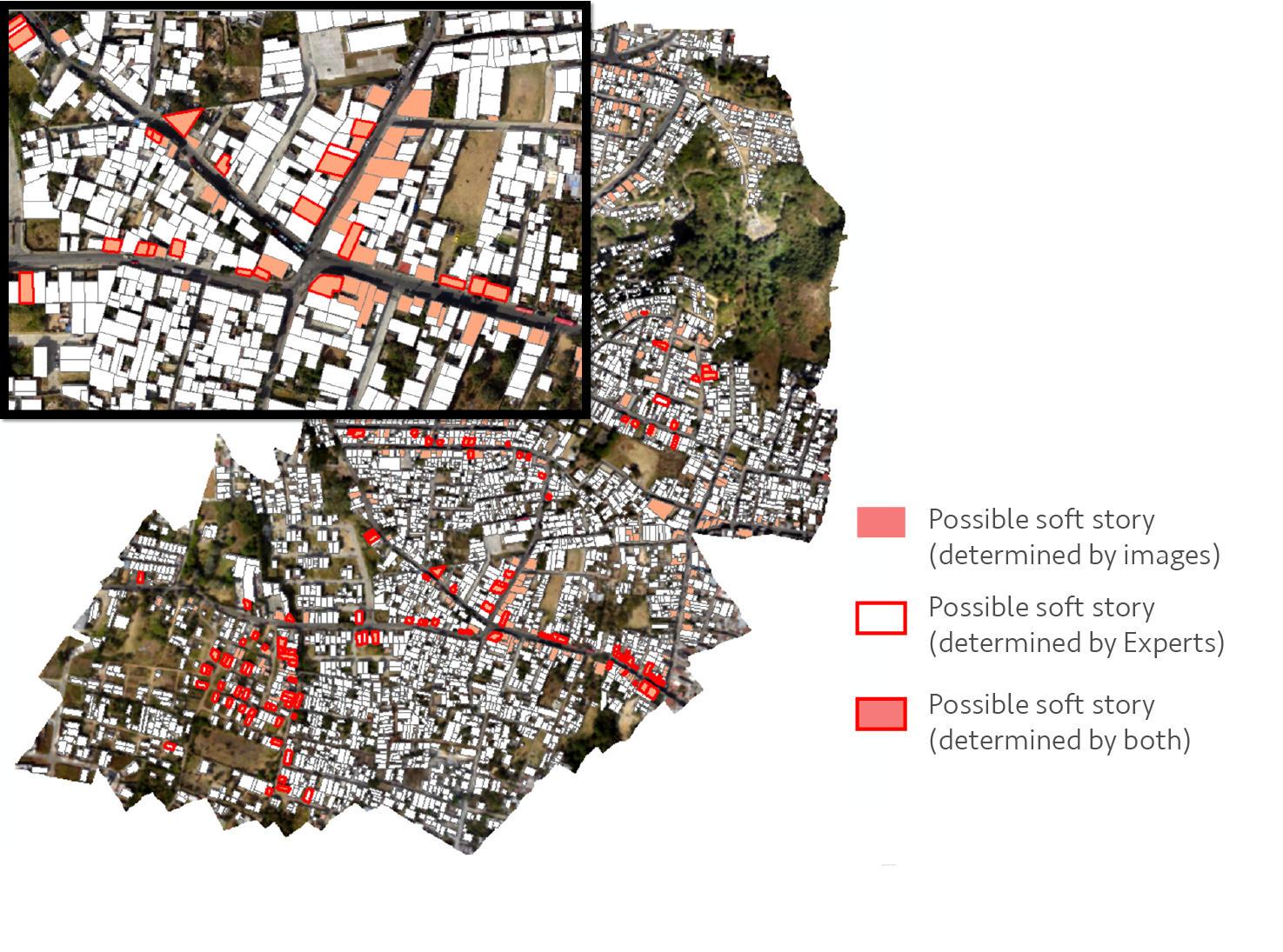
In another case, machine learning was used to process input satellite imagery in order to separate informal settlements, sometimes referred to as “slums,” from formal, planned ones. The project led by Jordan Graesser and the Oak Ridge National Laboratory was done in cooperation with the World Bank, and the methods are now being implemented by GOST to apply to other user cases.
The impact of this type of work cannot be understated, as putting the most vulnerable neighborhoods, and people, on the map, allows us to more accurately define the vulnerability of populations to hazards. More accurate vulnerability mapping is one of the foundations of more effective disaster risk management. Other methodologies have seen their share of success in collecting accurate vulnerability information, for example, by adding more boots on the ground for surveying techniques or doubling down on crowdsourcing campaigns in efforts to map communities. Using machine learning algorithms can vastly reduce project costs, whether it is applied to satellite, drone or street-level imagery, or data from entirely different sensors and sources, such as seismic sensors or social media posts.
Democratizing data, minimizing bias
GFDRR’s Open Data for Resilience Initiative (OpenDRI) advocates the integrated use of these technologies to maximize the impact of projects and minimize costs. Machine learning-based automated mapping, crowdsourcing campaigns to collect “training” and “validation” data, and the analysis of this data by experts, can and should all be tied together seamlessly. Training and testing data are used to ‘teach” (train) the machine learning algorithms and then assess (test) how well they work. Expert knowledge is necessary for collecting “ground truth” data, for implementing machine learning software and for analyzing results in a meaningful way.
Open data means that data inputs and outputs of projects should be freely available and easy to use. By being open to all stakeholders, the disaster risk management process is democratized. Data ownership then rests with the communities it refers to, leading communities to own their DRM projects and hence the resulting DRM recommendations. Capacity building and crowdsourcing are a vital component of projects that focus on integrating open data, as by definition these activities involve communities in the creation and sharing of data.
Minimizing bias in all projects is of paramount importance. Just because computers are analyzing and processing data using machine learning, it does not mean that the entire process is aseptic and free of bias. Notoriously, computer models are only as good as their input data. Therefore, biases in the input data can persist through to the results. Biases can affect the mitigation strategies that protect people and communities and therefore have a big downstream impact on the loss of lives and property.
More than a buzzword
Artificial Intelligence and machine learning have become buzzwords in the last few years, somewhat replacing terms like “big data” and “data science”. In reality, these terms all refer to the same set of statistically-based computer science methods that are used to sift through, separate and act upon data. Machine learning methods simply do so in recursive programs, or algorithms that learn from the input data to improve subsequent results.
Machine learning algorithms already power the internet: in online shops, search engines, targeted ads and social media feeds. They power our homes with the burgeoning appearance of smart-home and voice assistant devices and they also power our mobile world from personal assistants to voice commands to instant translation, just to name a few applications. What would the world look like if we harnessed all of that automated computational power to protect people?
READ MORE
Join the Conversation